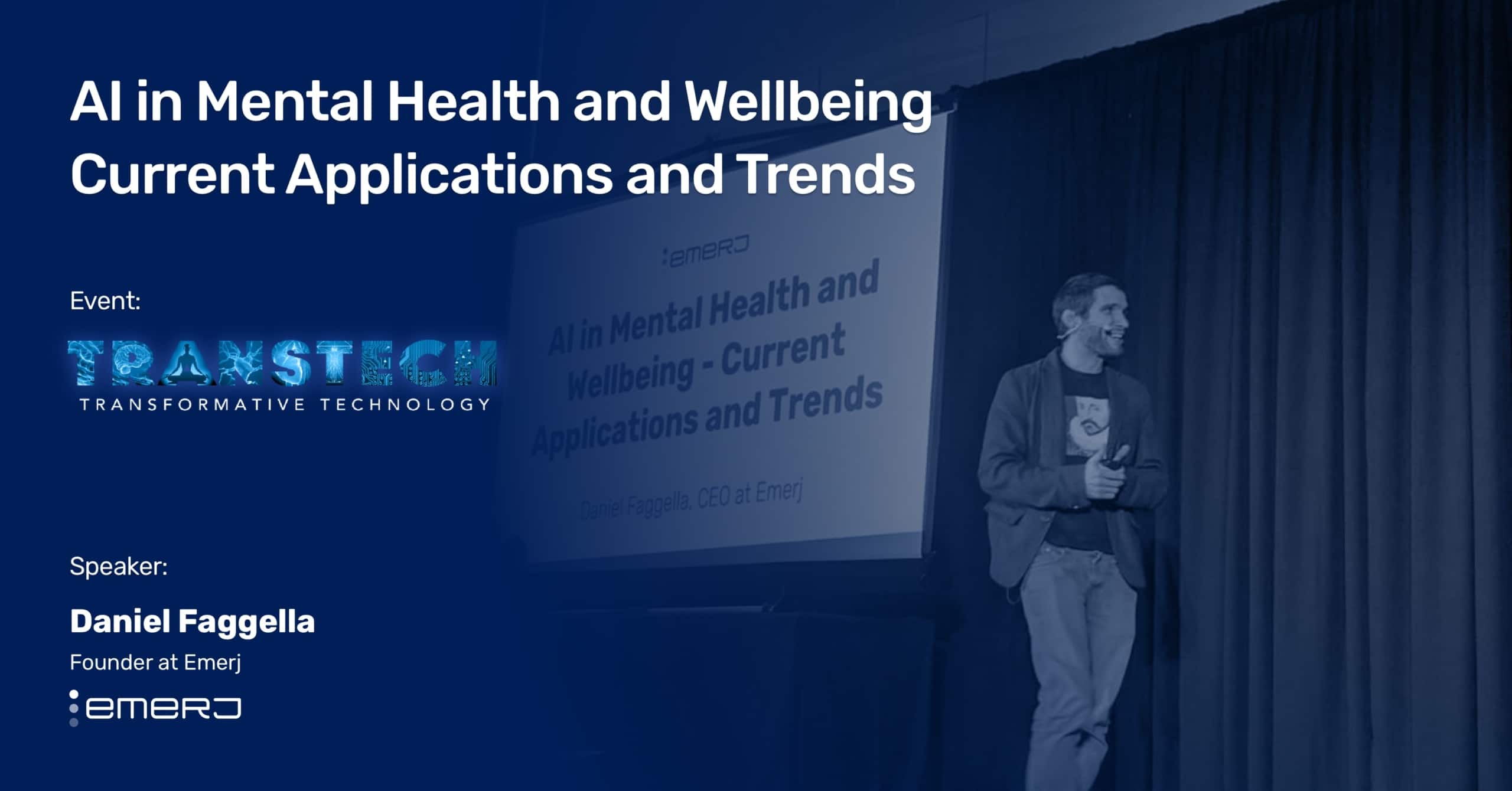
There are numerous AI initiatives in progress across the healthcare industry; some of these are for mental health and well-being. In this article, we offer an overview of how AI is facilitating mental healthcare.
We discuss patient needs that are currently unmet and how AI may meet them. We also consider the possible benefits and risks of adopting AI for mental healthcare.
This presentation is based on my presentation at the Transformative Technology Conference in Silicon Valley:
The full slide deck is also available on Slideshare:
The Unmet Needs of Mental Health and Wellbeing
By the Numbers
Funding for the National Institutes of Health (NIH) for fiscal year 2015 was $30 billion. Less than 10% of that funding was invested in mental health and addiction-related programs. This stands in contrast with research that may imply improved mental health can result in longer life and higher productivity.
In 2001, the World Health Organization (WHO) suspected that more than 10% of lost years of healthy life and 30% of all years lived with a disability can be attributed to mental health concerns. Additionally, depression is estimated to cause approximately 200 million lost workdays each year, which would cost US employers $17 to $44 billion. It is important to note that the economic effect of mental health concerns is hard to measure or quantify perfectly.
Most prominent AI vendors serving the mental healthcare space are currently offering solutions within one of two categories:
- Conversational Interfaces
- Patient Behavioral Pattern Recognition

Conversational Interfaces
Example Vendors: Wysa.io, Woebot.io, Ginger.io
Some AI vendors attempt to facilitate wellbeing by offering automated text interactions. While the technology is not made to replace a real therapist or psychiatrist, it could serve to encourage people to make appointments with and follow up on appointments with a professional.
Some vendors advertise the ability to provide tips and reminders that emphasize good sleeping habits and positive ways of thinking. These are often based on cognitive behavioral therapy and can link users to a human coach when necessary.
Currently, the limitations of creating an effective mental health chatbot are relatively steep. Some of the largest banks in the world are still having issues creating effective chatbots for simple consumer banking functions.
The comparative size of the healthcare industry would indicate that they would see even more issues trying to aggregate data and funding for this type of project.
Behavioral Pattern Recognition
Example Vendors: Marigold Health, Mindstrong, Ginger.io
The second category of AI applications in mental health also fits into a larger AI trend that will be increasingly important in the coming years. The ability to recognize behavioral patterns in patients, customers, or users of any kind is used as a solution for various business problems across industries. This emphasizes the importance of maintaining data traceability and accessibility which makes future AI initiatives easier to adopt.
Similarly to conversational interfaces, this type of AI tool could be used to help therapists and doctors find indicators of certain conditions in their patients. The machine learning algorithms behind this type of software intakes behavioral information about a patient such as internet activity or travel, and then ask them to self-assess based on that information. The software will usually ask how the patient feels that day. This way, it will be able to use this information to approximate the patient’s mental condition and determine if they need help from a therapist.
A person’s mental condition and the physical factors that might play into it can serve as important data for determining exactly what the problem might be. Currently, AI vendors have purported to track the following types of biometric data from patients:
- Exercise and sleep
- Location and movement
- Self-report assessments (daily or weekly)
- Content of text messages
- Phone use or activity
Despite the varied sources of data available for this type of project, there are still a few prominent limitations to creating an effective pattern recognition application for mental health. For example, there are many privacy concerns surrounding the application’s ability to analyze text conversations a patient has in private.
When this data is used later to train the algorithm, it will need to be properly anonymized, which can be difficult for complex situations. Additionally, the reliability of self-report data such as exercise and sleep habits is usually questionable. In the coming years, companies will need to focus on optimizing these channels for procuring data and find ways to leverage it more efficiently.
Below is an example of how MarigoldHealth describes their use of sentiment analysis technology to allow healthcare networks to better understand different populations of people:
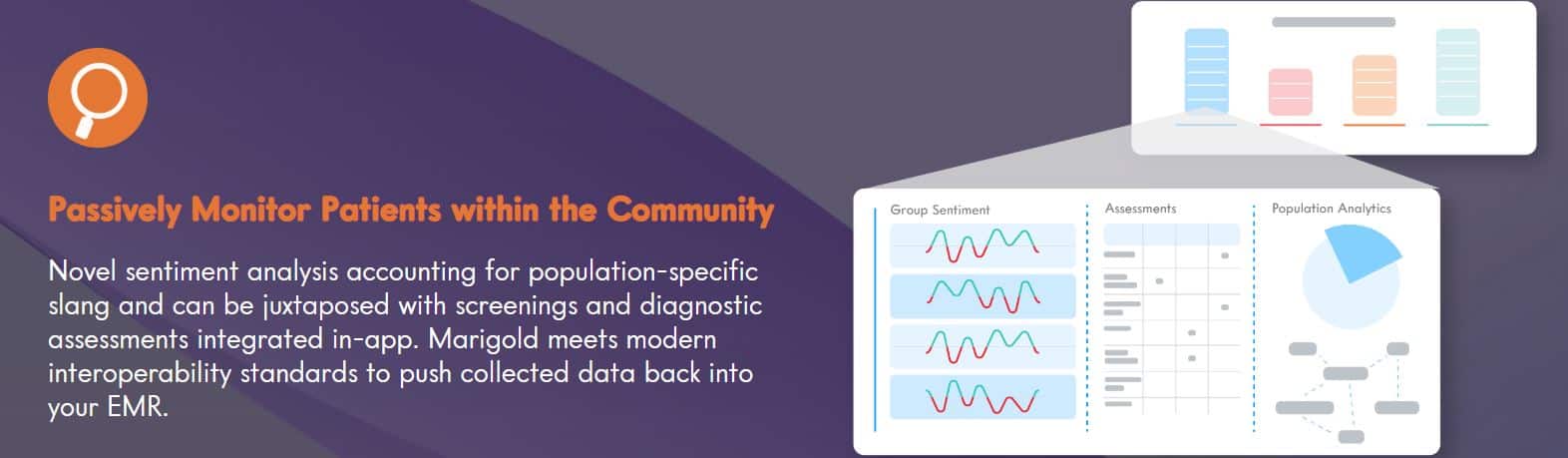
Believable Benefits and Predictions
We suspect that the core value proposition of the mental health space lies in finding patterns across new data streams. This includes data from various sources including mobile device activity, geolocational data, app use, the content of text messages, and sleep estimations.
Correlating new streams of real-time data to proxies for user wellbeing seems the most promising of the most prominent applications.
More specifically, these apps correlate data extracted from mobile device activity rather than self-assessment information. This type of app would then compare it to a given threshold, such as the frequency of suicidal thoughts or psychiatrist visits.
While it is unknown how large of a role these applications will play in offering patients direct care, this type of proxy data will likely provide much more accurate estimates of patient wellbeing as the technology improves.
It may take years to develop an AI application that can realistically suggest actions or behavior change for human patients. This is because these suggestions would require much more context than what our current mobile devices and conversational interfaces can provide, such as trauma or phobias. It is most likely best to keep the gathering this context within the purview of human doctors.
Replicating human interaction in order to suggest behavior change through chatbots will also be particularly challenging. The practice also poses a number of dangers to healthcare companies and patients, such as the numerous examples online of very disjointed or nonsensical conversation with mental healthcare chatbots.
The long term goals of these conversational interfaces may still be a long time ahead, but text is still a potential source of feedback and patient data. It could be critical for discerning higher-level risks such as their response to questions like “are you okay?” and “how have you been lately?”
Additionally, these apps could serve to help those who cannot access traditional mental health care for a variety of reasons. These include:
- People who cannot afford therapy
- People who might be too shy or ashamed to try therapy
- People in rural or remote areas with no access to a therapist
While the opportunity to help these groups in ways outside of traditional therapy, the best way to engage each patient remains unclear. It is clear from this initial problem that more research is needed in order to determine the impact of these kinds of digital treatments and diagnostic applications before they can be used as a replacement for therapy.
There is evidence that AI startups in this space seem to understand the purpose of their applications are predominantly to keep patients safe and eventually move them to human therapists when the time is right.
For example, the value proposition of the vendor Mindstrong is representative of “preemptive” insight, much like what many other firms currently speak of:
Risks to Consider
Business Model
One risk to consider when building this type of application is the incentives that drive business models away from accessible and reasonable pricing for these services. As more AI mental health firms gain traction, they will begin to consider these problems more strongly. For example, a monthly bill may subtly encourage the patient to use the service as much as they can to get all the value they can out of it.
Another problem arises with the idea that these applications are made to eventually find a therapist for potential patients. With a purpose such as this, the business model may begin to prioritize pushing patients towards therapists instead of determining with certainty that they need one.
If the business model revolves around pay per-chat conversations with remote therapists or coaches, there may be a similar propensity to refer more and more customers to these coaches. Rather than trying to help the patient by determining if they need one of these coaches, there may be a greater incentive to keep the patient engaged with either the app or a coach as many individuals as possible.
Many conversational interfaces see similar concerns to these, but in the mental health space, the risks are much higher.
AI startups making these types of applications need to find a way to become profitable, but it is currently unclear whether the for-profit incentives will bend the products in a direction that does not serve users well.
Long-term Effects
In order to determine the long term effects of virtual mental healthcare, there will need to be more research before any estimations could be considered accurate. Currently, there is not enough objective research regarding this relatively nascent AI use-case.
Additionally, the same apps are downloaded by people with varied mental health issues. Determining the potential benefits and harms of each app on patients with each type of disorder would be particularly difficult.
Another issue with discovering the long term effects of these apps is the fact that the technology users will have to interact with will change rapidly across the next decade.
As previously mentioned, healthcare companies may benefit from these apps by proxying data from them to make important determinations about individual patients. The way they will accomplish this will need to change in order to fit the capabilities of smartphones and other devices as they develop in the future.
Conclusions and Takeaways
There is still a lot of information to unearth if we can gather enough data around sleep and phone activity, and conversational interfaces might be part of that. However, it will take a considerable amount of time to achieve a level of AI technology that can suggest actions and behaviors that tangibly improve wellbeing or reduce risk.
Even within two or three years, mental health chatbot suggestions may not improve beyond the most generic and basic mental health advice. Healthcare companies and AI startups should keep their expectations low in this regard.
At the same time, it is auspicious that there are many companies, academics, and healthcare providers who are interested in this space at all. Some C-level executives have chosen to invest in these types of AI initiatives, and there are many forums and events where the potential benefits and challenges they pose can be discussed.