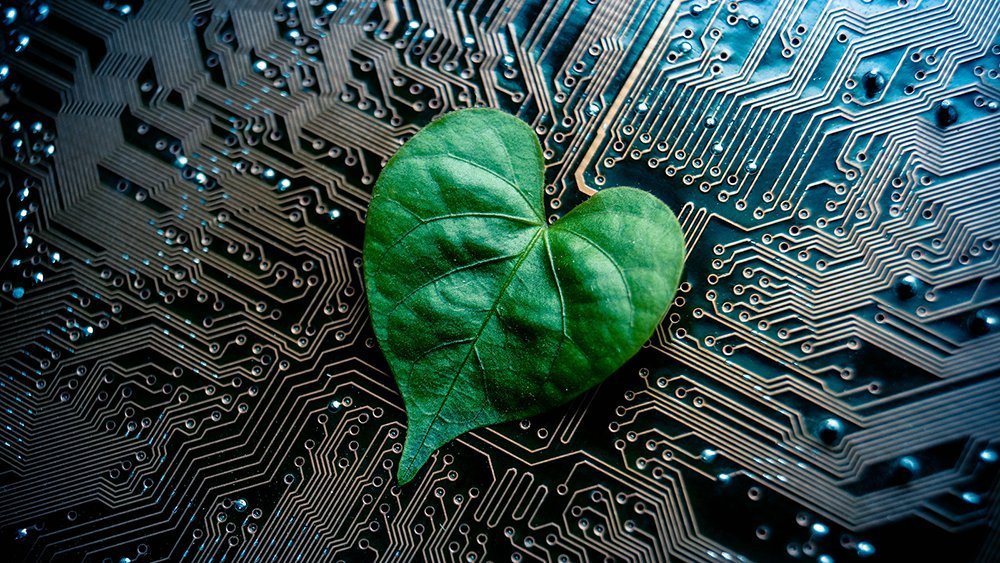
The U.S. Energy Information Agency (EIA) defines renewable energy as an energy source that naturally regenerates, such as solar or wind. In contrast, fossil fuels are considered finite. The EIA reports that in 2016, 10 percent of all energy consumed in the U.S. was derived from renewable energy sources. This is equivalent to roughly “10.2 quadrillion British thermal units (Btu) —1 quadrillion is the number 1 followed by 15 zeros”.
Despite the increasing use of renewables which notably became the leading global source of electricity in 2015, there are still persistent barriers to wider implementation related to policy and technology. Researchers and companies are exploring how artificial intelligence could assist in improving accessibility and efficiency of renewable energy technology.
In this article we present examples of renewable energy technologies which incorporate AI. We cover three major categories of renewable technologies that should be of interest to business leaders in the green energy space:
- Energy Forecasting – Industry data is used to train AI algorithms to make accurate forecasts, helping to inform power supply and demand
- Energy Efficiency – AI is used to track and optimize how energy efficiency
- Energy Accessibility – AI is used to model utility cost savings and provide recommendations for smart home investments
For each application we provide a company overview, explanation of how the platform functions and outcome data and/or results where available. Each example is organized under a sub-heading which serves as a quick reference when navigating through the article.
AI for Energy Forecasting
Xcel
A consistent challenge with renewable energy sources such as wind and solar power is their unreliability. Weather-dependent power sources will often fluctuate in their strength.
In Colorado, energy provider Xcel is implementing AI in an attempt to address these challenges. Through the National Center for Atmospheric Research’s new AI-based data mining method, Xcel was able to reportedly access weather reports with a higher level of accuracy and detail.
This meant that greater precautions could be taken to harness and preserve the energy that was generated. In order to provide these detailed weather reports, the AI system mines a combination of data from local satellite reports, weather stations as well as wind farms in the surrounding area. The algorithms driving the system are trained to identify patterns within these data sets and make predictions based on those data points.
Xcel reports that wind power has doubled in Colorado since 2009. Earlier this year, Xcel reported plans to expand its wind farms by 50 percent by the year 2021.
Nnergix
Weather can often be unpredictable, destabilizing the power supply generated from weather-dependent energy sources such as solar and wind. This puts pressure on the renewable energy sector to efficiently balance supply and demand.
Historically, weather forecasts have helped energy suppliers make predictions regarding their power supply. Today, companies such as Nnergix are incorporating artificial intelligence to improve the accuracy of renewable energy forecasting.
Nnergix is a data mining and web-based energy forecasting platform which pools data from the energy industry. The company reportedly combines satellite data from weather forecasts and machine learning algorithms trained on industry data to make more accurate forecasts.
High resolution weather forecasts appear to be generated from satellite images. Large scale and smaller scale weather models are reportedly generated based on these images.The machine learning algorithms analyze these data and can then predict the state of the atmosphere for a particular area.
For example, the company offers three main services including a solar energy solution and claims that forecasts can range from 6 hours to ten days in advance with data updates occurring eight times a day. Reports can be delivered in multiple formats in as depicted in the graph below:
To delve deeper into AI applications of weather forecasting, readers may find our article title AI for Weather Forecasting – In Retail, Agriculture, Disaster Prediction, and More to be a useful resource.
AI for Energy Efficiency
Verdigris Technologies
Founded in 2011, California-based Verdigris Technologies offers a cloud-based software platform that claims to leverage artificial intelligence to help clients optimize energy consumption. Designed for large commercial buildings and managers of enterprise facilities, the process begins with installation of IoT hardware.
Smart sensors are directly attached to the client’s electrical circuits to track energy consumption. The data captured by the sensors is sent to the cloud “securely over Wi-Fi or Verizon 4G/LTE.” and is presented to the client on a dashboard which is accessible online 24/7.
The involvement of Verizon runs a bit deeper than a wireless connection. In October 2016, corporate Venture Capitalist firm Verizon Ventures made an undisclosed investment in a Series A funding round amounting to $6.7 million. To date, Verdigris has raised over $16.5 million in total funding.
Every appliance tends to have a unique electrical footprint, as a result the algorithms have been designed to be able to identify each unique energy source while still providing a comprehensive analysis of the captured by the smart sensor hardware. The data analysis is communicated to Verdigris’ cloud-based servers.
The 57 second video below provides a visual overview of how the company’s platform works:
In one case study, Verdigris reports that it worked with W Hotel San Francisco to identify energy inefficiencies in the hotel’s commercial kitchen. WIthin a three month period, the company reportedly identified inefficiencies that were costing the hotel more than $13,000 in preventable annual losses.
Google DeepMind
Founded in London in 2010 and acquired by Google in 2014, AI company DeepMind Technologies Ltd. reportedly reduced the amount of energy required to cool Google’s data centers by 40 percent.
DeepMind reported these results in July 2016, however, the company claims that it first began applying machine learning two years prior to improve energy usage. Specifically, a set of data center operating scenarios and parameters were used to train a system of neural networks. The neural network “learned” how the data center functioned and began identifying opportunities for optimization.
Google claims that data was pulled from thousands of sensors located in the data centers. Information collected included temperature and power consumption. Power Usage Effectiveness is defined as the ratio of “total building energy usage to IT usage” and was used to train the neural networks. The PUE model helps ensure efficiency so when the neural network system provides recommendations they do not exceed operating constraints.
The graph below depicts an average day where the model was tested using live data and indicates when machine learning recommendations were switched on and off.
Image credit: DeepMind
The Google data centers house the servers which power Google’s top applications including Gmail and Youtube which estimates over a billion users, representing roughly one-third of all internet users. Google’s capital expenditures have been reported to mainly support data center operations and improvements. In 2016, total expenditures reached an estimated $10.9 billion, up from $9.9 billion in 2015.
(DeepMind’s own Dr. Nando de Freitas joined us on our AI in industry podcast in 2016 to explain “deep learning” in simple terms – and the episode is one of our most popular of all time. Listen to the episode on Soundcloud or iTunes.)
AI for Energy Accessibility
PowerScout
In an effort to improve consumer education and access to renewable energy technologies, PowerScout reportedly uses AI to model potential savings on utility costs using industry data.
The company reportedly leverages data analytics to identify “smart home improvement projects” based on the unique features and energy usage in a client’s home. PowerScout’s algorithm appears to match clients to potential hardware installation providers in an online marketplace format to ensure competitive rates.
Essentially, the AI acts as a marketplace advisor, providing recommendations to help clients make informed decisions regarding to make renewable energy technologies purchases for their homes. We imagine that this use of AI is similar to the recommendation capabilities seen in other marketplace businesses (which we’ve covered in greater depth in our recommendation engine use-case article). The development team claims the platform has collectively overseen the installation of solar capacity roughly equivalent to powering 250,000 homes as of March 2017.
PowerScout lists Google and the US Department of Energy among its partners. In fact, the company is the recipient of two grants from the US Department of Energy amounting to a total of $2.5 million.
Verv
Verv is an AI-powered home assistant created by London-based Green Running Ltd. The system reportedly uses its technology to assist clients with energy management in their homes.
Verv supplies energy data on home appliances and itemizes energy costs on a consistent basis. Users are reportedly able to see a record of how each appliance in their home uses energy and monitor and regulate their energy expenses before bills are due.
When a household appliance is turned on, the algorithms driving the the AI assistant recognize patterns and can automate a running tally of the energy costs that the item is generating. Verv also reportedly has several safety features that provide notifications when devices are left on for prolonged periods of time as well as tips to reduce a household’s carbon footprint. The app is available for tablets, laptops and Smartphones.
The 1:28 minute video below provides a visual of Verv’s technology and an equipement installation demonstration:
Concluding Thoughts and Future Outlook
The renewable energy sector is a growing economic force and an effective strategy towards improving environmental sustainability. Artificial intelligence is being integrated across major sectors of this industry, increasing the capacity of data analytics.
The variable nature of weather presents inherent challenges which may cause suppliers to rely on traditional energy sources to meet consumer demands. Therefore, AI-driven energy forecasting platforms may hold promise for providing energy suppliers with the data required to respond to fluctuations that may negatively affect operations and to plan accordingly.
2015 was a banner year for renewables evidenced by the commitments by the G7 and G20 to accelerate implementation and improve overall energy efficiency. However, overcoming the barriers to widespread and accelerated implementation will require ongoing evidence of benefits, particularly in the economic and political arenas.
Platforms which can accurately identify cost savings and energy efficiency for consumers and companies will prove valuable in the near term.
Readers interested in understanding how AI is being used in the traditional energy sector may find Artificial Intelligence in Oil and Gas – Comparing the Applications of 5 Oil Giants to be a useful read.
Header image credit: itpro.co.uk