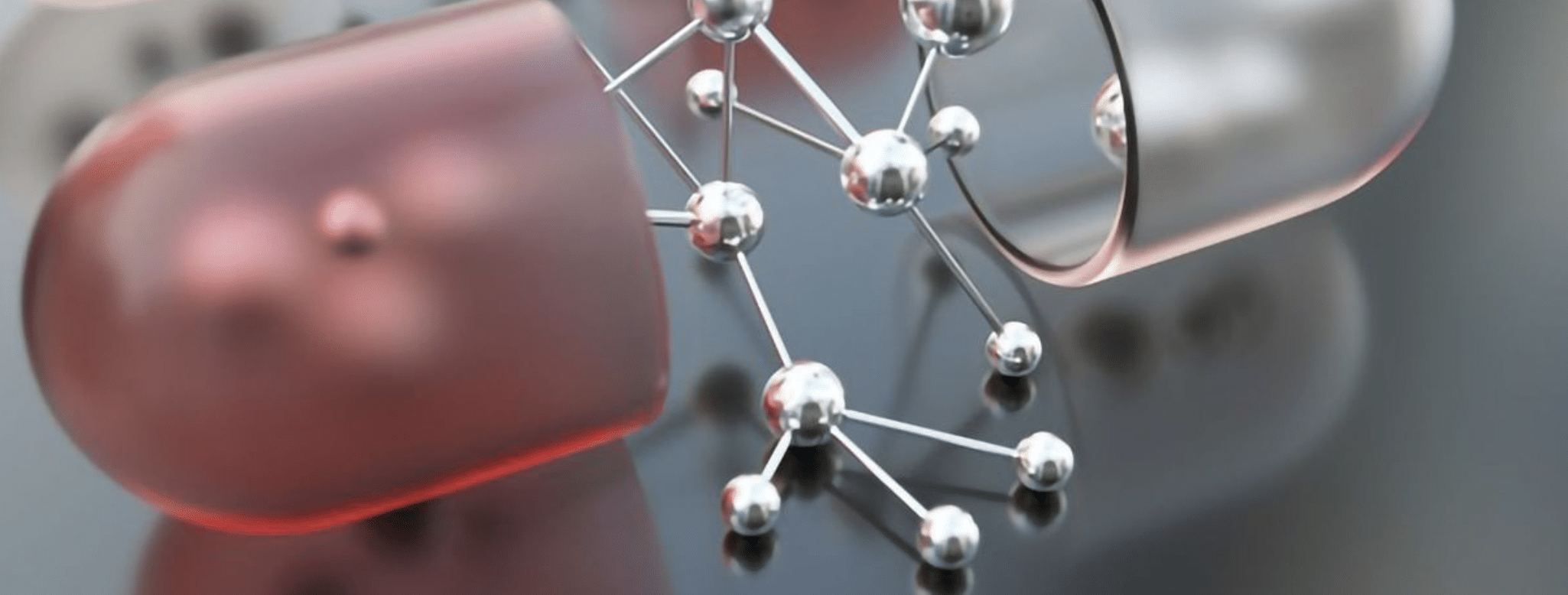
This article was originally written as part of a PDF report sponsored by expert.ai, and was written, edited and published in alignment with our transparent Emerj sponsored content guidelines. Learn more about our thought leadership and content creation services on our Emerj Media Services page.
We live in a world brimming with information. The digital universe is growing exponentially, year over year, and the data available to humans today far exceeds their ability to process and act on it before it becomes stale and irrelevant.
Data means everything to leaders and stakeholders in the life sciences industry. How do they navigate these vast stores of data and confront today’s health, economic and financial challenges?
“Many health researchers assert that 80% of scientific publications contain assumptions that must be verified and cross-checked within the document against real-world evidence,” says Christophe Aubry, Head of Sector Strategy for Life Sciences and healthcare at expert.ai, an NLP firm based in Italy.
“The knowledge included in this data can offer valuable insight for strategic activities like R&D, innovation, competitive intelligence, and adverse events monitoring,” Aubry continues. “Thus, an organization can establish a competitive advantage by making data more accessible and actionable. The quantity, variety and complexity of scientific content makes it difficult to find what you need when you need it. In this context, AI-based semantic applications have evolved from a game-changing service to a de-facto requirement for both competition and innovation.”
Unfortunately, researchers constantly struggle with information overload, and this challenge is only exacerbated by the nuance of industry-specific terminology. Because few people have the depth of knowledge necessary to understand and process medical concepts, scaling that knowledge is a nearly insurmountable task.
Can artificial intelligence (AI) help? It can…with the right technology and approach.
Traditional data mining tools leverage keywords to extract information and are incapable of reading text or understanding language like humans. As a result, they miss critical information that fails to match up perfectly with the user query.
This is particularly applicable to scientific content where the same term may indicate different concepts (e.g., CAT as gene vs. CAT as Computed Axial Tomography) or different terms may indicate the same concept (e.g., REGN-10933 and Casirivimab refer to the same drug).
AI technologies such as machine learning (ML) and natural language understanding (NLU) are truly capable of impacting the ecosystem of modern medicine. There are a number of reasons why:
- The push for personalized medicine
- The exploration of mRNA and gene therapies in creating new treatments
- The need to find patterns amid terabytes of data
- The pressure to make decisions and adopt solutions
“AI must leverage its understanding of language to classify and extract what matters from biomedical content, resulting in actionable insights for informed decision making,” says Aubry.
Whether the focus is on drug discovery, the design of clinical trials, or the tracking of adverse effects in the drug safety process, AI stands ready as a worthwhile partner to the industry’s subject matter experts.
Here is a glimpse into how AI technologies are helping life sciences companies scale key business processes, overcome operational challenges, and realize new opportunities.
Use-Case 1 – Evidence-Based Knowledge Discovery
Introduction
The drug discovery process, a key application of evidence-based knowledge discovery, costs an immense amount of time, effort, and resources. Even as a target passes through the successive phases of the drug discovery process—from hypothesis generation to clinical trials—there’s no guarantee that it will become a viable drug.
Today’s pharmaceutical companies have turned to AI-enhanced technologies in an effort to speed up the drug discovery process.
In addition to drug discovery, drug repurposing has emerged as a key area that promises both savings and new treatments, on a potentially faster timeline. Because these drugs have already reached the market, how they work and their mechanism of action is already known. As a result, applying AI to researching these existing drugs can save valuable time and expand their reach.
Indeed, the drug discovery and drug repurposing processes have long awaited the capabilities promised by AI and its innovative capabilities.
Actions Taken
To help researchers identify key data amidst massive amounts of scientific literature as well as scale the drug discovery and drug repurposing processes, expert.ai provided clients with its patented technology that enables accurate identification and connection of biomedical information such as diseases, drugs, treatments, symptoms, genes, proteins, and other data elements from content—but at rates that far exceeded human capabilities.
In building the logic, expert.ai designed a world-class knowledge graph specialized for life sciences and healthcare. The knowledge graph allows for data standardization and linking, such as grouping conditions into families of diseases or identifying mechanisms of action and drug classes. Preeti Chawla, Senior Clinical Data Analyst at expert.ai:
“There has been immense knowledge available from scientific publications to understand how genes could possibly contribute to a disease. The scientific community is facing a lot of challenges in mining such data sets to associate genetic markers with particular diseases and to develop treatment strategies.”
Expert.ai’s solution helps researchers identify new data elements to enrich the knowledge graph and helps to keep the graph consistent and current, as changes happen frequently in various therapeutic areas. It also helps researchers monitor multiple data sources (from publications to real-world evidence through clinical data) at rates that far exceed human capabilities.
Results
Between years of experience in the life sciences industry and collaboration with subject matter experts, expert.ai specialized its innovative AI technology to help researchers scale the drug discovery process. This technology is fueled by a unique knowledge graph that contains more than 2 million concepts and 6 million relations across 12 different languages. Within that, you can find more than:
- 100,000 diseases
- 450,000 drugs and chemicals
- 115,000 symptoms or findings
- 86,000 genes and proteins
- 65,000 geographic locations
The depth and breadth of the knowledge graph enables extraordinary precision, coverage and granularity when categorizing documents, extracting meaningful data, and connecting information in scientific content or medical notes across any therapeutic area.
With scientific literature as the primary source of data on target association with disease, expert.ai mines publications daily and structures drugs, diseases, targets or biomarkers to create a knowledge synthesis centered on a disease area. This allows for quick insights to discover causal relationships between targets and diseases, potential treatments and risk factors.
Expert.ai technology brings human-like comprehension of language to the drug discovery process by enabling researchers to speed up their research and data analysis. Additionally, it also supports the identification of potential new applications for existing drugs, in an effort to repurpose treatments in other disease areas.
Use-Case 2 – Clinical Trial Design
Introduction
Drug development is a long and expensive process. It takes on average 10–15 years and USD $1.5–2.0 billion to bring a new drug to market. Approximately half of this time and investment is consumed during the clinical trial phases of the drug development cycle (reference: https://doi.org/10.1016/j.tips.2019.05.005).
Given recent events (e.g., COVID-19), it has become imperative that the development timeline is shortened for new treatments. As the world raced to develop a vaccine that could win the war against the pandemic, we saw a surge in the number of clinical trials being conducted as experimental treatments started to emerge. The clinical landscape of who was doing what, where, and when rapidly evolved. By monitoring clinical trial data and learning from previous outcomes (i.e., what went well, what went wrong), researchers can, in turn, design better clinical trials.
What was emphasized during the COVID-19 outbreak is an ongoing need that spans numerous therapeutic areas and rare diseases. Creating a clinical landscape for drug development requires capturing, mining, and linking clinical trials around the world, extracting key data points from semi-structured to unstructured data and presenting them in an easy-to-use manner for informed decision making.
“Clinical trials allow us to tap into the potential of AI throughout the drug development life cycle, starting with design and planning, identifying principal investigators & site locations, enrolling patients, and monitoring adverse events,” says Archna Bhandari, Executive VP, Data and Analytics, at expert.ai.
Actions Taken
Expert.ai’s technology mines data from more than 700,000 clinical trials worldwide. This includes clinical trial registries such as clinicaltrials.gov, EUDRA, EUPAS, Japanese registries, Australian registries, and others. Their AI platform takes care of data mapping, deduplication, and linking across registries to make it easy for researchers to consume data.
By incorporating cutting-edge NLU and ML technologies combined with standard and custom taxonomies, expert.ai can understand and link important terms like “coronavirus,” “COVID-19,” and “SARS-CoV-2” so that researchers can identify and target the data most likely to help them accelerate the design and development of their clinical trials.
One of the important criteria for clinical trial design and success is related to patient recruitment. Such information is described under the eligibility criteria of the trial, which is detailed in an unstructured data field.
Inclusion criteria lists the key features that the targeted patient population must qualify for, such as:
- Demographics
- Clinical characteristics
- Duration of disease
- Severity of disease
Exclusion criteria list the additional key features that could interfere with the study or increase the risk for an unfavorable outcome or adverse events. For instance, the presence of comorbidities must be avoided for a patient to qualify for a recruitment in clinical trials.
Expert.ai technology makes it possible to convert this unstructured data to structured information and create key attributes for a patient profile. These patient profiles can then be used as a screening tool to identify patient populations from real-world data such as Electronic Health Records (EHRs). In addition, this analysis is further expanded from one single trial to a set of related trials, thus allowing the use of this information for cohort design for new trials.
Expert.ai technology also facilitates retrospective analysis for new trial design by tracking data points such as change in enrollment numbers and time taken in moving from one recruitment status to another during the length of the clinical study.
Results
Expert.ai technology allows centralized access to all clinical trials around the world. It provides the most up-to-date and comprehensive data landscape by disease, drug, mechanisms of action, organization, or geography. Not only can researchers find related trials, they can also access related publications, news, study results, and principal investigators all in one place.
Thanks to deep natural language understanding capabilities, key data elements are accurately identified from trials and standardized to offer data analytics capabilities that go way beyond keyword search. Reporting features include drill-down and filtering that help researchers find site-level information, research facilities, lead researchers, and networks of collaborators.