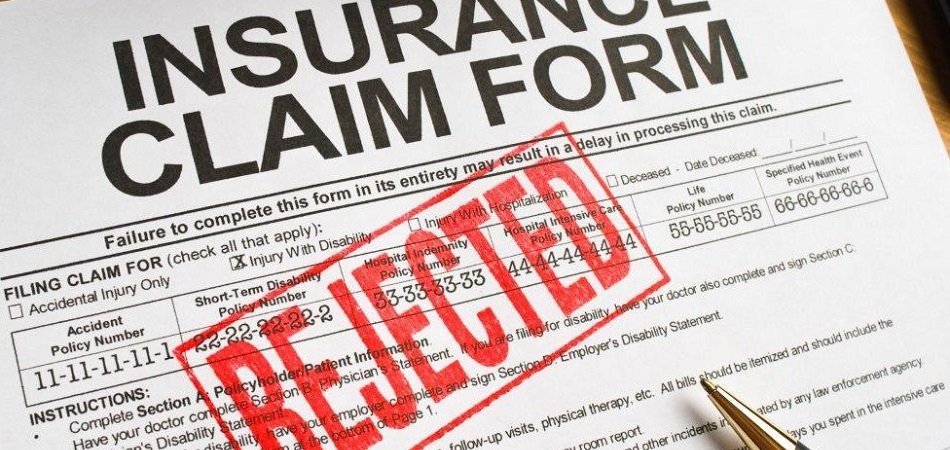
In recent years, the demand for greater cybersecurity has risen even among the everyday citizen. This is especially true for one’s personal and financial information, which fraudsters are constantly finding new methods of breaching accounts to find. This need for security has also risen in insurance, and numerous AI firms are selling claims fraud detection solutions to the insurance sector.
In this article, we outline the current possibilities of AI for fraud detection in insurance. We discuss which software some of the top global insurers are using and why they claim it worked for them. Then we outline the types of fraud detection software available in the AI space and how each of them could offer their clients ROI and flag less claims as false positives.
Our approach to this overview of AI-based fraud detection for insurance companies is listed as follows:
- How top insurers are using AI-based fraud detection, their choices of vendors, solutions, and information regarding its implementation
- How anomaly detection makes fraud detection possible in insurance
- How predictive analytics makes fraud detection possible in insurance
We begin our overview of the possible AI applications for insurance fraud detection with the methods used by some of the top insurers in the world including Anadolu Sigorta, AXA, and CNA.
How Top Insurers are Using AI-Based Fraud Detection Software
Anadolu Sigorta
Anadolu Sigorta is the first and one of the largest insurers in Turkey. The company employed a team of 50 people to manually check each claim for fraud based on loose rules and the team’s personal experience before switching to an AI software solution. They purchased a predictive analytics software from Friss, and according to the customer success story, they saw 210% ROI within one year of using the software.
With 25,000 to 30,000 claims to scrutinize for fraud indicators each month and a nearly two week manual process for checking each claim, Anadolu Sigorta wanted to speed up this process and allow for more claims to be processed. After implementing Friss’ software, the company was able to gauge the fraud risk of a claim in real time. Friss claims in their customer success story that Anadolu Sigorta saved $5.7 million in fraud detection and prevention costs.
The video below shows Faith Gören, Deputy Chief Officer of Anadolu Sigorta, talking about their decision to work with Friss and how they benefited from automating the claims fraud detection process:
AXA
AXA, one of France’s top life insurance companies, also adopted an AI fraud detection software. They worked with UK-based startup Darktrace to employ a solution that could help them detect and handle threats from advanced cyber-criminals.
Darktrace claims that AXA was primarily concerned with monitoring their entire network and the ability to contain emerging threats before they become a larger problem.
With a complex infrastructure of acquired companies of different levels of integration with AXA proper, the insurer was looking for a solution that could handle very different circumstances depending on the branch of the company it is analyzing.
According to a case study published by Darktrace, their Enterprise Immune System software is trained on the behavior of computer- and network-using employees and detects their patterns across the workday.
Once it has discerned each user’s patterns, it purportedly begins to correlate the data to discern the relationships between the duties of separate users. The Darktrace Enterprise Immune System is packaged with Antigena, the company’s autonomous response software that takes action against detected threats.
Antigena is advertised as able to shut down users within a client company’s network whose behavior is consistent with what it has been trained to detect as a data security thread, claims fraud, or preparation for fraud.
CNA Financial
Another large insurer that has adopted AI software for fraud detection is CNA Financial. The company wanted to automate their claims fraud detection process but also understand the context surrounding the discovered fraud. They chose Shift Technology as their preferred vendor for automating this process and became the first company to use Shift Technology’s FORCE software solution.
CNA Financial stated in a press release that they would be able to optimize their special investigations through a focus on the most suspicious claims with predetermined analytical paths.
Regarding the company’s deal with Shift Technology, Rob Thomas, Senior Vice President of Claim Analytics, Finance and Operations for CNA’s P&C claims unit said, “By partnering with Shift Technology, CNA will optimize its special investigations efforts by focusing on the most suspicious cases with pre-identified paths for investigation.”
This means the insurer wanted to ensure all known fraud methods are investigated within their claims. It follows that they sought out the FORCE software for its ability to offer context into the observed fraud situations and guidance towards the proper resolution.
Because Shift Technology claims their FORCE software includes predetermined paths for fraud analysis and provides contextual information regarding the best next step for the client, we can infer that the software is a predictive analytics application.
How Anomaly Detection Makes Fraud Detection Possible in Insurance
Anomaly detection-based fraud detection differs from the less common predictive analytics approach to AI fraud solutions. The anomaly detection approach is similar to other AI applications in that their machine learning models are all trained on a stream of labeled data.
This allows machine learning models to establish a baseline of what a normal claim might look like, and thus a general sense of how to recognize any anomalies.
When a given event or claim deviates from the now established normal pattern, the software will notify a human monitor. That monitor, usually a claims expert or data scientist, may either accept or reject this notification. This indicates to the machine learning model that its conclusion on whether a given claim is fraudulent is right or wrong.
This further trains the machine learning model to understand that its conclusion was correct and to allow for similar deviation in the future if it was incorrect. At the same time, the claims expert or data scientist will also be able to use the machine learning model’s conclusions on what is fraud or not to further improve it.
Anomaly detection could also be used for insurance fraud detection outside the claims process. Like CNA, an insurer may use anomaly detection to identify the suspicious behavior of users on an insurer’s network. Additionally, this type of software could be combined with other AI applications under one solution.
For companies that want to be able to search for relevant information regarding the problem the machine learning model would solve, a solution combined with NLP document search software could help. This would be for business leaders to review data from the same database the machine learning model pulls from in order to better understand something the machine learning model revealed such as a new fraud method.
We spoke with Kevin Lee, resident Trust and Safety Architect at Sift Science, a global AI fraud detection software vendor. On our podcast, AI in industry, we asked Lee about the current possibilities and applications for AI fraud detection.
With regards to the benefits machine learning could offer insurers looking to automate the claims fraud detection process, Lee said:
A human analyst or a human reviewer can only look at a handful of signals at a time and make a determination. But there is enough data out there and that’s really when machine learning comes into play. Because it’s literally able to crunch thousands of signals and look at probabilities of abuse or probabilities or fraud. That’s really where the industry is going from a machine learning viewpoint.
How Predictive Analytics Makes Fraud Detection Possible in Insurance
In addition to anomaly detection software, machine learning models for insurance fraud detection can be used as the basis for predictive analytics and prescriptive analytics software. Prescriptive analytics software takes the correlative predictions of predictive analytics one step further to provide the user with recommendations for the best next step to take when fraud is detected. That said, each of these requires the same data and training requirements to properly implement and develop.
Predictive analytics-based fraud detection software operates differently from software based on anomaly detection. Claims experts from the client insurer will need to label a large amount of claims as fraudulent and legitimate respectively.
This way, the machine learning model will discern fraud methods according to the fraud methods present in the labeled fraudulent claims.
For example, fraudulent claims used for training the machine learning model could employ a fraud method relating to the typed dollar amount in the claim.
Once the software has learned to detect this fraud method, it will be more sensitive to the dollar amount of each claim and flag claims with suspicious claims amounts
Many companies will find they already have this data labeled because of their storage of fraudulent claims records from years past. The claims experts working on the machine learning model for the software dictate which claims are fraudulent or not during training. As they expose the software to these labeled claims, it gradually gets better at discerning the difference on its own.
The software would then discern data points that correlate to past fraud attempts within new claims as they enter the system.
SAS is one such vendor that offers predictive analytics software to insurers to help them automate the fraud detection process. Their predictive analytics solution, SAS Enterprise Miner, can purportedly help health insurance companies detect fraudulent claims and locate important information for determining the fraud risk of new claims.
The vendor also states the software can use client enterprise data to create models, or mock claims showing possible fraud cases based on the variables between data points of past claims. For example, a claims expert training the machine learning model might want to test how often fraudulent claims are for amounts above $100 and below $200.
In this case, they could test the “fraud” variable” against the “price” variable, and view the results that show claims valued within that range.
Below is a short demonstrational video on how Enterprise Miner works. The video is a walkthrough of the process of creating and using predictive models. The demonstrator uses an example topic, but the same principals can be applied to insurance claims fraud. The steps covered within the video are as follows:
- 0:00 – Selecting data points and variables and reviewing data
- 3:00 – Checking target variables against others to find correlations, and model creation
- 4:05 – Results and how to read predictive models
- 5:19 – Using multiple models concurrently
According to a case study published on SAS’ website, the company helped insurer DentaQuest reduce fraud and see raise ROI from their marketing campaigns using Enterprise Miner. DentaQuest was purportedly able to identify 50 customer behaviors that correlated with their claims data labeled as fraudulent.
“SAS enables us to not only reduce costs but also improve quality of care. Now, instead of using high-cost restorative services, we can shift patients to providers with more beneficial preventive, diagnostic-type services,” said Dean Webb, Senior Manager of Analytics at DentaQuest.
We can infer from this quote that the insurer was able to extract predictive insights about their clients that helped them recommend preventative and diagnostic dental services.
This means DentaQuest spent less money on claims for insured dental operations and their customer base had more access to preventative dental care.
Header Image Credit: DG Law