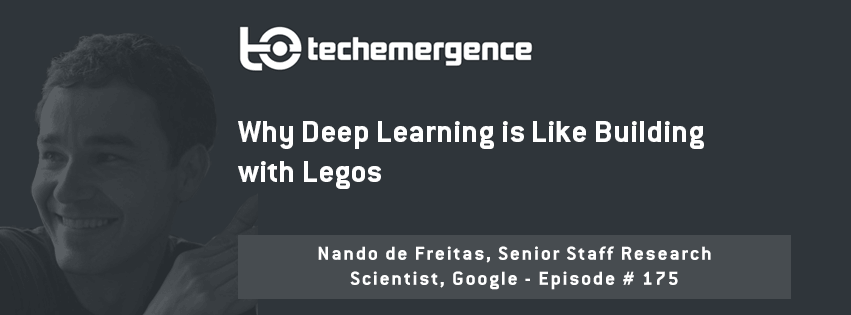
Episode Summary: One of the most memorable moments from this interview is when our guest mentioned that Larry Page hired him to solve intelligence; very few people can say this, and this says something about today’s guest, Dr. Nando de Freitas – a senior researcher at Google and professor at Oxford – as well as the gravity of his present work. Today, I speak with Nando about a topic well known through his research at Google, deep learning. de Freitas gives his perspective on the basics of deep learning, the applications in conversational interfaces and recognizing images and videos, and what the future of this technology might look like in the nearer future.
Expertise: Machine learning, prediction, statistical analysis, optimization, web-scale information extraction, and artificial intelligence
Recognition in Brief: Nando de Freitas, PhD, is a Professor of Computer Science at Oxford University as well as a senior staff research scientist at Google in London. Prior to Google, de Freitas was co-founder in 2014 of Dark Blue Labs. He has won numerous awards and fellowships over the course of his career, most recently the Charles A. McDowell Award for Excellence in Research at IJCAI 2013. From 1999 to 2001, de Freitas was a visiting post-doctoral scholar with Stuart Russell at UC Berkeley, where he worked on machine learning, computer vision, image retrieval, probabilistic models in artificial intelligence, variational inference algorithms, particle filtering and MCMC simulation. He earned a PhD (Bayesian methods for neural networks) from Trinity college, Cambridge University; a master’s of science degree in control systems at the University of the Witwatersrand, Johannesburg; and a bachelor’s of science degree in electrical engineering at the University of the Witwatersrand.
Current Affiliations: Professor at Oxford University; Senior Staff Research Scientist at Google; Fellow of the Canadian Institute for Advanced Research (CIFAR)
(Our interview with Nando took place in the media room of the KDD 2016 conference in San Fransisco)
Nando de Freitas Interview Questions:
(1:33) How do you articulate what deep learning is to people who didn’t academically study that domain, how do you explain why this matters?
Nando de Freitas: Deep learning is an approach that was influenced by neuroscience, and it start of started taking off from some results that neuroscientists found; for example they found that tnerurons wold respond to particular simtulis, paritulcalty neurons saw something like a vertical stripe it would fire, and if it saw a horizontal stripe, it wouldn’t fire. Inspired by this biological networks, we tried to build networks that would be able to recognize different types of things. We should think of the set networks with many level units, jus till the tnueronns, just like the brain, but you have billions of these units, they’re all conecnceted, and those connections can be large. So, if you give this network data, it will automatically change those connections to learn patterns that decsbire the data, we call those paternts features. And those features are useful to solve different tasks, os you might learn features that allow yo uno recognize an apple and udnerwtsn what an apple is, but you might recognize features which stocks are going to be more lucrative over the next five years.
(9:55) How are we going to get past where Siri is now with deep learning?
NdF: The ingredients are always the same: bigger models, more computation, more data, more people, because the more ingenious people we have trying to cross solutions, the better…Speech will keep on getting better just by scaling, I think. With dialogue – this is very new, very recent over the last few years, we’re learning how to interact these recurring networks that do input/output speech with databases, so that whatever they say is grounded on fact, is grounded on some database, fact as implied by the database; so we’re going to see a lot more of that, and I think that’s more or less where the technology is going…when you talk to an agent it will be able to sort of back up the answer with a lot of facts that exist on the web, it will know which facts to use…and this grounding is also essential for common sense understanding.
(12:50) How does the common sense nut get cracked, are we going to pull from enough Internet information to come up with a good enough consensus, are we going to have sensors out in the real world – how do we get to common sense?
NdF: I think it’s coming from everywhere at this point in time…a professor in Montreal, for example, is using Unix conversations – when people ask questions about Unix they provide them with answers – she’s using those forums to decide how to automatically answer questions…others are looking at more general facts, reasoning – for example, who was the president of the United States who was born in a state that didn’t vote for Obama the first time he was elected president; so there’s a lot of that going on right now; and then of course we also have agents, especially at DeepMind, these agents that are embedded in environments, and as they are in their environments, they start forming episodic memories, so they start remembering what they did before, and when they start interacting with the other agents, those (interactions) will be based on their own experiences in their virtual world.
(16:53) How are we working at understanding what is in a video, you mentioned the ingredients, we need more data, we need more computation…is that essentially what we’re having to work on now and then finding ways to test that…how are those innovations progressing?
NdF: I think with video, one of the big challenges is scaling; not so much with data because the data’s there, there’s so much video being produced, but we still need to figure out what the best models are for video; this is an active research area, many people are working on this, and scaling is still a challenge, scaling the models to be large enough to be able to manipulate the video, although I think bringing more engineers on the deep learning side is what’s going to save us. So, in terms of media applications, the uses are huge, but of course we’re also looking at things like how to generate video automatically, have networks that dream video; currently the networks, we’re starting to see them dreaming images…I think the dream is that eventually one day you’ll be able to dream whatever you want, and that has all sorts of implications, and you can imagine how this will vastly change the world of entertainment and beyond.
(20:45) You had said in passing, developments in deep learning will be the way that we’ll make that progression, what saves us – how do you mean that in terms of understanding video, what within deep learning itself do we need to knock away at to see that progress?
NdF: Video in a sense is no different than images, and already a lot of our models are working with video, so video is not the future, video is the present…all our agents that are playing Atari or 3D games…they’re all working with video…self-driving cars use video, so this is something that’s happening, most videos on the web have been tagged, we know what objects exist in the video, you have actors and so on, so this is happening right now…from YouTube to robotics to games…but the creation of videos further into the future – in particular, we would like to create videos in which you can control part of your dreams, have these controlled dreams where you can imagine specific things.
Related Emerj Interviews:
If you’ve enjoyed this interview with Nando de Freitas, you might be interested in these other Emerj interview articles (and accompanying audio recordings) featuring renowned deep learning and machine learning researchers and executives: