The implementation of artificial intelligence applications in the healthcare space is poised to impact clinical workflows, physician training and the medical education model. A recent report by Accenture predicts that the AI health market will grow to an estimated $6.6 billion by 2021 and a compound annual growth rate (CAGR) of 40 percent. This represents a significant increase from a market size of $600 million in 2014.
It must be emphasized that AI is still in its early stages of testing and adoption in the healthcare space. However, the growing amount of use-cases is evident and naturally prompts questions of how AI will impact the role of the physician in the healthcare space. It has been suggested that AI could eventually replace the physicians, but there is limited research to help healthcare business professionals navigate these claims and their potential effects.
In this article we will explore the emerging role of AI in the hospital setting, the risk of physicians being replaced and provide research on perspectives from medical professionals and AI experts.
Background
Managing patient data is a core component of the healthcare delivery process and machine learning and natural language processing are increasingly being explored in the healthcare data management function. The evolution of the Electronic Medical Record (EMR) or the Electronic Health Record (EHR) has contributed to the need for more robust data management methods.
In 2009, the Health Information Technology for Economic and Clinical Health (HITECH) Act was enacted to “promote the adoption and meaningful use of health information technology.” As a result, the healthcare industry experienced a notable shift from paper-based to computer-based patient health records.
Main objectives of meaningful use include increased efficiency of healthcare delivery and better clinical outcomes. It is defined within four broad categories:
- Improve quality, safety, efficiency, and reduce health disparities
- Engage patients and family
- Improve care coordination, and population and public health
- Maintain privacy and security of patient health information
For example, from 2009 to 2015 the percentage of U.S. Non-Federal Acute Care Hospitals utilizing a basic EHR systems increased from 12.2 percent to 83.8 percent. 96 percent of these hospitals have implemented a certified EHR system meeting certain standards (such as data privacy measures) as defined by the U.S. Centers for Medicare & Medicaid Services.
As of January 2018, a total of $24.8 billion in Medicare and $12.6 billon Medicaid EHR Incentive Program payments were distributed to eligible providers enrolled in the EHR Incentive Programs.
However, the rapid progress of national EHR implementation has also introduced some new challenges to the field. Results from a national study of 6,375 physicians conducted by researchers at Mayo Clinic found that physicians using “EHRs (84.5 percent) were less likely to be satisfied with the amount of time spent on clerical tasks.”
While there are many factors which may directly or indirectly contribute to “physician burnout” managing the growing amounts of clerical and clinical data (such as EHRs) seems to be a leading factor. The anticipated shortage of 100,000 physicians by 2030 in the U.S. as reported by the Association of American Medical Colleges (AAMC) adds another level of pressure to healthcare providers and medical institutions to keep pace with the growing patient population.
The Role of the Physician in the AI Era
Benefits
Noted author, Stanford physician, and professor, Abraham Verghese, MD is among professionals who do not view AI as a threat to the physician workforce but rather as an opportunity to integrate the expertise of human clinicians and intelligent automation.
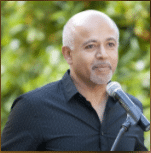
Dr. Verghese recently expressed his perspective in a co-authored opinion piece for the Journal of the American Medical Association (JAMA). In the article, he and Stanford colleagues emphasize how AI may be particularly useful in handling clerical tasks.
“The 2 cultures – computer and physician – must work together. For example, clinicians are biased toward optimistic prediction, often overestimating life expectancy by a factor of 5, while predictive models trained from a vast amount of data do better; using these well calibrated probability estimates of an outcome, physicians can then work appropriately for patients at the highest risk…
The ability of artificial intelligence to automate and help in the clerical functions (such as servicing the EMR) that now take up so much of a clinician’s time would also be welcome.”
– Abraham Verghese, MD et al., JAMA (January 2018)
The authors argue that data quality must be prioritized when implementing AI as “bad data” may contribute to a cascade of inaccurate clinical data, putting patients at risk.
In fact, it has been suggested that healthcare systems already possessing an effective data collection strategy may be better suited to implement AI-driven data management systems. In a conversation with Emerj, Sangeeta Chakraborty, Chief Customer Officer at Ayasdi, illustrates this perspective:
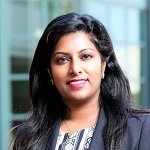
Source: LinkedIn
“The industry is going to have [hospitals] go in that direction because they’re focusing on the quality…so it is inevitable. Some of them are ahead of the curve where they have EMRs in place where they can access data in terms of all the clinical and operational things that have happened to a patient.
They have a system where they can set it up and they’re willing to look at this and talk to their physicians and say by no means is that replacing anybody. We are only here to augment the physician. This is all data for them to look at, make sure they have confidence because we ensure there is transparency in everything we provide. Then they can say ‘I’m interested to use this because this is new learning’.”
– Sangeeta Chakraborty, interview with Emerj (2017)
Another important consideration is the type of data that is being collected to be processed by an AI platform, as in the case of structured vs. unstructured data. Structured data generally refers to information typically sent to EHRs such as diseases that have a universal code and can be referenced by the SNOMED CT (Systematized Nomenclature of Medicine—Clinical Terms). For example, “Type I diabetes mellitus without complication (disorder)” would be coded as SNOMED CT code 313435000.
In contrast, unstructured data involves physicians recording narratives in their own way, not necessarily following a specific method. From these examples, it becomes more evident why training a machine learning algorithm on unstructured data would be quite challenging, and structured data would be more efficient.
Abraham Verghese, MD and colleagues also discuss the future potential of automating patient charts using natural language processing, and how the ability of AI to reduce physicians’ clerical duties could improve the quality and time spent with patients and other members of the healthcare team.
Thus the perspective illustrated here is one of partnership with AI and not one of replacement of physicians. However, there will most certainly be push back and concerns from healthcare providers, which must also be carefully considered.
Factors which may influence resistance to adaptation may include the limited amount of scientific literature on the clinical value of AI applications and the lack of inclusion of AI in standard clinical training models.
Challenges
In an interview with Emerj,
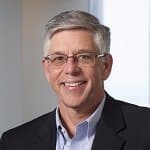
Source: LinkedIn
(an investment firm in the life science space), helps shed light on the challenges AI companies navigate when entering the healthcare space. Gullans’ expertise includes advanced life science technologies and former faculty positions at Harvard Medical School and Brigham and Women’s Hospital for nearly two decades.
“…There are some applications right now where physicians don’t enjoy a particular kind of call or one where having some assistance can actually be a big benefit to everyone involved…
I think what you’re going to see is very specific populations within a particular setting, such as calling a stroke in the ER as bleeding or non-bleeding, where there’s a life and death decision that’s very binary…but other applications will take a little more time, and obviously the AI is needed most where the decision-making is hardest, so there’s going to be reluctance to adopt it.”
– Steve Gullans, PhD, Interview with Emerj
Dr. Saurabh Jha, Associate Professor of Radiology at the Hospital
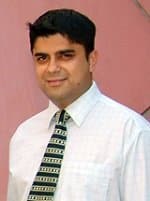
Source: University of Pennsylvania
of the University of Pennsylvania offers a perspective on the impact of AI on physician specialities. He sees AI replacing the work that radiologists currently do as “a mathematical certainty.” However, Jha also argues that the timeline for implementation is not as easy to determine.
“Workforce planning for organized radiology is tricky. That AI will do the job radiologists do today is a mathematical certainty. The question is when. If it were within 6 months, radiologists may as well fall on their swords today. A reasonable timeframe is anything between 10 and 40 years, but closer to 10 years.
AI may increase radiologists’ work in the beginning as it spits out false positives to dodge false negatives. I consulted R. Nick Bryan, MD, PhD, emeritus professor at the University of Pennsylvania, who believes that radiologists will adjudicate normal. The arc of history bends toward irony…The past belonged to sensitivity; the future is specificity. People are tired of being told that they have “possible disease.” They want to know if they’re normal.”
– Saurabh Jha, MD, Will Computers Replace Radiologists? (2016)
While Jha envisions a significant implementation of AI in his specialty, which may reduce the number of jobs, he still believes that human radiologists will still be needed to inform interpretation of data and delivery of care.
Curtis Langlotz, MD, PhD, a professor of radiology and medicine at Stanford and researcher of machine learning and deep learning radiology applications suggests that AI will become a requirement for the specialty. In fact, Langlotz has stated that “radiologists who use AI will replace radiologists who don’t.”
Looking to the Future
Educating the healthcare workforce on the potential value of AI is crucial. In fact, researchers have suggested that such education should begin as early as medical school.
In 2016, Humanitas University announced its collaboration with IBM Watson to integrate its Medical Cognitive Tutor into the medical school curriculum. Trained on large data sets including “scientific literature, de-identified information from patients, EMRs and Humanitas Doctors experience (best practices),” the objective is to personalize the learning experience and improve the efficiency of instruction.
In theory, students receiving instruction integrating real-world data would be more prepared to navigate data complexities and would have a stronger understanding of applications of machine learning.
Similarly, the Human Diagnosis Project (Human Dx), an open source collaboration between 7,500 doctors and 500 medical institutions, is a global project that claims machine learning is used to improve patient diagnoses and improve clinical outcomes. Partners and data contributors include the American Medical Association (AMA), Harvard Medical School and Massachusetts Institute of Technology (MIT).
A collection of teaching scenarios based on data collected through Human Dx is distributed every day to subscribers in a format called the Global Morning Report (GMR). According to the American Association of Medical Colleges (AAMC), approximately 40 percent of U.S. internal medicine residency programs currently receive the GMR.
As the role of the physician evolves in the era of AI, the humanity of healthcare delivery will remain critical. Rituals that may been deprioritized in favor of clerical tasks may reemerge with a newfound appreciation that emphasizes critical skills such as teamwork and shared decision making.
In general, AI applications are only as robust as the data they are trained on. Additionally, the interpretation of recommendations proposed by these systems require the expertise of the humans who will make the ultimate decision.
Conclusion – Automation: Risk or Reward?
It appears that there are several important factors to consider regarding the impact of AI in the healthcare industry and if it could eventually lead to the replacement of physicians.
We aimed to summarize these insights below to provide clinicians and healthcare executives with an at-a-glance perspective on the major factors poised to impact the industry:
Risks
- AI is capable of processing larger amounts of data and at a faster rate than human clinicians
- AI is capable of achieving a higher level of accuracy and is not subject to fatigue or burnout
- AI is capable of impacting workforce capacity due to its ability handle a large capacity of tasks that are well suited for automation
Rewards
- AI may enhance clinician productivity; predictive models trained on large amounts of data are capable of more accurately predicting patient outcomes
- AI may reduce physicians’ clerical duties thus improving the quality and time spent with patients and other members of the healthcare team
- AI lacks human sensitivity; clinical applications still require human expertise in the interpretation of data and recommendations
Header image credit: Adobe Stock