Hackers are cyberattackers are using more sophisticated methods to break into digital networks; they themselves have also started employing artificial intelligence techniques to bypass detection systems.
Cyberthreats can compromise enterprise networks at a greater speed than ever before, and cybersecurity analysts alone may have difficulty responding to such large-scale threats faster than attackers. As such, banks will likely need to upgrade their security systems to keep up with the change in the landscape.
Banks stand to lose both money and their banking licenses if they inadvertently facilitate fraud and money laundering or if their digital infrastructure is compromised by a cyberattack. Perhaps most importantly, banks can take a hit to their reputations, hindering the acquisition and retention of future customers.
The Global Banking and Finance Review claims that cyberattacks have cost nearly $360 billion per year in losses every year or the past three years. In recent years, global ransomware attacks, such as WannaCry, have put financial institutions on edge, and many banks are now investing in artificial intelligence to combat hackers.
In this article we list some of the more common uses for AI in cybersecurity processes at banks using case studies from AI vendors, We run through use-cases for two different AI approaches:
- Anomaly Detection
- Natural Language Processing
We recently launched our AI in Banking Vendor Scorecard and Capability Map report, within which we categorized over 77 AI vendor products specific to the banking industry by the business function in which they apply. We found there are more AI vendors offering products for fraud and cybersecurity than there are vendors offering products for other functions in banking, as shown in the figure below:
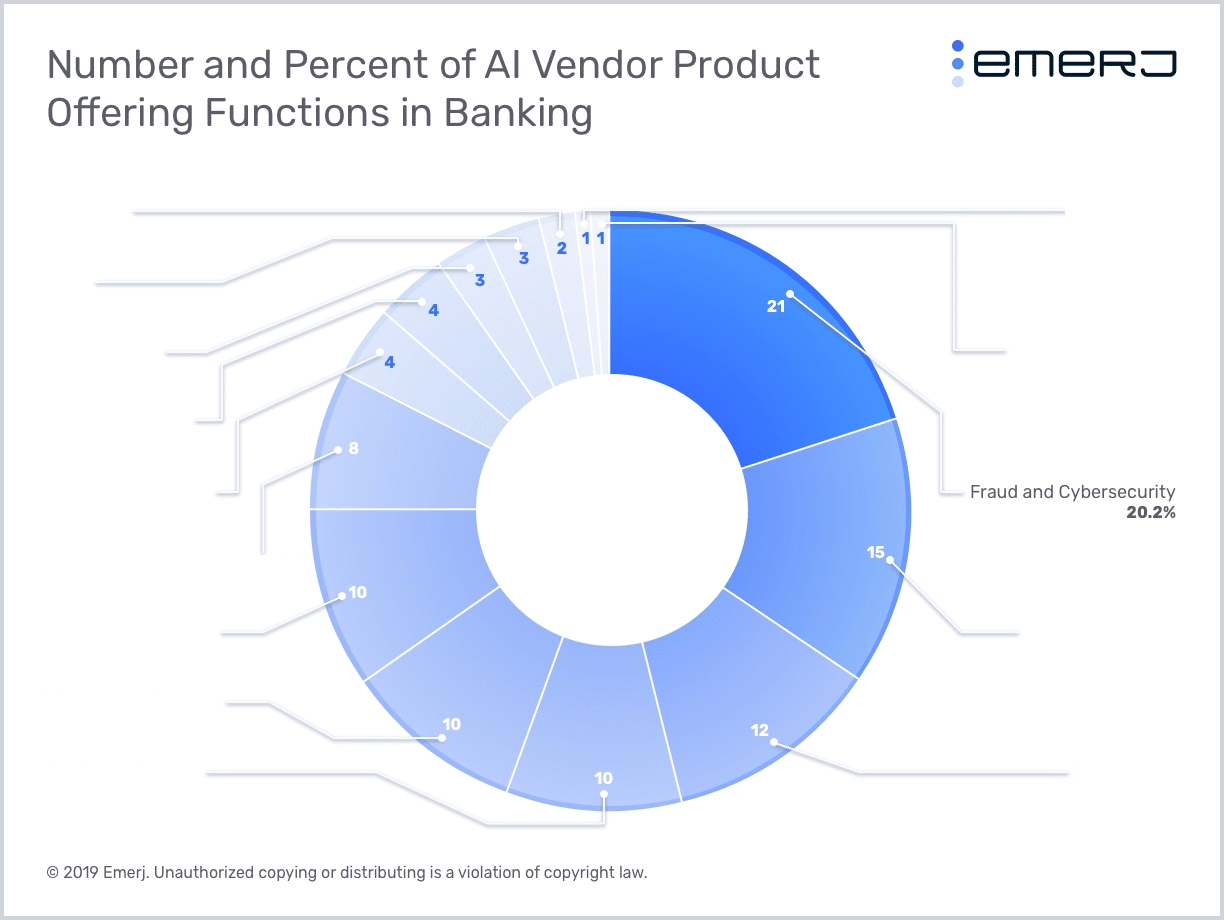
In addition, we scored these product offerings and vendors on three factors that, when summed, calculated an Overall Score: Expertise and Funding, Evidence of Adoption, and Evidence of Returns.
Fraud and Cybersecurity as a category scored a 1 on its Average Evidence of Adoption Score, indicating that banks and financial institutions are unlikely to allow fraud and cybersecurity vendors to discuss them in the vendors’ case studies and press releases.
This is likely because banks are disincentivized to discuss their cybersecurity efforts publically on two main fronts:
- Doing so could compromise their security. If a hacker knows which cybersecurity software a bank uses, they may have an easier time figuring out how to bypass it.
- When a bank discusses their cybersecurity efforts, it signals that the bank needs to invest in cybersecurity, which means they’re under attack. This could scare customers who don’t want to hear that their information is constantly under threat of being stolen by hackers.
That said, AI vendors offering cybersecurity products to banks have raised a collective $757 million, the highest among all Functions and nearly $300 million more than the Function that scored second highest in terms of Total Funds Raised: compliance.
What this means is that despite the fact that the large banks are not publicizing their cybersecurity AI projects as much, banks and venture capitalists see a fit for deploying AI in cybersecurity.
Anomaly Detection for Cybersecurity in Banking
Anomaly detection is an AI approach that can help to identify deviations from a system’s normal activity in real-time, making it a particularly useful approach in cybersecurity.
Some cyber attacks, such as phishing attempts on enterprise systems, can compromise specific user accounts in the organization. Such attacks are difficult to detect and respond to mainly because once a user account is compromised, the hackers have legitimate access to the enterprise network.
In such cases, AI software can be trained to identify the behavioral patterns for each user account or device in the organization. When hackers gain access to a user account, the way they use that account will likely be significantly different from the normal behavior of that particular user.
Vendor Profile: Darktrace
For instance, Darktrace’s Enterprise product can monitor a bank’s incoming and outgoing network communication traffic to identify patterns of abnormal behavior indicating a threat.
The video below gives an overiew of Darktrace’s Enterprise Immune System:
The company claims their software’s algorithms “learn” what normal behavior looks like when there are no instances of cyber threats and then creates flags when this behavior seems out of the ordinary at any point. The software requires a constant stream of data to run through the digital network.
AI vendors such as Darktrace claim their software can analyze raw network traffic data to understand the baseline of what normal behavior is for each user and device in an organization.
Using training datasets with user behavior information and inputs from subject matter experts who indicate to the software what “normal” patterns are and what constitutes an abnormality, the software learns to identify cyber threats and can alert the relevant cybersecurity experts at the bank.
Vendor Profile: Feedzai
Another company offering AI based cybersecurity products is Feedzai. The company claims their OpenML Engine platform can help banks with anti-money laundering and fraud detection.
The company claims their software can be integrated into a bank’s enterprise network. The software can be trained on existing cybersecurity data taken from rules-based legacy systems that have threat incidents clearly labeled.
Feedzai claims their software can potentially analyze these data streams and identify fraudulent transactions using anomaly detection to see if the transactions are significantly varying from historical customer behavior or vastly different from other customers with a similar profile.
The company claims their software also creates a “risk engine,” a score that a bank’s cybersecurity team can use to assess the most important fraud threats as opposed to spending time and resources following threat incidents without any priority order.
Feedzai’s software can purportedly alert human fraud and cybersecurity analysts with incidents that the software has categorized as high-risk (based on predefined factors), in an attempt to speed up the detection of such incidents while simultaneously reducing false positives.
As an example, Feedzai claims to have worked with an unnamed top 10 US retail bank in a project where they screened incoming customers on the basis of whether or not their applications for opening accounts were cases of fraud. The system purportedly helped the bank manually review the customers and that risk factors were clear for easy decision-making, reducing the time spent on each case.
Below is a promotional video from Feedzai explaining how its software works:
Vendor Profile: Versive
AI vendor Versive (acquired by eSentire) offers enterprise cybersecurity AI software called the VSE Versive Security Engine, which they claim can help banks and financial institutions analyze large datasets of transactions and cybersecurity-related data using machine learning.
Versive claims banks can use data from NetFlow (network protocol developed by Cisco for collecting IP traffic information and monitoring network traffic), proxy and DNS data (computer network data) as inputs to the Versive Security Engine. The software can then monitor enterprise networks using anomaly detection to alert human officers in case of deviations in the data that might be similar to events in past cyberthreats.
NLP for Cybersecurity in Banking
Phishing is a type of cyber attack in which the user accounts in an enterprise network could be compromised through email communication from fraudsters. Enterprise firms might need to monitor email communications coming in and going out of the network.
The number of emails being exchanged through an enterprise network every day might be very large, and manually scouring through each of these emails in an attempt to find any potential threats may be extremely difficult.
Natural language processing (NLP) could help cybersecurity teams at banks read through large volumes of emails automatically and identify parts of the text in emails that might indicate phishing attempts. AI is very well suited to this task and can augment the ability of banks to handle large scale data analysis.
AI software can be trained using inputs from cybersecurity experts to flag emails that seem suspicious for further review. Over time, the AI algorithms can learn to identify these threats better, allowing human experts to focus on fewer, more serious cases that might require more of their attention.
Vendor Profile: Tessian
One AI vendor offering AI-based email monitoring software for the banking sector is Tessian. The company claims they can help banks identify data breaches from misdirected emails, prevent data breaches and phishing attacks. The company’s software likely uses natural language processing and anomaly detection in different steps in order to identify which emails are likely cybersecurity threats.
Vendor Profile: Expert System
Another company offering similar AI capabilities is Expert System, which claims its Cogito platform uses natural language processing and machine learning to help banks with anti-money laundering activities. Cogito also seems able to search through a bank’s historical Suspicious Activity Reports (SARs) to better detect what might potentially constitute suspicious activity.
As an example, Expert System claims they helped Crédit Agricole Corporate and Investment Bank with automatically searching for and retrieving information from the web in one of the bank’s projects.
The promotional video below explains how Cogito gives a breakdown of some of Cogito’s features and how they work:
The Future of Cybersecurity in Banking
The industry experts that we spoke to for our report almost unanimously agreed that AI will play a big role in the future of fraud and cybersecurity. Traditional rules-based systems cannot account for new methods of fraud, and adversaries are starting to use AI themselves to hack into systems. As such, it might be necessary for banks to use machine learning to combat new cyber threats.
AI approaches such as anomaly detection and NLP are very well-suited for large-scale data handling that cannot be achieved by human experts alone. This makes AI an important tool for cybersecurity teams at banks to seriously consider in the near future.
Header image credit: Corporate Compliance Insights