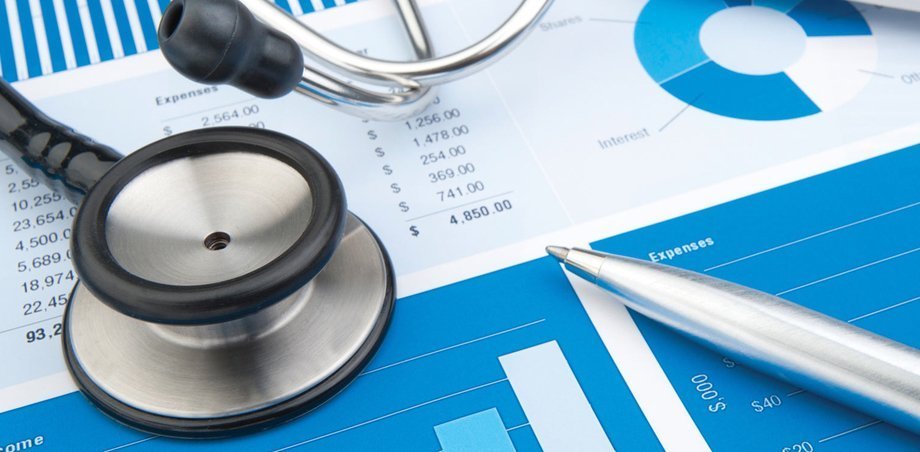
The healthcare domain seems ripe for disruption by way of artificial intelligence in the form of predictive analytics. Accenture estimates the AI in healthcare market will reach $6.6 billion by 2021.
As of now, numerous companies claim to assist hospitals and health insurers in aspects of their roles from predicting whether or not a patient will contract an illness to assessing health insurance plan risks on exchanges.
One of the key reasons for this rapid growth is the existence of vast amounts of healthcare data in the form of patient clinical data, including lab results and data from diagnoses, insurance claims data, and R&D data, such as that from published papers and clinical trials.
We researched the use of predictive analytics in healthcare to better understand where AI comes into play in the industry and to answer the following questions:
- What types of predictive analytics applications are currently in use in healthcare?
- What tangible results as predictive analytics driven in healthcare?
- Are there any common trends among these innovation efforts? How could these trends affect the future of healthcare?
This report covers vendors offering software with 3 applications:
- Predicting Risk Scores For Healthcare Insurers
- Preventing Hospital Readmissions
- Clinical Variation Management
This article intends to provide business leaders in the healthcare space with an idea of what they can currently expect from Ai in their industry. We hope that this article allows business leaders in healthcare to garner insights they can confidently relay to their executive teams so they can make informed decisions when thinking about AI adoption. At the very least, this article intends to act as a method of reducing the time business leaders in healthcare spend researching AI companies with whom they may (or may not) be interested in working.
Predicting Risk Scores For Healthcare Insurers
Apixio
Apixio also offers software called HCC Profiler, which they claim can help hospital coders sort through patient charts in electronic health records (EHRs) and present to hospital coders only the data they need for their job using machine learning. HCC profile could also allow health insurers to predict the risk of their Affordable Care Act (ACA) insurance plants.
Hospital coders add and designate codes based on the diagnoses and demographic data in a patient’s medical records. These codes are then used to determine how much the insurance companies owe the hospital.
Apixio claims hospital coders can upload patient record data from a hospital or provider’s EHR or from image scans or PDFs of patient records into the HCC Profiler system. Then, HCC Profiler seems to mine the patient record data for relevant information, including blood pressure, diagnoses, and the drugs the patient was prescribed or administered during their stay at the hospital.
According to Apixio, insurance providers can use HCC Profiler to predict the commercial risk of health plans they list on ACA health insurance exchanges. The software could potentially analyze data from patient records and medical billing statements to provide health insurers listing certain plans with an overall risk score for those plans. The company claims this could allow health insurers to better predict the costs of offering their health insurance plans and the success they might have on ACA exchanges.
It was unclear how an insurer might interact with HCC Profiler, but below is a short 2-minute video demonstrating how HCC Profiler works for hospital coders:
We could not find evidence of Apixio selling to any prominent hospital systems or insurance agencies, nor any evidence that the company’s C-level executives have robust academic or professional experience in AI at institutions and marquee companies respectively. That said, as of writing this report, the company has raised $36 million in funding and is backed by Bain Capital. Apixio also currently employs 85 people. In other words, we believe the company has traction.
Lumiata
Lumiata offers a software they claim can help hospitals and healthcare insurance providers reduce risk and improve operational efficiency using predictive analytics. The company offers solutions for:
- Risk Management: predictive analytics for identifying and predicting patterns in healthcare operations for patients with chronic conditions.
- Utilization and Underwriting Management: predicting future clinical states of patients for risk and actuarial tools
Lumiata’s software first gathers customer datasets such as Electronic Medical Records (EMR) health records and clinical lab results from hospitals. The data is then run through a deep learning model to generate a custom risk assessment for each patient.
Lumiata claims users can log into a dashboard and access insight notifications from Lumiata’s system. The software is reportedly integrated into hospital IT networks by Lumiata, which employs both data science and clinical subject matter experts. According to the company’s website, Lumiata’s predictive analytics software is trained on data from 175 million patient records and 50 million articles extracted from PubMed among other sources. It was curated by actively practicing physicians.
Healthcare and insurance customers can integrate the software’s APIs into existing risk tools.
Below is a screengrab from Lumiata’s dashboard which shows medical graphs on the right and the insights provided by the platform at the top:
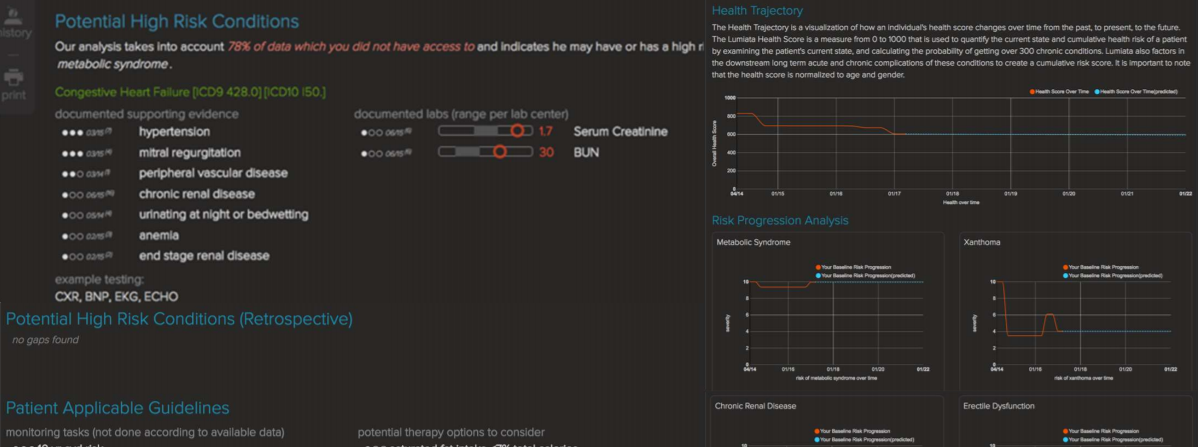
Lumiata does not make available any robust case studies reporting success with their software.
Lumiata hasn’t made clear which marquee clients they have worked with, but they are backed by several prominent venture firms, including Khosla Ventures, BlueCross BlueShield Venture Fund, Intel Capital, and Sandbox Industries.
Rohun Kshirsagar is Senior Director of Data Science at Lumiata. He holds a PhD in Pure Mathematics from Texas A&M University College.
Preventing Hospital Readmissions
Health Catalyst
Health Catalyst offers predictive analytics solutions that they claim can help reduce hospital readmission rates, predict the likelihood a patient will contract a life-threatening condition and predict the likelihood a patient will miss their appointment.
Integrating Health Catalyst software involves collaboration between the company’s data scientists or integration specialists and the hospital’s equivalents. Then, hospital employees could log into a Health Catalyst dashboard showing the likelihood a patient at the hospital might contract a CLABSI as a percentage. Doctors at the hospital could be alerted with a notification on the dashboard when a patient is deemed at high risk for a CLABSI.
This percentage is calculated with Health Catalyst’s machine learning algorithm, which is trained on a hospital’s Electronic Medical Record (EMR) data and the company’s own internal data warehouse records on CLABSI cases
The 2-minute video below gives an overview of some of the applications for their predictive analytics software:
The company claims to have worked with the University of Kansas Health System in a project aimed at reducing readmissions. The hospital system first worked alongside data science professionals from Health Catalyst to review the 30-day historical readmission data and determine that inadequate at-home care plan at discharge and advancement of chronic disease were the two major reasons for hospital readmissions.
The University of Kansas Health System reportedly needed a way to predict which patients could be classified as high-risk for chronic diseases and, therefore, which were more likely to be readmitted within a 30-day period. The hospital system used Health Catalyst’s analytics platform to do this.
Health Catalyst claims that after the integration, the university saw a 39% relative reduction in the 30-day readmissions rate for all causes, including a 52% relative reduction in the 30-day readmission rate of patients with a principal diagnosis of heart failure. It was unclear how long the integration lasted or what other readmission reduction measures were taken by the hospital at the same time.
Health Catalyst seems to have worked in several successful predictive analytics projects with Texas Children’s Hospital and Allina Health among others. Health Catalyst raised a total of $292M from firms such as UPMC and Northwest Venture Partners.
We could find no evidence of robust historical AI projects among the executive leadership team at Health Catalyst.
Clinical Variation and Population Health Management
Clinical variation is a concept in contemporary medicine dealing with studying the overuse, underuse, and different use of healthcare practices and services resulting in varying outcomes for individual patients.
Hospitals and clinics intend to reduce clinical variations by creating uniform clinical guidelines and order sets, reducing tests and procedures. In business terms, reducing clinical variation means delivering precise, cost-effective care.
Population health management is the aggregation of patient data across multiple health information technology resources, the analysis of that data into a single, actionable patient record, and the actions through which care providers can improve both clinical and financial outcomes.
Ayasdi
Ayasdi claims to offer healthcare predictive analytics solutions for clinical variation management and population health management using machine learning to analyze EMR and financial data for thousands of patient procedures.
The company claims they use machine learning algorithms that can find patterns in EMR data to cluster together similar patient procedures and generate clinical pathways that result in the best patient outcomes at the lowest costs. This helps hospitals reduce the time for clinical pathway generation compared to traditional care path generation methods which are done manually by humans.
Machine learning algorithms trained on claims and clinical data can help clinicians at hospitals can identify which care events are costing the majority of the dollars for the hospital and how much of that money is translating into positive patient outcomes. Clinicians in a hospital can access visual representations of insights derived by the analytics platform through a dashboard.
From 4:00 to 9:20 in the demo video below, Ayasdi shows how a hospital can use predictive analytics on EMR/EHR and billing data to reduce clinical variation, enhance patient outcomes, and decrease costs:
Flagler Hospital worked with Ayasdi to create a better care process model for pneumonia. Flagler Hospital claims the data set for the predictive analytics model included 1,573 patients who were discharged with pneumonia after 2014. Ayasdi gathered data from five different systems using SQL (EMR, Surgical, Analytics, EDW, Financials) and the Ayasdi CVM application to create nine potential pneumonia clinical pathways.
Two weeks after the project, Flagler was able to develop care pathways for sepsis. Flagler also reportedly increased its expected care pathways production in the next year after the pneumonia project from eight to twelve, covering cases like heart-surgery and childbirth.
Ayasdi was chosen as one of the “Technology Pioneers” by World Economic Forum in 2015 and has worked with clients such as Lockheed Martin, Credit Suisse, and Siemens. The company was founded by CEO Gurjeet Singh, a Stanford PhD in computational and mathematical engineering. He previously served at Texas Instruments and Google.
Takeaways for Business Leaders
Of all of the companies we covered, Ayasdi seemed to have the most clout. Its Predictive analytics solutions boast impressive clients. Businesses can expect predictive analytics to be applied to more applications in the healthcare domain in the next two to three years as EMR data and claims data become more structures as a norm.
We list out some of the key questions that business leaders in healthcare might need to answer before they decide to invest in AI analytics applications:
- What are the data sources for your business today? EMR data? Clinical data? Claims data?
- What are the methods of collecting that data?
- What historical data might be needed in the next two years? Is this data being collected now? If so, is it structured for use with a machine learning system?
Header Image Credit: American EHR