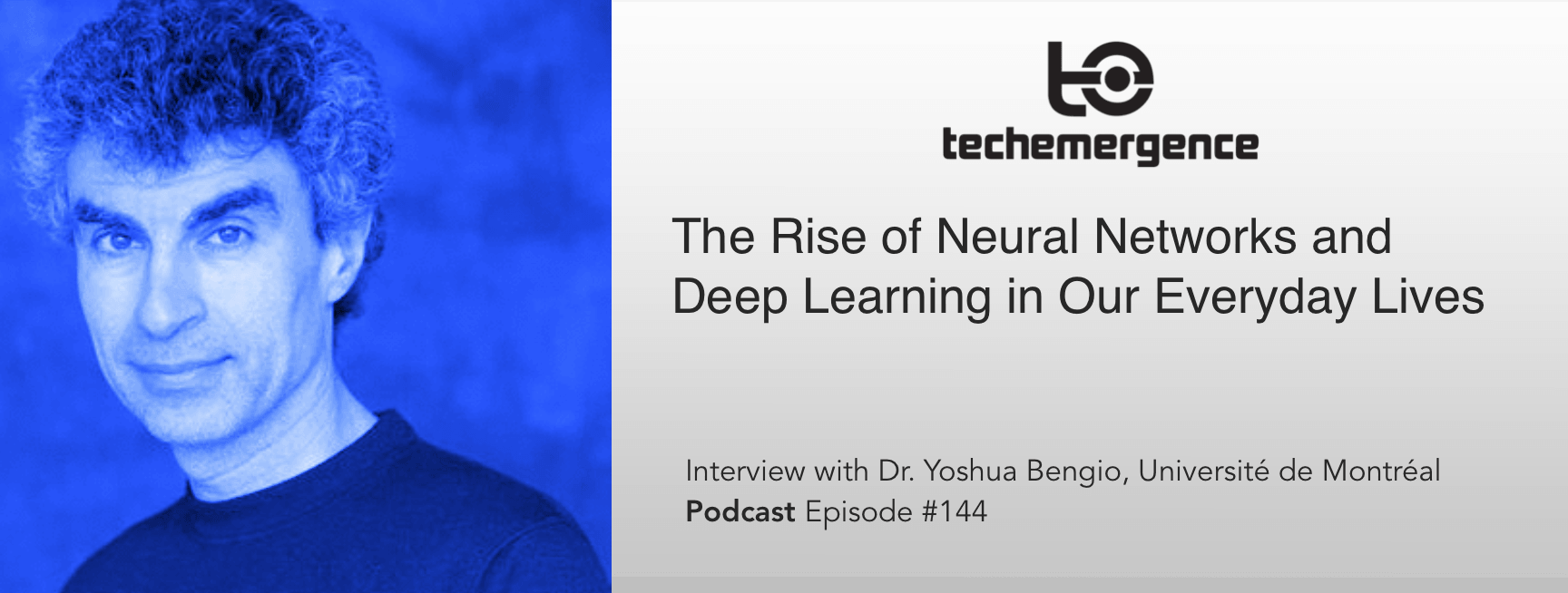
[This interview has been revised and updated.]
Episode Summary: How do neural networks affect your life? There’s the one that you walk around with in your head of course, but the one in your pocket is an almost constant presence as well. In this episode, we speak with Dr. Yoshua Bengio about how the neural nets in computer software have become more ubiquitous and powerful, with deep learning algorithms and neural nets permeating research and commercial applications over the past decade. He also discusses likely future opportunities for deep learning in areas such as natural language processing and individualized medicine. Bengio was a researcher at Bell Labs with Yann LeCun, now at Facebook, and was working on neural nets before they were the “cool” new AI technology as it’s often perceived today.
Guest: Yoshua Bengio
Expertise: Computer Science and Artificial Intelligence
Recognition in Brief: Yoshua Bengio is Full Professor of the Department of Computer Science and Operations Research at Université de Montréal. His main research ambition is to understand principles of learning that yield intelligence. His research is widely cited (over 22,000 citations found by Google Scholar in early 2015, with an H-index of 60). Yoshua Bengio was Program Chair for NIPS’2008 and General Chair for NIPS’2009 (NIPS is the flagship conference in the areas of learning algorithms and neural computation). Since 1999, he has been co-organizing the Learning Workshop with Yann LeCun, with whom he has also created the International Conference on Representation Learning (ICLR).
Current Affiliations: Professor at Université de Montréal and head of the Machine Learning Laboratory (MILA); CIFAR Program co-director of the CIFAR Neural Computation and Adaptive Perception program; Canada Research Chair in Statistical Learning Algorithms; NSERC-Ubisoft industrial chair; action editor for the Journal of Machine Learning Research; associate editor for the Neural Computation journal; and editor for Foundations and Trends in Machine Learning
Advocating for Neural Networks
Though they’re the makings of exciting news in AI today, there’s been a major shift in the world of neural networks over the past decade. The study of them went out of fashion around 2005 until a small group of researchers, including Dr. Yoshua Bengio, decided that something needed to do be done to revive them because it was “the right thing to do,” says Bengio.
In response, Yoshua and other scientists formed a research program funded by CIFAR, or the Canadian Institute for Advanced Research, which focused on deep learning and discovered ways to transition the neural nets of old to the deep learning networks of today.
Deep neural nets have more layers of representation, which allow them to capture more abstract concepts and the computer to make better decisions. While this change-over happened about 10 years ago, Yoshua notes that it took about 5 years to get neural nets to show impressive results, first in speech recognition, then computer vision, and more recently in natural language processing. The resulting algorithms have sparked a revolution in academic and industrial applications.
By 2012, those with an android already had neural nets on their phone and in server that boosted speech recognition capabilities. Advancements in computer vision followed closely on speech recognition’s heels, with the introduction of convolutional neural networks (CNN) as a solution for object recognition. CNNs are specialized for images, and within the last couple of years neural nets have improved to the point of computers that are able to recognize thousands of image categories, including human faces.
As far as applications in the field are concerned, advances in computer vision have miles of potential, with many companies and institutions – including Massachusetts General Hospital – starting to use this technology in the areas of image detection and diagnostics. Bengio explained,
“The important thing with deep learning and machine learning in general is it needs a lot of data to train on, so a computer learns to do a task like recognizing an object in an image or identifying that there is a cancer cell or recognizing which word you’re saying when you’re speaking by looking at millions of examples, and one reason why neural nets didn’t catch on earlier is that we didn’t have that much data in the 90s.”
This influx of data paired with much more powerful computers, while still tiny in parameters compared to real brains, was the key to unlocking deep learning’s capacities. Yoshua stated,
“Something happened around 2009, which is the use of graphics processing units (GPUs) by researchers in deep learning that suddenly changed the game, it allowed us to train models that were…10 to 20 times bigger and we just passed a threshold thanks to this extra computation power.”
During the 1990s when Bengio was in graduate school, a typical data set might consist of handwritten digits that were a 32 x 32 image; today, algorithms deal with resolutions like 256 x 256. “Video is something which we still don’t have enough computing power, but within a few years we’ll see more applications within the video area because of extra computing power,” said Bengio.
A Deepening Field with Far-reaching Applications
While the future remains to be seen, Yoshua believes that one of the big upcoming revolutions will be in the area of natural language understanding, specifically in getting a computer to understand the meaning of a written word.
“Computers are now pretty good at understanding images, but in terms of understanding the meaning of a sentence, a paragraph, a document, we still have a lot of progress to make, and there’s now a lot of people around the world designing new algorithms based on deep learning for exactly that.”
While some in AI thought neural nets would never be good for much outside of pattern recognition, researchers are discovering that special forms of recurrent neural networks can be trained to reason sequentially, combine evidence, and use the output for actions like understanding and generating dialogue. This means that people will, at some point, be able to talk to their computers, which will not only translate but understand what a person is talking about most of the time, in simple language.
Another big change, though less obvious for people to see day to day, are improved use of algorithms in a machine learning function called supervised learning. In simple form, this works by data scientists telling the computer what semantic content is contained in images and documents, often thousands of images over time (i.e. ‘here’s an image and it contains a cat’).
The problem with supervised learning is that there’s a limit to the human capacity to label the millions of pieces of data available. “The way we learn is just by observing the world, we don’t have somebody sitting near us and telling us every second..this was the right thing to do,” said Yoshua. We do of course receive feedback and give feedback to children, but it’s a very small fraction of the learning experience.
“Getting information from unlabeled data, what we call unsupervised learning, is a very hot topic right now but clearly not something we have cracked yet, something that is still in the column of challenges.”
While it’s difficult to put dates on progress, Yoshua also believes it’s likely that we’ll see lives greatly changed by advancements with neural networks in the medical domain, both on the research end – understanding how molecules interact with each other and the body and the development of better drugs, for example – as well as in the burgeoning arena of personalized medicine.
“We can find the treatment that’s most appropriate for you with all your history and all the test results we have for you, which isn’t what exactly what’s happening right now…maybe you need a different dosage, or maybe it should be combined with another drug, there’s lots of things specific to you that are not being taken into account right now,” explained Bengio
This is an area in which he believes we’ll start to see a great impact on people’s lives; in fact, it’s one in which he is currently working and would like to see more researchers dedicating their efforts. “I feel like this is the kind of application where we can directly help people, not just by building better gadgets but by directly influencing the health of humans.”
Image credit: Ivado