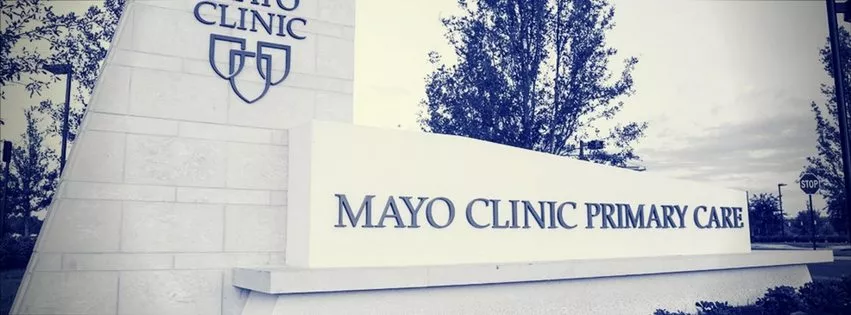
Despite the massive venture investments going into healthcare AI applications, there’s little evidence of hospitals using machine learning in real-world applications. We decided that this topic is worth covering in depth since any changes to the healthcare system directly impact business leaders in multiple facets such as employee insurance coverage or hospital administration policies.
Industry analysts estimate that the AI health market is poised to reach $6.6 billion by 2021 and by 2026 can potentially save the U.S. healthcare economy $150 billion in annual savings. However, no sources have taken a comprehensive look at machine learning applications at America’s leading hospitals.
In this article we set out to answer questions that business leaders are asking today:
- What types of machine learning applications are currently in use and which are in the works at top hospitals such as Massachusetts General Hospital and Johns Hopkins Hospital?
- Are there any common trends among their innovation efforts – and how could these trends affect the future of healthcare?
- How much has been invested in machine learning and emerging tech innovation across leading hospitals?
This article aims to present a succinct picture of the implementation of machine learning by the five leading hospitals in the U.S. based on the 2016-2017 U.S. News and World Report Best Hospitals Honor Roll rankings. (While a respected industry source, we acknowledge that the Honor Roll ranking methodology may not fully represent the complexities of every hospital, we’re simply using the ranking as a means to finding a representative set of high-performing hospitals).
Through facts and figures we aim to provide pertinent insights for business leaders and professionals interested in how these top five US hospitals are being impacted by AI.
Before presenting the applications at each of the top five hospitals, we’ll take a look at some common themes that emerged from our research in this sector.
Machine learning in Hospitals – Insights Up Front
Judging by the current machine learning initiatives of the top five US hospitals, the most popular hospital AI applications appear to be:
- Predictive analytics – The ability to monitor patients and prevent patient emergencies before they occur, by analyzing data for key indicators (see Cleveland Clinic’s partnership with Microsoft and Johns Hopkins’ partnership with GE below)
- Chatbots – Automating physician inquiries and routing physicians to the proper specialist, (see UCLA’s “Virtual Interventional Radiologist (VIR)” case below)
- Predictive health trackers – The ability to monitor patients health status using real-time data collection (see Mayo Clinic’s investment in AliveCor)
In the full article below, we’ll explore the AI applications of each hospital individually. It’s important to note that most of the applications of AI at major hospitals are relatively new, and few of them have distinct results on their the improvements or efficiencies that these technologies allowed for. We tried our best to exclude AI use-cases that seemed more like PR stunts than actual genuine applications and initiatives.
In either case, you’ll see in the article below that we’re very clear about which applications have traction, and which have no results to speak of thus far. We’ll begin with the #1 ranked report in the Best Hospitals Honor Roll, the Mayo Clinic.
Mayo Clinic
In January 2017, Mayo Clinic’s Center for Individualized Medicine teamed up with Tempus, a health tech startup focused on developing personalized cancer care using a machine learning platform. The partnership involves Tempus conducting “molecular sequencing and analysis for 1,000 Mayo Clinic patients participating in studies relating to immunotherapy” for a number of cancer types including “lung cancer, melanoma, bladder cancer, breast cancer and lymphoma.”
While currently in the R&D phase, the initial goal is to use the results of these analyses to help inform more customized treatment options for Mayo’s cancer patients. Mayo joins a small consortium of healthcare organizations in partnerships with Tempus including University of Michigan, University of Pennsylvania and Rush University Medical Center.
“The holy grail that we’re looking for, and that Tempus is actively trying to build, is a library of data big enough that these patterns become a therapeutic, meaning you can start to say, ‘People that have this particular mutation shouldn’t take this drug, people that have this particular mutation should take this drug’” -Eric Lefkofsky, Tempus Co-Founder and CEO
Tapping into the estimated $13.8 billion DNA sequencing product market, the startup apparently follows two compensation models depending on client type: Tempus charges hospital systems directly for their services and in the case of individuals or patients, the costs are billed to the insurance provider. Tempus CEO, Eric Lefkofsky is also co-founder of eCommerce giant Groupon and a handful of tech companies with analytics software leanings including Uptake Technologies and Mediaocean.
According to the CDC, cancer is rivaled only by heart disease which is the leading cause of death in the U.S. and in March 2017, Mayo Clinic in conjunction with medical device maker Omron Healthcare made a $30 million Series D investment in heart health startup AliveCor.
Kardio Pro, designed by AliveCor, is an AI-powered platform designed for clinicians “to monitor patients for the early detection of atrial fibrillation, the most common cardiac arrhythmia that leads to a five times greater risk of stroke.” Kardia Mobile, AliveCor’s flagship product, is a mobile-enabled EKG. Results of the Kardio Pro investment have yet to be reported.
Cleveland Clinic
In September 2016, Microsoft announced a collaboration with Cleveland Clinic to help the medical center “identify potential at-risk patients under ICU care.” Researchers used Cortana, Microsoft’s AI digital assistant, to tap into predictive and advanced analytics.
Used by 126 million Windows 10 users each month, Cortana is part of Microsoft’s Intelligent Cloud segment which increased by 6 percent or $1.3 billion in revenue according to the company’s 2016 annual report.
Cortana is integrated into Cleveland Clinic’s eHospital system, a type of command center first launched in 2014 that currently monitors “100 beds in six ICUs” from 7pm to 7am. While improved patient outcomes have been reported by William Morris, MD, Associate CIO, specific improvement measures have not been released.
The Microsoft-Cleveland Clinic partnership is focused on identifying patients at high risk for cardiac arrest. Vasopressors are a medication administered to patients in the event of a cardiac arrest. While part of a “pulseless sudden cardiac arrest management protocol,” vasopressors also raise blood pressure. Researchers aim to predict whether or not a patient will require vasopressors.
Data collected from monitored ICUs is stored in Microsoft’s Azure SQL Database, a cloud-based database designed for app developers. Data collection points such as patient vitals and lab data are also fed into the system. A computer model is built from the data that integrates machine learning for predictive analysis.
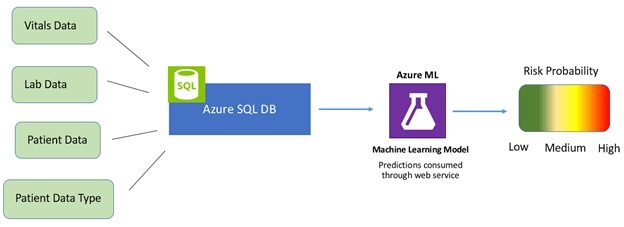
Massachusetts General Hospital
Currently in the early stages of its AI strategy, in April 2016 NVIDIA announced its affiliation with the Massachusetts General Hospital Clinical Data Science Center as a “founding technology partner.” The Center aims to serve as a hub for AI applications in healthcare for the “detection, diagnosis, treatment and management of diseases.”
Officially presented at the 2016 GPU Technology Conference, NVIDIA DGX-1 is described by the company as a “deep learning supercomputer” and was installed at Mass General (readers unfamiliar with GPU technology may be interested in our NVIDIA executive interview titled “What is a GPU?“). The NVIDIA DGX-1 reportedly costs $129,000.
With a hospital database comprised of “10 billion medical images” the server will be initially trained on this data for applications in radiology and pathology. The Center aims to later expand to electronic health records (EHRs) and genomics. If NVIDIA DGX-1 delivers on its promises it could mitigate some of the challenges currently facing the field:
“If we can somehow seamlessly capture the relevant data in a highly structured, thorough, repetitive, granular method, we remove that burden from the physician. The physician is happier, we save the patient money and we get the kind of data we need to do the game-changing AI work.” -Will Jack, Co-founder and CEO of Remedy Health
Johns Hopkins Hospital
In March 2016, Johns Hopkins Hospital announced the launch of a hospital command center that uses predictive analytics to support a more efficient operational flow. The hospital teamed up with GE Healthcare Partners to design the Judy Reitz Capacity Command Center which receives “500 messages per minute” and integrates data from “14 different Johns Hopkins IT systems” across 22 high-resolution, touch-screen enabled computer monitors.
A team of 24 command center staff is able to identify and mitigate risk, “prioritize activity for the benefit of all patients, and trigger interventions to accelerate patient flow.” Since the launch of the command center Johns Hopkins reports a 60 percent improvement in the ability to admit patients “with complex medical conditions” from the surrounding region and country at large.
The hospital also reports faster ambulance dispatches, 30 percent faster bed assignments in the emergency department, and a 21 percent increase in patient discharges before noon among other improvements.
Johns Hopkins leaders recently convened in April 2017 for a two day discussion on how to leverage big data and AI in the area of Precision Medicine. Industry analysts estimate the global Precision Medicine market value at $173 billion by 2024 (see our full article on AI applications in medicine for more use-cases in medicine and pharma).
While specific details have not been released, a talk on “how artificial intelligence and deep learning are informing patient diagnosis and management” was presented by three speakers including a VP for IBM Watson Health Group, Shahram Ebadollahi, and Sachi Saria, PhD, Assistant Professor of Computer Science.
Saria’s research on machine learning applications to improve patient diagnoses and outcomes was recently presented at the 11th Annual Machine Learning Symposium at the New York Academy of Sciences.
UCLA Medical Center
In March 2017 in Washington, D.C., UCLA researchers Dr. Edward Lee and Dr. Kevin Seals presented the research behind the design of their Virtual Interventional Radiologist (VIR) at the Society of Interventional Radiology’s annual conference. Essentially a chatbot, the VIR “automatically communicates with referring clinicians and quickly provides evidence-based answers to frequently asked questions.”
Currently in testing mode, this first VIR prototype is being used by a small team of UCLA health professionals which includes “hospitalists, radiation oncologists and interventional radiologists” (readers with a deeper interest in cancer treatments may want to read our full article about deep learning applications for oncology). The AI-driven application provides the referring physician with the ability to communicate information to the patient such as an overview of an interventional radiology treatment or next steps in a treatment plan, all in real-time.
VIR was built on a foundation of over 2,000 example data points designed to mirror questions that commonly come up during a consultation with an interventional radiologist. Responses are not limited to text in format and may include “websites, infographics, and custom programs.”
The research team integrated VIR with natural language processing ability using the IBM Watson AI system. In the tradition of customer service chatbots across industries, if VIR cannot provide an adequate response to a particular inquiry the chatbot provides the referring clinician with contact information for a human interventional radiologist.
With increased use the researchers aim to expand the functionality of the application, for “general physicians interfacing with other specialists, such as cardiologists and neurosurgeons.”
In March 2016, UCLA university-based researchers, were published in Nature Scientific Reports with a study combining a special microscope with a deep learning computer program “to identify cancer cells with over 95 percent accuracy.”
Photo (see Photonic time stretch microscope): http://newsroom.ucla.edu/releases/microscope-uses-artificial-intelligence-to-find-cancer-cells-more-efficiently
The photonic time stretch microscope, invented by Barham Jalali, the research team’s lead scientist, produces high resolution images and is capable of analyzing 36 million images per second. Deep learning is then used to “distinguish cancer cells from healthy white blood cells.”
Blood based-diagnostics are a growing sector and an increasingly competitive space as discussed in a recent Emerj interview with the founder of a leading firm investing in early-stage tech startups:
“…Looking at DNA, RNA, proteins, all kinds of biomarker information to diagnose someone as early as possible is definitely a very active area…for example if you look at Grail, the very large spinout from Illumina, they’re very well-funded and they’re trying to use DNA and other information from the blood to be able to detect cancer early.” – Shelley Zhuang, Founder and Managing Partner, Eleven Two Capital
Concluding Thoughts on Machine Learning at the Top 5 Hospitals
It’s important to note that healthcare machine learning applications (unlike other applications in – say – detecting credit card fraud or optimizing marketing campaigns) struggle with unique constraints. Treating patients is a more delicate procedure than testing an eCommerce up-sell, and with regulatory compliance and a multitude of complex stakeholder relationships (doctors to use the technology, hospital execs to buy it, patients to hopefully benefit from it), we must be somewhat sympathetic with these top hospitals for not having tangible results from AI applications in such a touchy and new field.
Our interview with health-tech investor Dr. Steve Gullans covered the unique challenges of hospital AI applications in greater depth. Steve mentioned the overt fear that many specialist physicians feel around AI tools, and the other psychological factors that will likely make hospital adoption slow.
When asked how hospitals and healthcare facilities might get around these barriers (assuming the technology will in fact better the lives of patients), he expressed his opinion on where adoptions opportunities may exist:
“It is tough, but there’s always a few beachheads that are going to pay off; there are some applications right now where physicians don’t enjoy a particular kind of call or one where having some assistance can actually be a big benefit to everyone involved…I think what you’re going to see is very specific populations within a particular setting, such as calling a stroke in the ER as bleeding or non-bleeding, where there’s a life and death decision that’s very binary…” – Dr. Steve Gullans, Excel Venture Management
It’s also important to note that we should remain skeptical of technology applications until quantifiable results can be verified. As in nearly all other AI-infused industries, machine learning in healthcare is resulting in plenty of “technology signaling” (the hyped-up touting of “AI” for the sake of garnering attention and press, and not actually for improving an organizations results).
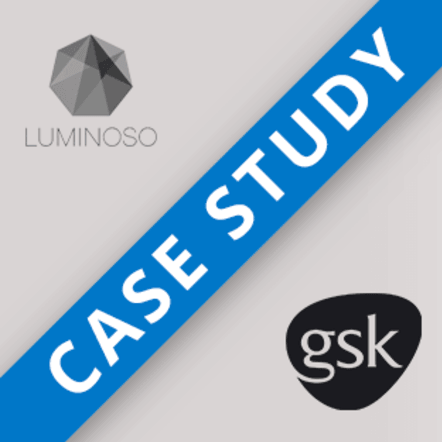
It’s mutually advantageous for an AI vendor like NVIDIA or Microsoft to grant a “new and super-fancy” AI technology to a top hospital, and grant the hospital the title of “founding technology partner.”
These kinds of events are nearly guaranteed to get press and (probably) reflect favorably upon both parties – whether or not the AI application ever drives results for either the hospital (efficiencies) or patients (better health outcomes).
We certainly didn’t compose this article to insult the efforts of any of the hospitals or vendors involved, but we as analysts must remain skeptical until results can be determined. Our aim is to inform business readers (like yourself) of the applications and implications of AI, and we always prefer projects with recorded results rather than “initiatives.”
That being said, it’s important for business leaders to understand the common trends of such developments to get a sense of the “pulse” of an industry, and we hope to have done just that in this article.
One of the reasons we insist that vendor companies list a client company and quantifiable result in our Emerj AI case studies is because – as with any emerging technology – AI is often used as a signal for the “cutting edge,” a tool for hype and not function.
We’re of the belief that some of the hospital AI applications highlighted in this article will in fact make their way to real and ubiquitous use (particularly those which we highlighted in our “insights upfront” section at the beginning of this article). Just when fruitful applications will become commonplace – time will tell.
Image credit: Static1