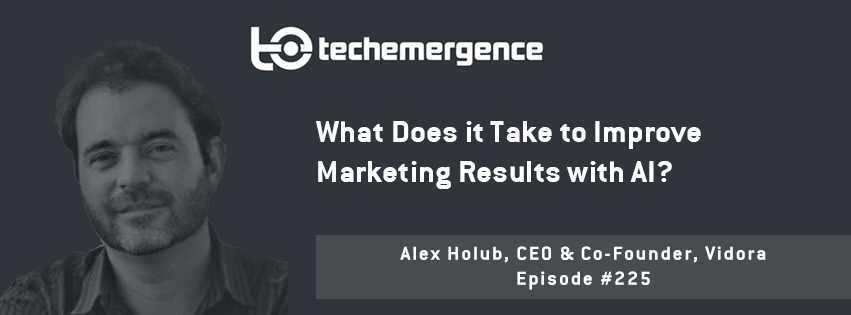
Episode Summary: In this episode we speak with Co-founder and CEO Alex Holub of Vidora about how businesses, particularly in the digital and B2C spaces, can improve marketing results with AI. Holub discusses the resources needed—time, money, in-house or outside expertise, calibration, and data—in order to leverage AI in a realistic way. It’s safe to say that today, some businesses are not yet set up to be leveraging AI, while others should be seriously considering taking the leap to using machine learning in their marketing processes. Holub draws some firm lines as to what kinds of businesses are primed to take advantage of AI, and what it takes to flip the switch and make AI a useful and inspired revenue driver in the marketing domain. Many of Holub’s useful insights are echoed in our machine learning in marketing consensus from last month, also worth reading if you’re interested in additional first-hand perspectives from executives using AI in the marketing space. Alex was introduced to us by our friends are BootstrapLabs.
Expertise: Machine learning, predictive analytics, and product management
Brief Recognition: Alex studied artificial intelligence at Cornell University and earned his Ph.D at Caltech. He has published over 15 academic papers and holds numerous patents in the areas of machine learning, computer vision, and artificial intelligence. Prior to co-founding Vidora, Alex was a technical and product management lead at Ooyala.
Current Affiliations: Co-founder and CEO of Vidora
Big Ideas:
1 – AI in Marketing Isn’t for Every Business, and Here’s Three Reasons Why
AI is changing how business is done in marketing and how companies relate to and interact with their customers. From real-time, personalized push notifications, to personalized email content for an individual user, AI is turning outreach and targeting processes that might have taken months into hours. Holub gives the fundamental building blocks for businesses considering leveraging machine learning (ML) in their marketing processes. He emphasizes that all three of the following points need to be in place to realistically garner any real value from using ML technology.
Turning Insight into Action:
1 – You need to have a defined problem, with a defined set of parameters, for which data is consistently being collected. At its most basic level, finding solutions to well-defined, rich data problems is where ML shines.
2 – You need the talent in-house, at least some of it (i.e. one data scientist), or you can’t realistically set up and work with AI as part of your systems. Holub notes that even this often isn’t enough—what’s in high demand now are people who can interface with a machine learning system and who know how to operationalize the data to automate business processes. In our previous executive consensus about machine learning misconceptions, it became apparent that underestimating the need for specific ML talent is common.
3 – You need huge volumes of transaction data and data that leads to transactions in order for ML systems to glean value. This is why businesses predicated on mostly digital marketing channels (volumes of data), businesses that are bigger (volume of data), and businesses selling B2C (higher transaction volumes, more “sales” events to train the system on) are all more likely to “win” with ML technology.
Interview Highlights:
The following is a condensed version of the full audio interview, which is available in the above links on Emerj’s SoundCloud and iTunes stations.
(1:45) What does it take to improve marketing results with AI? I think a good place to start is where AI is currently driving results. Where do you running a marketing company see it making a difference in the bottom line in the marketing world?
Alex Holub: We focus on online businesses, those that have a heavy online component, it’s probably useful to put that in context in terms of what’s made AI possible now, in order to understand what domains AI can really be applied in…what we find when we talk to a lot of online companies is they’ve begun to consolidate their data, the data that exists about their customers…at a fundamental level what’s really driving AI is that this data is available and so one of the beauties that’s always been thought about when we talk about the online world is this ability to understand each user in so much more detail…when we think about where AI can make the biggest impact, I think for right now its businesses that have a large number of users and a lot of data that’s been consolidated about those users.
(4:05) It sounds like for you, the more your engagements and interactions are tracked digitally, and the more touch points that are tracked, and the higher volume, particularly for transactions…the more likely that AI will be able to wiggle its way into your marketing process, is this safe to say?
AH: I think that’s spot on. It’s the amount of data you have on those users. Data is really the lifeblood of most AI algorithms, and the more data you have on those users across a broader set of users, the more effective these algorithms and technology are really going to be…
…there’s been a dramatic increase in the scale of data that’s been available, and that’s driven a lot of exciting advancements in AI. Specifically, I consider AI to be a super domain of what’s machine learning, which is a discipline within AI, and machine learning really has been what’s driving a lot of this AI revolution that we’ve seen recently. But some of the specific areas within marketing—”martech”—have really grown because of this data and because of these kind of intelligence mechanisms that sit on top of that data, so one of these areas I think is business intelligence (BI), and when you think of BI…you think of mechanisms that may have traditionally been stored on big spreadsheets, but that now can really be fed into these AI algorithms, which can tell you for every user in real time what their probability of churn is, what their probability of up-sell is, etc., so this traditional discipline of BI has really just been transformed by big data and by combining it with AI.
(6:56) BI has some far-reaching ramifications, particularly in the marketing space…what are the other aspects that BI touches on, tasks or results that could put words to how BI is beinginfluence by this influx of ML?
AH: I think a broad category that I’ve seen a lot of advances in is basically anomaly detection. You’re running a business, you want to know, is there something out of the ordinary happening in my business; traditionally you might look at a dashboard…now there’s technology out there that can say, there’s something unusual happening with your business today, this is something you should look at. While BI in the past was much more reactive, now you can be much more proactive, and I think in general BI as a discipline is changing and evolving and I think we’re seeing a merging of BI and customer relationship management that wasn’t possible before…
…there’s a variety of areas that are being transformed, everything from how we send out emails, what the content of those emails are. There’s companies leveraging AI and ML technology, not just in the personalized content of emails, but actually personalizing the verbiage; (for example), what should that header be…all of this can be driven by big data, all of this can be driven by AI, and the notion to keep in mind is machine learning will find the optimal way to engage each user if you give it a chance.
(10:48) What are the time requirements, the money requirements, the talent requirements…that are actually going to be needed in order for someone to step forward…what are the base line requirements to get something off the ground and tune the machine to be able to legitimately improve results?
AH: One of the things you’ll see in the industry is you’ll see folks like Google, Amazon, coming out with these open-source machine learning platforms, and the message that they’re sending out to the ecosystem is, look we’ve created platforms that will allow anyone to get up and running with basic machine learning and AI technology; so they’re looking to really enable any organization to leverage a combination of their cloud services with these AI libraries. What we see when we really start talking to businesses is, there is a level of expertise that’s really needed in order to drive results from these different AI technologies…what sort of features to pump into these systems—say you have data on a user, how do you map that data into the ML algorithm? And without that expertise we see a lot of folks getting a lot of suboptimal results…
(13:07) Do we need a whole department for this kind of thing, do we need a guy or two—what’s realistic within a department or business for this kind of talent?
AH: I think there’s extremes, so if you want to do everything in-house…one of the first things you’re going to do is rank out the highest priority areas for your data scientists to work out, so you’ll need at the minimum one data scientist, but just being able to crunch data and produce algorithms usually isn’t enough for most organizations, because you want to be able to automate certain aspects of your organization. So, you can think of two different components—someone that’s working on the data, someone that’s mapping machine learning to the data—but then you need folks that can operationalize that information, and those folks will often have a data bent, and understand how to interface with these machine learning systems, how to make them real-time, responsive, and automated, and those two sets of skills are in high demand right now…
(19:19) What are the facets of the marketing process that humans really still own, are responsible for in the creative and operational side, and then what are the aspects that the machine tackles? How do you like to frame that so people understand what it looks like when you start applying this?
AH: When you think about where machine learning…is really having the biggest impact, is humans basically frame the problem and almost frame a parameter set, which the machine learning optimizes over…a very simple parameter might be when to send a push notification, and to whom to send a particular push notification…that’s where we see machine learning being most effective, when there’s a defined set of parameters, that the machine learning algorithm can learn, take in more data, iterate and get better and better at figuring out who should be sent this particular notification…
…but what machine learning is not really doing right now as effectively is, say you wanted to generate the text to write to a particular user or you wanted to figure out whether you should run a campaign at all…machine learning right now wouldn’t bee able to think strategically and understand enough about the world to say, okay we should be running campaigns that have a lot of red in them over the holidays, because that’s a Christmas color, so the creatives and the strategy right now are still sitting with humans who are defining that, but really then it’s the parameters of how those campaigns look that are being optimized, but what’s really fascinating is the domain of machines expanding almost on a weekly basis…I think in the foreseeable future, humans will define strategy, but the domains of what’s possible for machines to do is going to expand