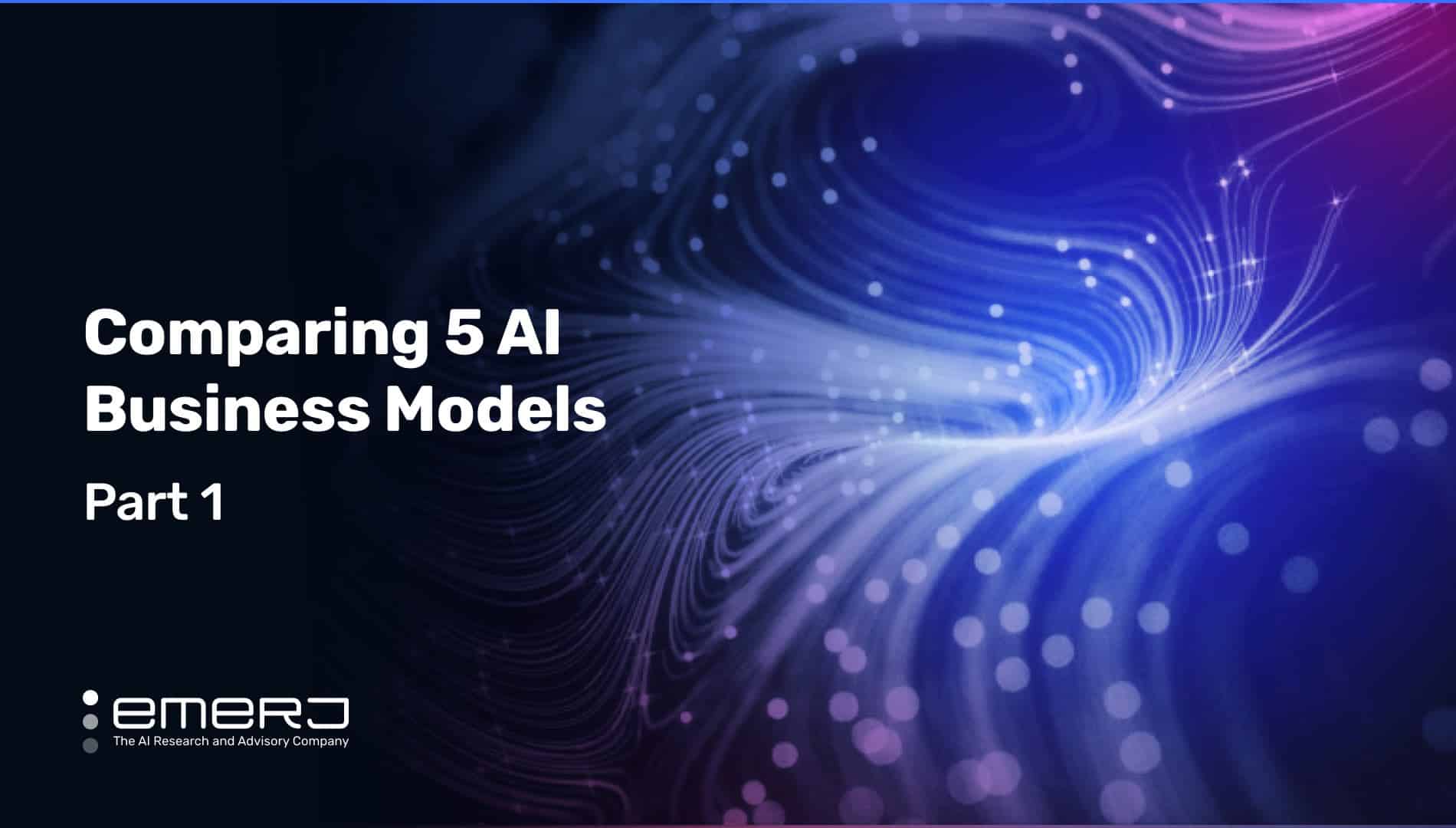
This article was a request from one of our Catalyst members. The Catalyst Advisory Program is an application-only coaching program for AI consultants and service providers. The program involves one-to-one advisory, weekly group Q-and-A with other Catalyst members, and a series of proprietary resources and frameworks to land more AI business, and deliver more value with AI projects. Learn more at emerj.com/catalyst.
Over the last five months working with Catalyst members (mostly AI product and service company execs), I’ve been forced to do a lot of thinking on the pros and cons of various AI business models.
As an AI vendor the kind of business model you choose will impact the way to market to – and serve – your clients. If you’re founding an AI companies – or thinking of spinning one out of an enterprise – this article should help you think through the type of company you want to start.
As an AI buyer the vendor type you select will have a serious impact on whether your results AI initiative is skewed towards near-term or long-term impact. If you’re in the market to buy from AI vendors, this article will help you think through which vendor type is most likely to be a fit for your goals.
Before directly comparing the five AI business models, we’ll have to cover the dichotomy between near-term value and long-term AI transformation.
AI Project Quadrant Overview
Our Emerj Plus members will recognize the Emerj AI Project Quadrant as one of our best practice frameworks:
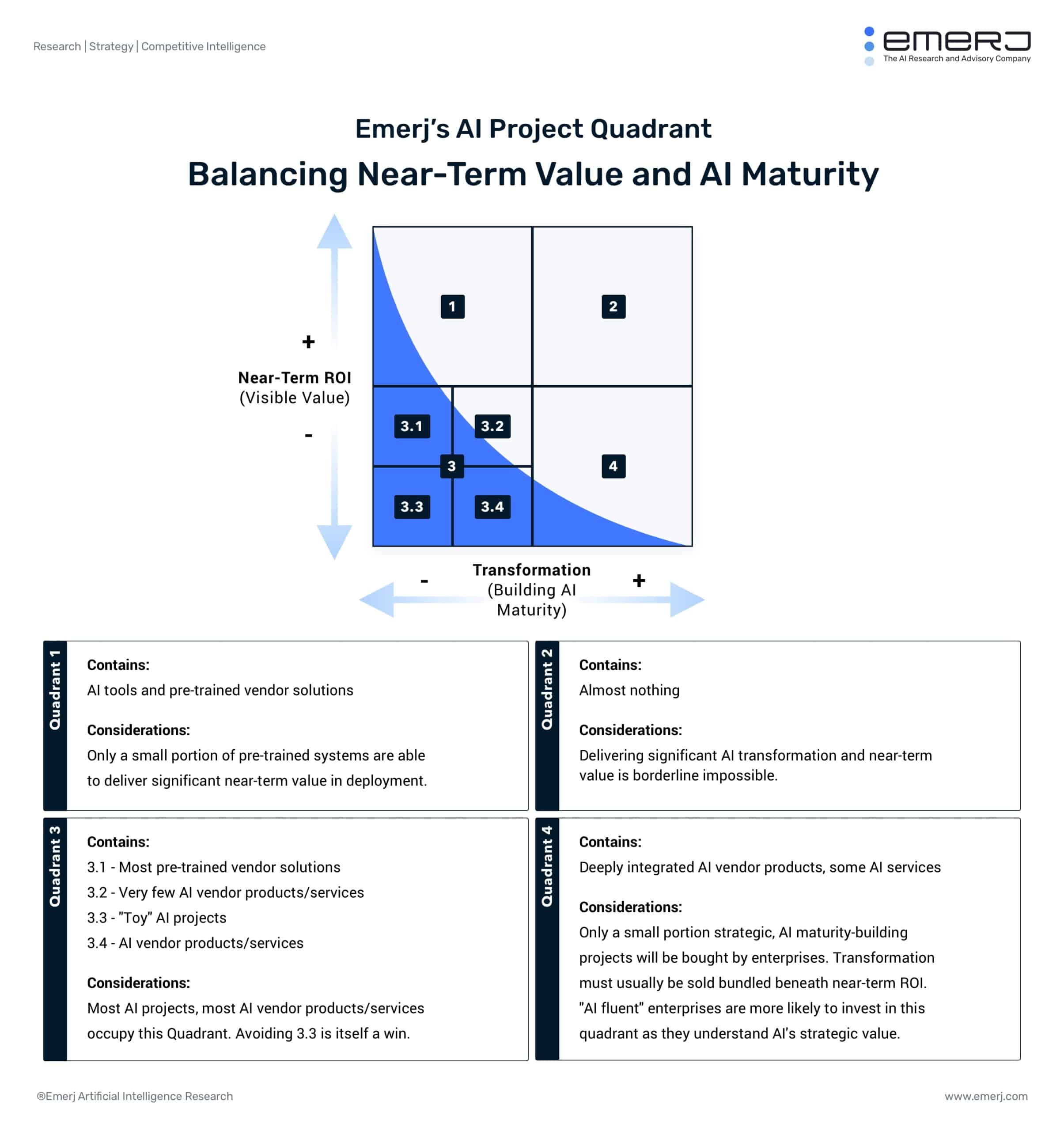
While I won’t explore all the details about this graphic in this article, I do want to make one point clear:
Building AI maturity (re-vamping data infrastructure, cleansing data stores for use with models, encouraging a culture of iteration in the enterprise, developing executive AI fluency, etc) is usually directly at odds with delivering immediate financial ROI.
Notice the absence of activity in quadrant 2.
We’ll have a lot more “takeaways” on the incentives and tendencies of different AI vendor types at the conclusion of this article. For now, let’s explore each of the most common AI vendors types in order:
Comparing 5 AI Business Models
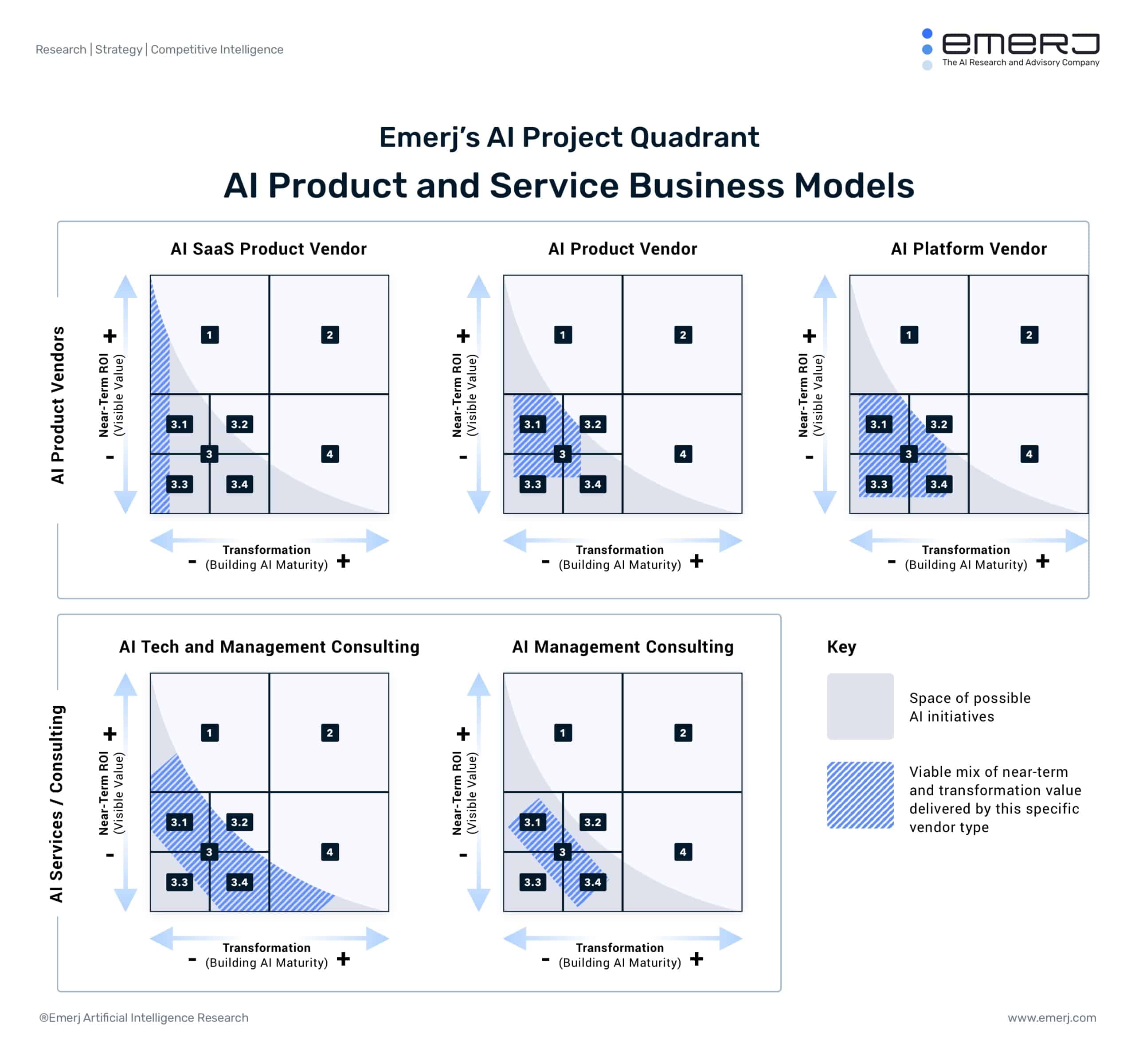
Notes about the graphic:
- The landscape of possible AI companies is vast, and a complete list (if we wanted to get a bit more granular here) of business models might run to 20 or 30 types – including crowdsourced data firms like Appen, AI ops software like Domino Data Labs, and many others. I’ve chosen these five types because they’re
- These graphics are useful generalizations, but there are exceptions. There are almost certainly some technical consulting firms with very narrow and near-term value focus, while there are also AI products that often require significant AI maturity development.
- The business models that cover more of the “space of possible initiatives” are not “better” business models, they are simply different – and involve trade-offs. AI SaaS products may be able to deliver quick results, but are unlikely to develop real AI fluency and proficiency in their client companies. Consulting firms may have a wide range of solutions they can offer, but no pre-made technical solution that solves a few problems extremely well. Consulting firms are somewhat of a commodity, but betting on specific AI functionality when building a product company can be a massive risk.
As we explore each business model type, I’ll highlight the focus and incentives for each. I’ll provide examples of companies in each category, along with interviews with the leadership of those companies when possible.
These interviews will help to add color to how each of the business model types think, and frame AI problems and solutions. You’ll hear EDITED’s CEO (under the AI SaaS section below) talk a lot about near-term value and bypassing the need to do complicated integrations, and you’ll hear the leaders of Accenture and SFL scientific (in the AI Technical and Management Consulting section below) talk much more about deeper integrations and long-term industry impact or business process change.
(1) Product Model 1 – AI SaaS Product Vendor
Value Focus:
- Near-term value (100%), AI transformation (0%)
Incentives:
- Near-term. Do as little white-glove work as possible, get the contract signed, send the invoice every year, and go focus on winning more contracts.
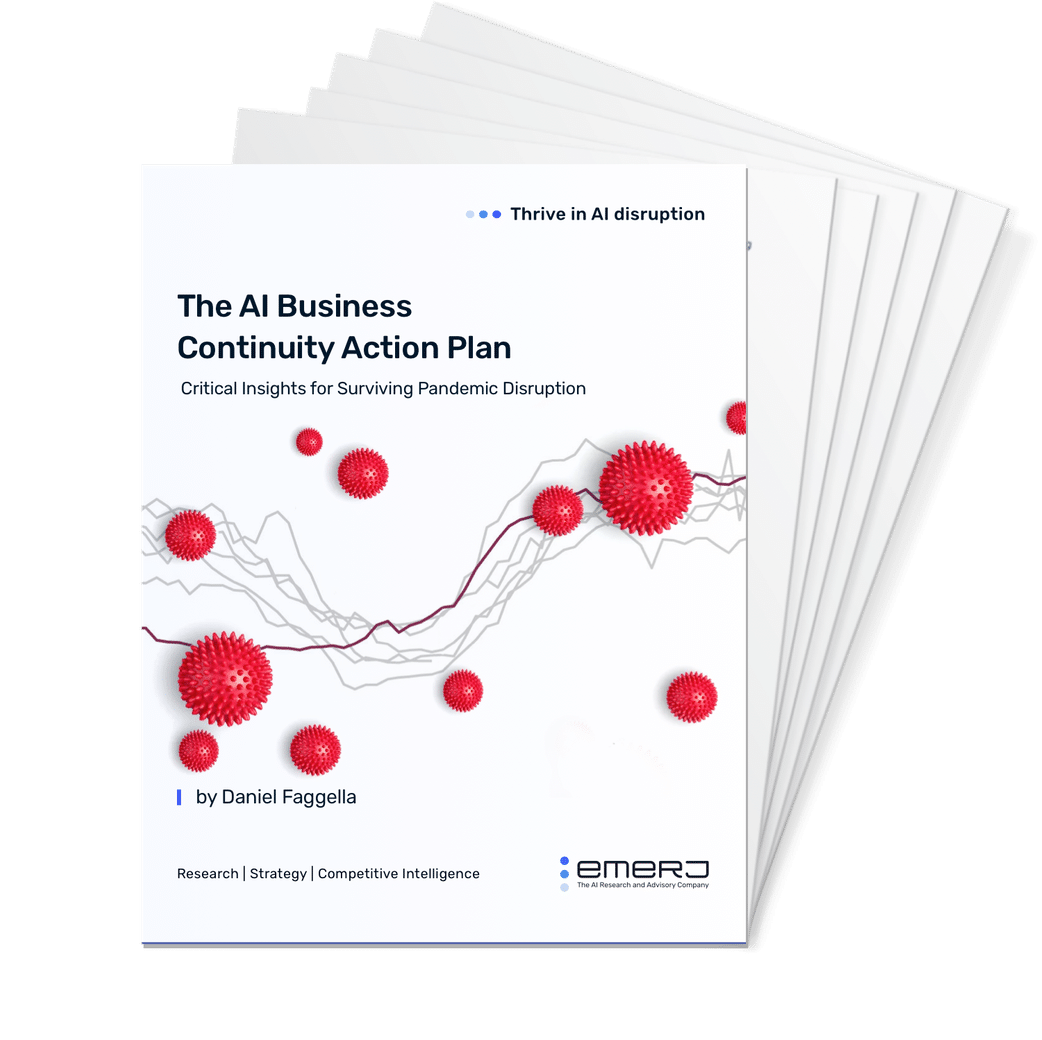
About this Model:
- Offer solutions that play at the surface of existing systems.
- Often rely on APIs, or don’t rely on integrating with a client’s existing infrastructure in any way.
- One core corpus of training data often usable for almost all clients.
- Usually require close to zero AI fluency within client companies. May involve some collaboration with client SMEs to calibrate the system, and potentially some ongoing feedback, but little more than that.
- Require almost no improvement in AI maturity on behalf of the client.
Example Firms:
- Aquabyte (Listen to our interview with Aquabyte CEO, Bryton Shang)
- Product: Underwater computer vision solution.
- Clients: Fish farms.
- User interface: Set up underwater cameras at client sites to track the behavior and health of fish. Users (fish farmers) get a dashboard indicating the number, size, and health of fish, based on computer vision analysis from within the pen.
- Data science: Done behind closed doors at Aquabyte. Clients can provide input, but training and management of algorithms is done by the vendor. Algorithms come in almost entirely pre-trained.
- Drone Deploy (Listen to our interview with Drone Deploy CEO, Nick Pinkington)
- EDITED (Listen to our interview with EDITED Data Science Lead, Alejandro Giacometti)
Back in March of 2020, I predicted the rise of AI SaaS startups – and an increasing trend towards AI vendor companies that require less integration time, and focus on near-term value, not AI maturity or transformation.
I suspect these lower-barrier-to-change firms will have an advantage in winning market share as the economy pulls out of the COVID-19 crisis. This isn’t to say that what I’m calling “AI SaaS” is a “better” business model, but that it’s one that is well suited to the pinched budgets and AI ignorance (the opposite of AI fluency) of most enterprises.
(2) Product Model 2 – AI Product Vendor
Value Focus:
- Near-term value (50-90%), AI transformation (10-50%)
Incentives:
- Near or mid-term. They want to be like AI SaaS firms (do as little white-glove work as possible, get the contract signed, send the invoice every year, and go focus on winning more contracts), but their integration challenges require longer pilots, more hands-on integration, and more hands-on maintenance. Building some AI maturity is necessary to get the sale, and building AI maturity might also help them expand their contract and do more work (i.e. win more revenue) with their clients in the long-term.
About this Model:
- Offer solutions that integrate with client systems, and use of client data.
- Data and integration requires other elements of AI maturity, including skills around cross-functional teams, and building a culture where experimentation is permitted or encouraged.
- Often involves a wider range of client applications than AI SaaS offerings. This is partially a result of being able to use the client’s own data, and partially a result of a broader tool set in general.
Example Firms:
- Kasisto (Listen to our interview with Kasisto CTO, Sasha Caskey)
- Product: Chatbot solution.
- Clients: Retail banks.
- User interface: Users within the bank can see a dashboard of the chatbot’s activity and adjust the algorithm itself (I haven’t talked with Kasisto in some time and this may not be wholly accurate). Customers of the bank interact directly with the chatbot itself, which replies to their questions and/or routes them to the proper human customer service agent.
- Data science: Clients must provide historical and real-time customer support data, as well as integrations to Kasisto’s system. Algorithms have some existing understanding of common banking terms, but must be calibrated to the unique problems and issues of the client.
- Thetaray (Listen to our interview with Thetaray CEO, Mark Gazit)
(3) Product Model 3 – AI Platform Vendor
Value Focus:
- Near-term value (30-60%), AI transformation (40-70%)
Incentives:
- Mid to long-term. These firms can’t possibly focus on near-term value alone, and training clients on their product inherently implies AI maturity. While they might use near-term “lighthouse projects” a way to encourage the sale and get momentum, they’ll make the most revenue when clients build AI capability for the long-haul.
About this Model:
- Offer a platform with more open-ended, broad use-case potential.
- Must sell clients on a broader roadmap of AI transformation, not just an individual solution. This often requires lots of education and AI maturity-building through the course of the sale and adoption of the solution. Platform vendors are incentivized to encourage AI maturity and long-term thinking in their clients.
- Must educate clients on the potential of AI, and the specific features and workflows of their platform.
- Must support clients in pursuing varied and diverse AI applications on top of their platform.
Example Firms:
- Ayasdi (Listen to our interview with Ayasdi Founder, Gunnar Carlsson)
- Dataiku (Listen to our interview with Dataiku Chief Customer Officer, Kurt Muehmel)
- Product: A data science studio that supports predictive modeling and building business applications.
- Clients: (Various enterprises / organizations)
- User interface: Varies widely depending on user. Involves a graphical user interface and various reports – almost infinitely customizable depending on use-case and industry.
- Data science: Dataiku is not handling the data science for the client – thought they help train them on the product. Their product allows firms to conduct data science experiments across their enterprise.
(4) Services Model 1 – AI Technical and Management Consulting
Value Focus:
- (Varies greatly)
Incentives:
- Mid to long-term. Smart AI technical services firms know that becoming a long-term “AI partner” means lots of future projects. Since firms with technical and management consulting capabilities can bill projects for almost any kind of project – playing the long-game is what consulting firms are likely to do.
- Opportunistic, desperate, or misinformed AI technical services firms will focus on near-term value alone, but are often unlikely to develop actual near-term ROI compared to AI SaaS vendors who have a true focus on one business problem.
About this Model:
- Potentially offer the widest range of narrow, surface-level AI tools and SaaS integrations, or consulting and strategy work, or longer-term AI maturity at a technical level (data/IT infrastructure).
- These firms are almost necessary for an enterprise-wide AI roadmap to be developed, as they potentially operate across all functional areas without being married to any specific vendor allegiance.
- Smaller firms competing for enterprise clients may need to focus on specific industries, or specific kinds of AI projects (computer vision, fraud detection, etc), though some smaller firms don’t have any such sector-specific focus (SFL Scientific).
Example Firms:
- Fractal Analytics (Listen to our interview with Fractal’s Cheif Practice Officer, Sankar Narayanan)
- Accenture (Listen to our interview with Accenture’s CTO, Paul Daugherty)
- SFL Scientific (Listen to our interview with SFL’s CEO, Michael Segala)
(5) Services Model 2 – AI Management Consulting
Value Focus:
- (Varies greatly)
Incentives:
- Mid to long-term. Strategy or management consulting firms share the incentive of their technical consulting counterparts in benefitting from becoming a trusted “AI advisor” to their clients, but they lack the ability to deliver short-term AI pilots/products – which tends to lean even more towards building a long-term AI vision, and selling their client projects along the roadmap that said vision requires.
About this Model:
- Capable of advising on strategy, education, and process consulting, but not hands-on technical AI work (development, integration, data infra).
- May need to niche more specifically in order to compete with consulting firms also capable of AI technical work.
- Can potentially find a place in nearly any AI project or initiative, but will be expected to have very specific insights – particularly into what other players in the industry are doing (this is arguably the main appeal of McKinsey and other top consulting firms).
Example Firms:
Takeaways for AI Buyers and Sellers
Insights:
- AI SaaS and AI Products have the most narrow customer base. Their focus on specific business problems mean that they’re able to offer value to a smaller total market – but they may be more prepared to win that particular market than a “generalist” AI platform or consulting vendor might be.
- The sales cycle is longer for transformation. The more transformative (in terms of AI maturity) the solution, the longer the sales cycle – and usually – the higher the price. Firms like EDITED (mentioned above, involves zero integration, limited change to workflows, no AI maturity developed) are likely going to be vastly faster than Ayasdi or Dataiku (both mentioned above), who require clients to learn AI skills and change AI, data, and business processes.
- AI product vendors will gradually shift towards AI SaaS, or AI platforms. Today, companies like Kasisto and Thetaray (mentioned above) have to do much more white-glove AI maturity-building than they’d like. If an AI product company can streamline its onboarding, simplify its integration, and pre-train its core models with other customers’ data, it will likely move towards AI SaaS and focus on winning market share faster. If it’s market is small-yet-deep (say, global top 10 pharma giants, or something similarly narrow), or if they are unable to take away the bespoke, white-glove aspect of product delivery, then they’ll want to become a “base” upon which clients can build other AI capability over time – a platform. This gravitational pull in one direction or another will play out over the next two to five years.
Insights for Sellers:
- Be mindful of vendor incentives. An AI platform vendor may help you mature your AI capabilities, but by becoming more reliant on their proprietary tech. AI product vendors generally want as little hand-holding with you as possible, and will only develop AI maturity in your organization to integrate their product and leave.
- Use consultants wisely. The right consultant can do something an AI vendor (even a platform vendor) is almost never going to do: Help you flesh out an AI vision and roadmap to maturity. This perspective – untethered from any specific vendor or technology – is essential, but it’s important to work with firms who have a proven track-record of AI strategy, not a company that tacked “AI strategy” on their list of services nine months ago to keep up with the Joneses.
Insights for Buyers:
- Founding an AI vendor company is a bit of a gamble. Services is easy – anyone can potentially find work (albeit different every time, and comparatively hard to scale) as a consultancy. Build a narrow AI solution, and you run the risk of not having product-market fit, or not finding a problem where the client data ecosystem is able to support your (informed and well-intended) hypothesized solution. Build an AI platform, and you have the massive hurdle of getting companies to rely on your nascent platform for their important AI projects (a chicken-and-egg problem).
- Determine how hands-on you want to be. Are you okay with a company that needs to hand-hold it’s clients through the fundamentals of AI maturity – or do you want to sell, quickly integrate/provide licenses, and move on? The business model you select will lean you towards a very white glove services business, or more towards a traditional SaaS sales. Almost everything except an EDITED (referenced above)-level AI SaaS product will involve more hand-holding than traditional IT solutions.
In part 2 of this short Comparing 5 AI Business Models series, we explore some of the pros and cons of these business models from the perspective of the entrepreneur or AI vendor CEO.