Our sector-wide research suggests that natural language processing (NLP) is one of the more common AI approaches in banking AI use-cases today. Sentiment analysis is a capability of NLP which involves the determining whether a segment of open-ended natural language text (which can be transcribed from audio) is positive, negative, or neutral towards the topic being discussed.
AI-based sentiment analysis systems use NLP and machine learning to quantify (as a positive number, negative number, or zero) sentiments by looking for topics, themes, and categories within sentences. Large banks can use AI-based sentiment analysis software to gauge customer opinions about their brand or their products in an attempt to improve customer experiences.
We recently launched our AI in Banking Vendor Landscape and Capability Map report, in which we categorized over 77 different AI product offerings in the banking space. We worked with industry experts and PhDs in AI to categorize AI vendor products based on the technical type of artificial intelligence or machine learning behind an AI product.
In our report, we defined an AI approach as the technical type of artificial intelligence or machine learning behind an AI product. In other words, a product’s AI approach is a description of the AI algorithms that make its value proposition possible. AI Approaches include natural language processing, computer vision, anomaly detection, predictive analytics, and prescriptive analytics.
Through the course of our research, we found several use-cases that claimed to use NLP. With input from our AI expert German (should we mention him here?) we further classified NLP as having five sub-approaches, namely:
- NLP (Information Retrieval)
- NLP (Intent Parsing)
- NLP (Sentiment Analysis)
- NLP (Speech Recognition)
- NLP (Classification)
We defined sentiment analysis as follows: determining the tone and inferred opinions (positive or negative) behind human language in text form.
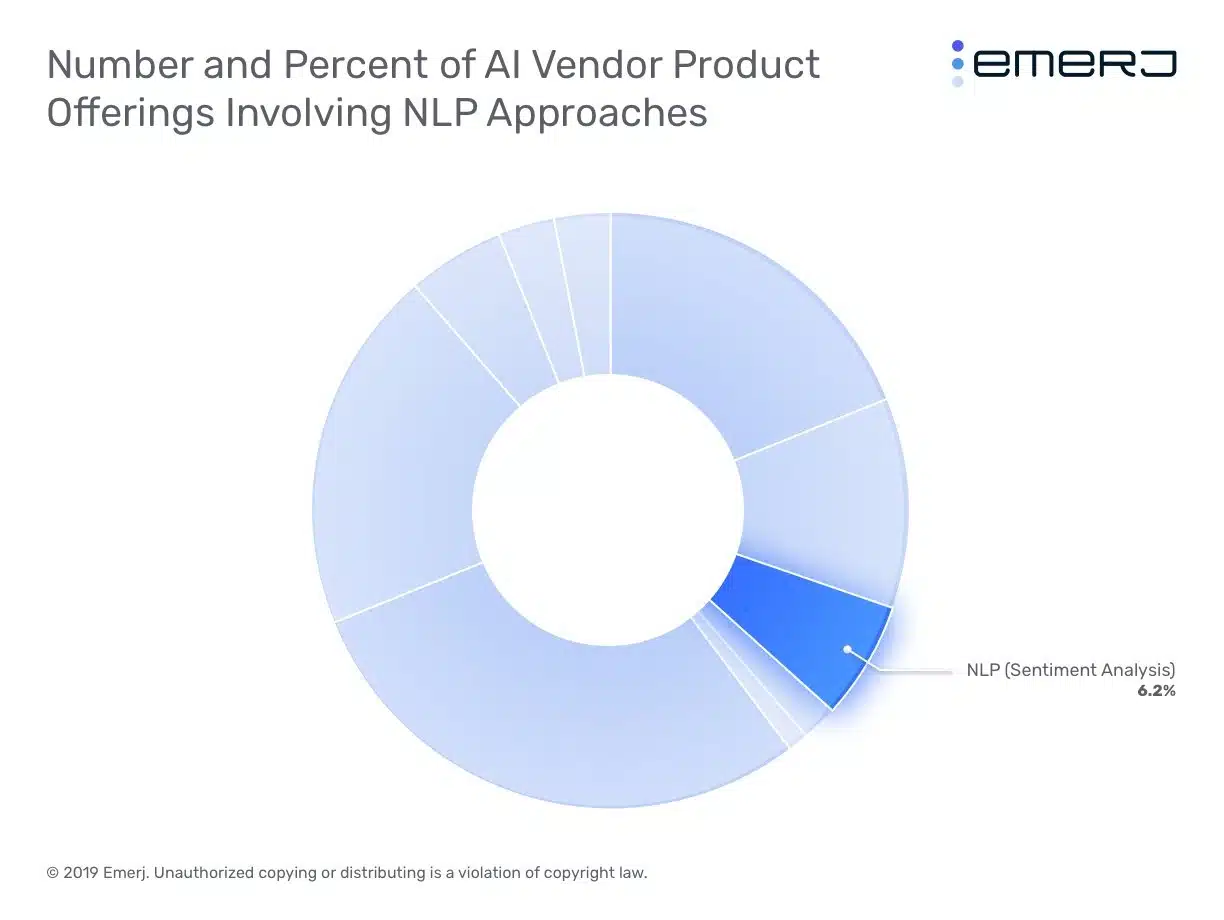
Our research shows sentiment analysis product offerings made up roughly 6% of the total number of AI approaches across AI vendor product offerings. We discuss this finding in the next section of this article.
Challenges for Banks in Sentiment Analysis Projects
Although AI-based sentiment analysis tools are now emerging in the banking sector, it seems likely that most such endeavors require a human in the loop to truly be useful. According to American Banker, Citi’s senior vice president of social media, Frank Eliason, was quoted saying:
To get the most out of social listening tools, I personally believe the best sentiment analysis requires human review on every post. Often the numbers are minuscule because the tool cannot accurately determine an author’s feelings. Typically, you see barely-negative-barely-positive readings with the remainder of the sentiment of the message unknown or neutral.
But as you dig into that you will see numbers drift dramatically when you use human intelligence. One company once offered sentiment analysis of banking, with certain banks clear winners. As I dug into it, the tool included topics not even related to the banks, very much skewing the results.
To understand how early-stage this technology is, we categorized all of the AI vendor product offerings for sentiment analysis from AI vendors selling into banking on the basis of what we call the Emerj Vendor Scores. Below are the scores and some aggregate data for sentiment analysis vendor product offerings and the vendors offering them:
We found only 6 AI vendors that both sold into banking and offered products for sentiment analysis, indicating that the technology is nascent in the sector. Moreover, sentiment analysis applications have very low Evidence of ROI and Evidence of Adoption Scores, which shows how few banks and financial institutions are actually buying sentiment analysis products, and if they are, how little evidence there is that those products are yielding them an ROI. These were some of the lowest scores among AI approaches. In addition, vendors offering sentiment analysis products account for less than 1% of the total venture funding across the AI in banking vendor landscape.
Banks and financial institutions looking to implement sentiment analysis projects need to understand that this is new even for vendors, and, as such, sentiment analysis applications may not be a great fit for a first-time AI project. We believe these projects might require a certain level of data competence that banks need to achieve before starting them in earnest.
We categorized the various sentiment analysis use-cases based on the types of data they tend to require:
- Understanding Customer Attitudes
- Equity Investing
- Monitoring Credit Markets
- Compliance Monitoring
In this article, we delve deeper into the various use-cases for sentiment analysis in banking and elaborate on real-world projects involving banks and AI vendor products.
In the section below, we list out some of the more common use-cases that currently exist for sentiment analysis in banking, starting with understanding customer attitudes.
Understanding Customer Attitudes
The importance of accurately understanding customer sentiments using was often brought up by some of the industry experts we spoke to during our research. This makes sense because this application is ripe for disruption with AI due to the large volumes of customer data involved which would take human employees far too long to accurately monitor.
Banks can use sentiment analysis AI software to read through open-ended text customer feedback forms, surveys, or social media posts about the banks from customers. The software can ingest vast volumes of such data and perform a number of tasks such as identifying if the overall sentiment is positive, negative or neutral, or pick out posts where a customer’s intent has been identified as positive.
By understanding how social media users comment on banks and financial institutions, banks can understand how to improve their customer acquisition and customer experiences. For example, if a competitor’s’ promotional campaign was receiving a strong positive sentiment, the bank might consider crafting their own campaigns in a similar way.
For example, the Bank of Italy claims to have engaged in an AI project to understand customer sentiments from twitter feeds. The bank input an AI algorithm with tweets about five European banks, BMPS, UCG, ISP, UBI and Deutsche Bank, to better understand how customers reacted to these banks. These reactions were then used to predict customer preferences and financial variables of the bank.
AI vendor products such as Expert System’s Cogito platform provide NLP-based sentiment analysis capabilities. Cogito could give banks the ability to gain insights about customer such as top customer issues from customer survey data. Additionally, banks might also gain the ability to merge all types of social data with other data streams to identify customer preferences and trends.
Equity Investing
While the concept of sentiment analysis has been used in financial trading in the past, natural language processing and machine learning are now starting to automate some of the tasks associated with trading and investing.
When dealing with research for financial trading, such AI software are input with research data from news media, social media, press releases and other sources.
For example, sentiment analysis can be used to augment the research process involved with equity investing divisions in banks. Researchers could use the software to look into far more companies because the algorithm could compile and prioritize the most relevant equity information for each company from multiple data sources.
In a real-world use-case, J.P. Morgan claims to have used NLP in a project to augment the research capabilities of their portfolio managers and research analysts in equity investing.
The bank worked with a team from APG Asset Management to extract data from European Central Bank statements in a pilot project to understand what was possible with sentiment analysis. The bank claim it developed an AI algorithm from almost 250,000 analyst reports and central bank statements to understand financial terms and jargon.
The bank then claims to have tested the software by feeding it with news articles that were focused on tracking equity markets. The software then generated equity investing insights from the data, such as identifying outlier companies that have launched new disruptive products. JP Morgan claims the equity investments they made that were based on the algorithm outperformed indices such as the NASDAQQ50.
Monitoring Credit Markets
Banks could also use sentiment analysis to monitor credit sentiments from news media. Press releases and other articles about credit markets contain a lot of information about the performance of credit securities provided by companies. Using NLP-based sentiment analysis, banks could potentially analyze news media related to credit markets such as those mentioning specific bonds or commercial papers issued by companies.
In a real-world business use-case, Citigroup claims to have launched the CitiVelocity, a tool that uses machine-readable news media data from Thomson Reuters. The bank claims they use the tool to research companies on the credit-default swap index in the U.S. and Europe.
The tool can give users a list of companies that being talked about most positively or most negatively. AI analytics engines can be input with this data to identify correlations between news events and the performance of securities in the credit markets. Generating these insights with AI software eventually helps cut down on the time and cost involved with the financial research process.
Compliance Monitoring
Just like with financial trading data, compliance divisions in banks have historically collected and stored records of compliance regulations and need to constantly update their processes to abide by these mandates. Not doing so could lead to potential fines or a loss of banking licenses. Thus, most large banks have existing budgets, IT teams and automation efforts in this space.
The automation software that banks use to scour regulatory websites for updates or changes are largely rules-based. AI-based sentiment analysis software might help banks manage these regulatory processes by scouring through a large volume of this information and classifying each update by how relevant it is or how high it ranks in terms of priority for action.
AI vendors like Compliance.ai provide AI software that could help manage regulatory changes and compliance risks. The company claims they use a human-in-the-loop AI training system to allow an algorithm to learn which updates are most relevant by using inputs from compliance experts at the bank. But, like many other companies in banking offering AI-based sentiment analysis products, such a use-case lacks robust ROi evidence.
Header image credit: Wells Fargo