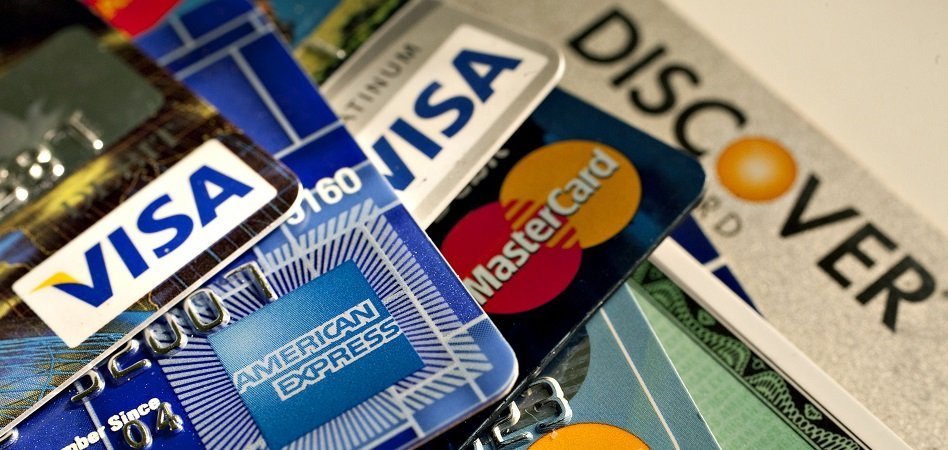
Several companies offer AI-based credit scoring applications to banks and enterprise creditors looking to better understand the risk associated with their potential borrowers. Traditional methods of credit scoring take into consideration the credit histories of potential borrows, but this might not allow certain people access to credit despite the fact that they could pay their loans back when their payments are due.
AI could allow banks and creditors to score potential borrowers on their creditworthiness using alternative data, specifically that from social media posts and Internet activity: what sites someone visits and what they purchase from eCommerce stores. Online behavior can indicate whether a person is likely to pay back their loans, and AI could allow banks and creditors to factor this into their assessments of their potential borrowers.
What Business Leaders in Banking Should Know
Artificial intelligence solutions for credit scoring more often than not are predictive analytics solutions. This makes sense given that credit scores are, in effect, scores predicting the likelihood that a customer will pay back their loans.
Generally speaking, these vendors offer software that allow banks to mine the web for information on potential customers. The software might factor in a customer’s social media posts or the sites on which they’re an active user into its assessment of the customer’s creditworthiness. The machine learning model would need to have been trained on labeled datasets indicating which kinds of social media posts or websites are indicative of a responsible customer and which are indicative of a risky customer. In order to come up with a score, the software runs all of the information it collects on the customer through its algorithm and calculates if the bank would be taking a small or large risk if it underwrote them.
This, in some cases, could provide people access to credit who wouldn’t have access to it through traditional means. Credit scores require a credit history, but many people without credit histories would be able to pay back their loans given access to credit. Banks often don’t feel comfortable lending to them, however, because they don’t have credit histories.
Again, however, some AI credit scoring software may work differently, and each software likely weighs certain online behaviors differently. For example, some companies tout their software’s natural language processing abilities. Although all of the companies in this report offer software that seem to use some amount of natural language processing to mine the social media of its potential borrowers’, some companies are more upfront about it than others. At the very least, it seems these companies consider the capability worthy of the spotlight in its marketing materials.
The companies that are selling AI credit scoring software or using it internally all seem to have the requisite talent for building and managing machine learning software, at least to some degree. This density of talent is relatively rare, especially in industries like marketing. There are also numerous vendors selling purported AI software for various applications in finance, but these companies are often lacking in the kind of AI talent we look for when vetting a company on their claims of leveraging AI.
Of the companies in this report, ZestFinance and Kreditech seem to have the highest density of data science talent. These data scientists holds Master’s and PhDs in computer science and various statistical fields and one in artificial intelligence itself. Also, Kreitech employed a Chief Data Officer with a PhD in AI from 2014 to 2018. As a result, we believe these companies have a very high likelihood of leveraging genuine machine learning software. This bodes well for them. Kreditech uses their software internally, but ZestFinance offers their software to enterprise banks.
LendoEFL employs a CTO with a PhD in Neuroscience from 2002, and the company also has several data scientists on its team. Two of these data scientists hold advanced degrees (a Master’s and a PhD), but neither in computer science. Despite, we think LendoEFL has a relatively high chance of actually offering a machine learning solution. Their company is not as robust as ZestFinance and Kreditech when it comes to AI talent, but they’re likely not lying about doing AI either.
SAS is of course the most established enterprise amongst the companies in this report, and although they certainly have the money to employ data science talent, we caution readers about automatically trusting large companies when they claim to offer AI. Many companies will hire data scientists just to say they are leveraging AI. They then fail to provide their data scientists with any meaningful work because they lack the ability to speak to them in terms they can understand. In other words, their subject-matter experts and data scientists don’t and can’t communicate well, and this is imperative for building machine learning software. The tech giants like Google, Facebook, and Amazon see success in their AI endeavors in large part because even those employees that aren’t data or computer scientists at those companies know how to speak the language in a way that allows them to inform the AI products the data scientists develop.
LenddoEFL
LenddoEFL offers a software called LenddoScore. We previously discussed Lenddo in our report on Natural Language Processing in Finance. The company claims its software can help banks determine the creditworthiness of potential borrowers using predictive analytics and natural language processing. Lenddo advertises that their software can be used to reach prospects that are unable to get credit because their software factors in data from online activity as opposed to credit history.
LenddoEFL claims users can install the company’s application onto their smartphones. The software uses natural language processing to analyze users’ social media posts and what they type into their browser for indicators of responsibility or risk-taking. Then, this information informs the predictive analytics algorithm that creates a credit score out of it. Banks and credit unions can then use the users’ LenddoScores to better understand the risk they pose of not paying back their loans.
We can infer the machine learning model behind the software was trained on thousands of customer data points including social media posts and internet browsing behavior. This data would have been labeled as indicative of responsibility or risk from the perspective of banks and creditors. The data would then be run through the software’s machine learning algorithm. This would have trained the algorithm to discern which data points correlate to creditworthiness. The software would then be able to predict which prospects are most likely to pay back their loans.
Below is a short 2-minute video demonstrating how LenddoEFL’s software works:
LenddoEFL does not make available any case studies reporting success with their software. Also, we were unable to find any mention of enterprise-level companies on LenddoEFL’s website nor in any of their press releases, but they have raised $14 Million and are backed by Golden Gate Ventures.
Naveen Agnihotri is CTO at LenddoEFL. He holds a PhD in Neuroscience from Columbia University. Previously, Agnihotri served as CTO at Milabra.
ZestFinance
ZestFinance offers a software called ZAML, which it claims can help financial agencies determine the creditworthiness of potential borrowers and reduce loan defaults using predictive analytics and natural language processing.
We can infer the machine learning model behind the software was trained on thousands of social media posts, geolocations, browsing activities, and other data points. This data would have been labeled as indicative of risk or not from a bank or creditor’s perspective. The data would then be run through the software’s machine learning algorithm. This would have trained the algorithm to discern which data points correlate to the types of borrowers that are most and least likely to pay back their loans.
In theory, the software would then be able to predict if loan applicants are likely to pay back their loans or not. That said, we could not find a video demonstrating how the software works explicitly.
ZestFinance claims to have helped Prestige Financial Services reduce lending losses and defaults without sacrificing credit approval ratings. Prestige used ZestFinance’s software to complement their traditional underwriting methods. According to the case study, Prestige saw a 33% decline in credit losses, along with a 14% increase in borrower approval ratings. ZestFinance also lists Ford Credit as one of their past clients.
Jay Budzik is CTO at ZestFinance. He holds a PhD in Computer Science from Northwestern University. Previously, Budzik served as Chief Product Officer at Kinetic Social.
Kreditech
Kreditech uses a namesake software internally, and it helps the company determine the creditworthiness of potential borrowers who do not have an extensive banking history using predictive analytics and likely natural language processing.
We can infer the machine learning model behind the software was trained on hundreds of thousands of customer data points from social media and internet browsing histories. These would involve conversations about monetary exchanges, as well as data points from eCommerce sites and payment processing sites, such as Amazon and Paypal respectively. This data would have been labeled as positive or negative indicators of responsibility and creditworthiness. The labeled data would then be run through the software’s machine learning algorithm. This would have trained the algorithm to discern the data that correlates to and creditworthiness.
A user could then feed the software with a potential borrower’s social media posts, for example, and the algorithm would search it for indicators of responsibility or creditworthiness. For instance, the software could comb through a conversation on the potnetial borrower’s social media and find that they promised a coworker that they would be paid back by a certain date. The algorithm might then label this activity as a positive indicator of responsibility.
Kreditech does not list any major companies as clients, but they have raised $497.3 Million and are backed by Rakuten and J.C. Flowers and Co.
SAS
SAS offers a software called Credit Scoring for SAS Enterprise Miner, which it claims can help banks and financial agencies predict credit risk using predictive analytics.
We can infer the machine learning model behind the software was trained on thousands of borrower profiles and credit histories. The data would then be run through the software’s machine learning algorithm. This would have trained the algorithm to discern which data points correlate to a borrower who poses a higher risk to the company than others. The software would be able to predict the amount of risk associated with a potential borrower.
We could not find a demonstration video available for the software.
SAS Institute claims to have helped Piraeus Bank Group speed up data analysis and report generation. Piraeus Bank Group integrated SAS Institute’s software into its core banking system so that it could access their data. According to the case study, Piraeus Bank Group was able to improve data analysis speed by 30%.
SAS Institute also lists Bank of America and Honda as some of its past clients.
Jim Goodnight is CEO at SAS Institute. He holds a PhD in Statistics from North Carolina State University. Goodnight has spent the last 42 years of his career at SAS Institute.
Header Image Credit: Time Magazine