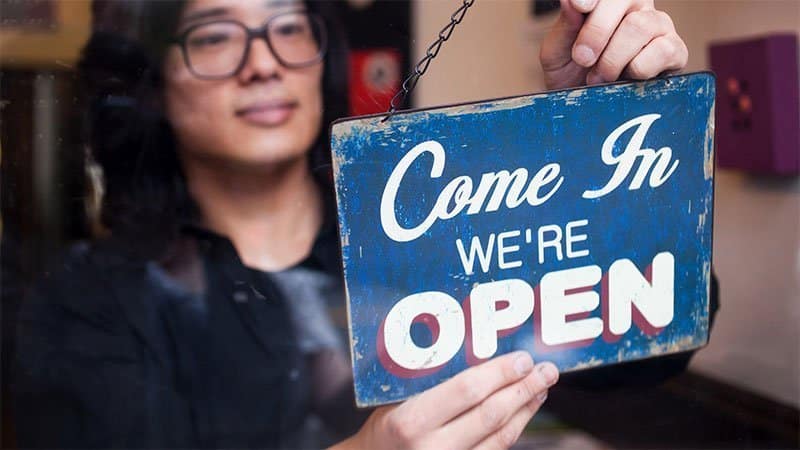
Most AI solutions are not for small businesses, but they will be over time. I decided to put together an understanding of where AI solutions are now and how they will eventually become accessible to small businesses.
This short guide discusses the levels of AI investment and expertise that companies will require to implement AI in certain ways and the challenges that artificial intelligence vendors need to overcome to make themselves more usable for and accessible to small businesses.
it’s important for small business owners not to be mired and distracted by the hype of AI, but to understand it for what it is and to only pay attention to the kinds of AI solutions that might make a difference in their businesses. This report seeks to provide small business owners an understanding of where machine learning fits into the overall technology spectrum of small business, allowing them to protect against the AI hype and make more fruitful use of AI when it does become accessible in their small business domains.
In this report, I discuss the following:
- The levels of investment and expertise necessary for implementing AI
- Two problems AI vendors need to solve for AI to be accessible to small businesses
- Hype traps small businesses need to avoid when looking at machine learning solutions
The Levels of Investment and Expertise Necessary for Implementing AI
There are three levels of investment and expertise that determine which businesses are able to access AI solutions. They are as follows:
- Creating an AI solution
- Working with an AI vendor company
- Using an AI tool
Creating an AI Solution
A business that wishes to create its own AI solution in order to solve its specific business problems will require the largest amount of investment and the highest level of AI expertise. More specifically, these businesses will need:
- Large volumes of data
- Robust data infrastructures and a data cleaning process
- AI expertise on their leadership teams
- Deep domain expertise and the role of AI in that domain
- Venture money
Familiar AI-based recommendation engines such as Netflix and Amazon can predict which recommendations will drive a particular user to do a desirable action, such as watch more movies and buy more products. This is because these companies have a large volume of data from which to draw. This is not the case for small businesses with 20 transactions a day.
Additionally, these companies employ large teams of data scientists to structure, clean, and update their databases and AI solutions, something small businesses simply can’t afford unless they’re backed by venture capitalist money that can support businesses as they build their AI solution before it hits the market.
Working With an AI Vendor Company
Although businesses looking to integrate AI solutions into their workflows could pay a vendor company to do it, they will likely need to hit the same prerequisites as companies looking to build their own AI solutions.
In some cases, it can take months of integration time to implement AI software, even with some data science talent onboard, and the software still may not provide the expected results. The difficulties of setting up and integrating AI solutions can largely undermine any benefits of the software unless a business already has the right AI talent onboard that would allow the integration to go smoothly.
Customer retention marketing company Optimove might be able to work with a company like 1-800-Flowers.com because it likely has the data science talent that a local lawn care company would not. The same goes for companies like Digital Genius, Luminoso, and Acquisio. They would love to work with mom-and-pop outlets if they could, but if they don’t already have the requisite data science talent onboard to set up and integrate the AI software into their existing systems, then those mom-and-pop outlets aren’t ready for AI.
In most cases, the best way to find out if a vendor has a genuine small business solution is to check the client or case study page of their website. If all the companies mentioned on the website have 200 or more employees, it is safe to say the vendor does not offer a software that a small business would be able to implement. For example, Acquisio offers Promote for Small Businesses for lead generation and PPC campaigns, but it does not mention any small businesses in its Success Stories pages.
Using an AI Tool
These robust prerequisites are difficult for small businesses to meet, and so the fact is that for a small business to implement an AI solution, that solution has to genuinely work “out of the box.” This means that it would be ready to use without any training, talent, or tweaking. Anyone would be able to learn to use it in a short time simply by going through a couple of online tutorials or reading the manual.
I refer to these solutions as “tools.” AI tools don’t require in-house data science talent, data infrastructure, venture money—none of that. Unfortunately, AI tools are a very small fraction of the AI business solution space, but they do exist.
One example is Google Maps, which businesses of all sizes can implement togood effect. Facebook Ads is equally simple to use. Both use machine learning APIs, but neither requires a data scientist to use and maintain. It’s really when something is consumer friendly that it’s accessible to small businesses.
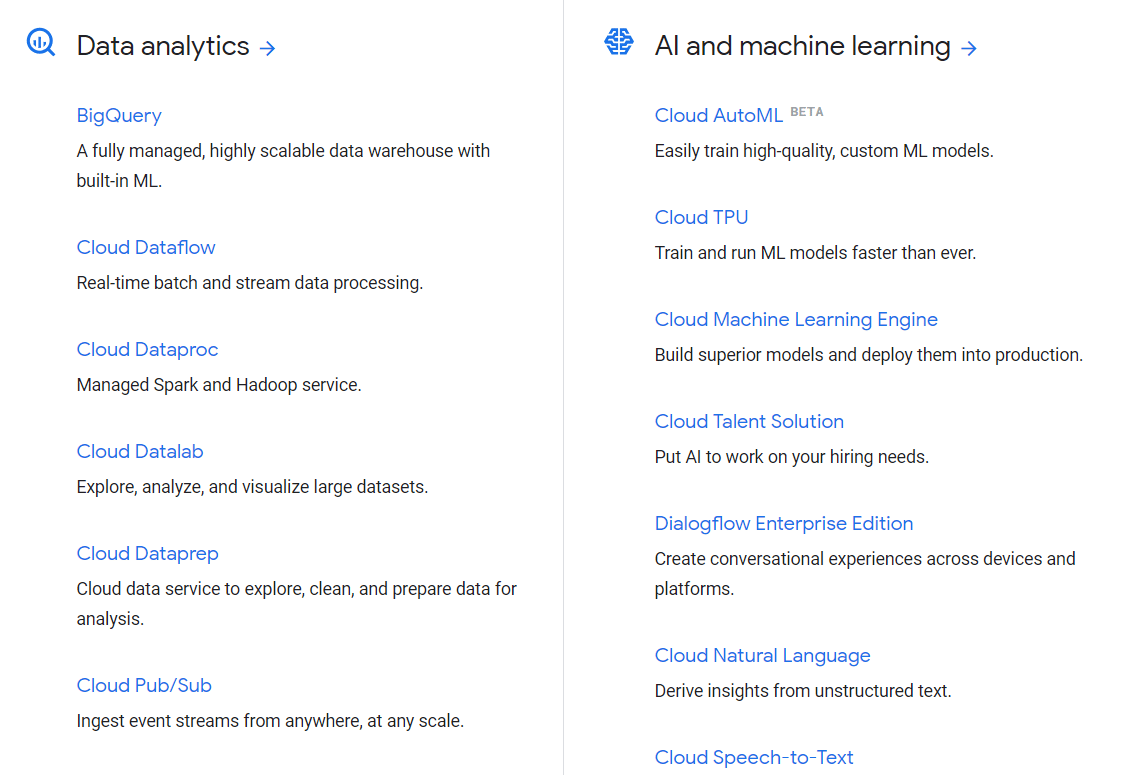
Two Problems AI Vendors Need to Solve for AI to be Accessible to Small Businesses
AI vendors would all like to tap into the small business market. As a result, they continuously work on coming up with solutions similar to Google Maps and Facebook Ads, which are highly accessible to small businesses. Amazon is also quite good at helping small businesses market their products through its recommendation engine.
However, none of these solutions became accessible overnight. Facebook, for example, did not manage to come up with a consumer-friendly advertising solution on day one. It took many years of intense effort and a large budget to get it to where it is today.
Companies such as Optimove would love to be able to give a five-person eCommerce company actionable, realistic, and legitimate recommendations for their PPC campaigns. Unfortunately, that is not possible right now because of 2 big problems:
- The data problem
- The UI problem
The Data Problem
Small business do not have large records of data, and this will continue to be a problem until a business grows to a point where they have enough data. This is even more of a problem for offline businesses because their data is likely not digitized to the capacity it would need to be for an AI solution to drive value for them.
On a website, for example, a business can hypothetically track each click and mouse movement without much trouble, and this would provide the business with key data points. It is not the same case for physical stores, where digitally tracking the movement of customers from aisle to aisle and product to product is much harder to capture.
The only solution for the data problem of small businesses is aggregation. An AI vendor can theoretically gather enough data from thousands of its small business software users selling 20 products a day and then train a machine to perform at a similar level as Amazon, for example. It can aggregate the data in order to train its recommendation engine software, which could then provide the same quality of recommendations to any small business and help them sell more products.
However, in order to do that, thousands of small businesses have to purchase the software in the first place. A startup AI vendor with venture money may conceivably put out software in beta form to gather these data points, but that will take a long time. In order to even get to that level of aggregation, the software has to become reliably high ROI for big enterprise.
Another problem with data aggregation is that it may not work in some sectors. The value of data aggregation stems from commonalities. In order for a machine learning software to identify patterns, the data needs to have essential points in common. For example, if a vendor can aggregate data on 1,000 small businesses selling 20 t-shirts a day, it can discern patterns of behavior that trigger more sales.
For niche products or businesses, data aggregation becomes problematic. A company that makes and sells customized golf memorabilia out of pewter is probably going to be only one of a handful of similar businesses. It may not be possible to get enough data together to train an AI system to be of much use for that business, no matter how many e-commerce websites plug into it.
It is also important to note that not all data will have value in machine learning. Just as data has to have some level of commonality, it also has to be “clean.” Clean data is relevant and recent information that has a uniform or consistent structure. This allows the machine learning software to learn from it. The data also has to relate to the rapidly changing trends and nuances of the business or else it will produce no usable results. In other words, clean data is better than big data.
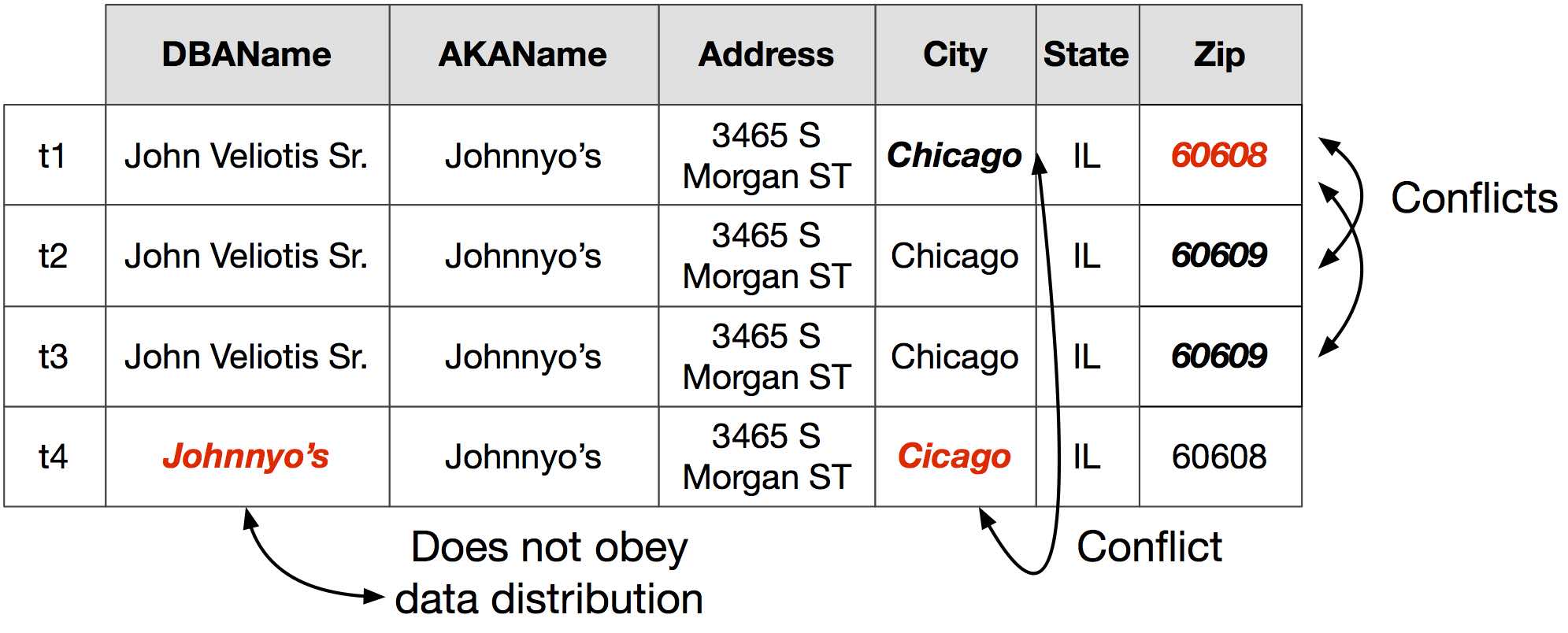
At some point, AI developers will come up with better algorithms that can train on less data. However, it is unlikely the scalability will get to a point where it can help the stay-at-home mom baking cakes for sale. The most likely solution for all these data problems is a combination of data aggregation and algorithms that can train on less data.
The UI Problem
The second hurdle in making AI solutions accessible to small businesses is the user interface of the software or UI. AI software UIs today are very difficult for someone who isn’t a data scientist to understand. They are not user-friendly, which is why a business would need a data scientist to set up, integrate, and manage the software as mentioned earlier.
In all likelihood, this will not be a problem in the future. Companies will find a way to make it easier for people to interface with AI software, but it may take many years.
It will not be the first time that new software evolved to become more user-friendly. One example is MailChimp, popular marketing automation software launched in 2001. It is relatively simple software compared to something like Marketo, but back when it first came out, it took someone with technical knowledge to use it.
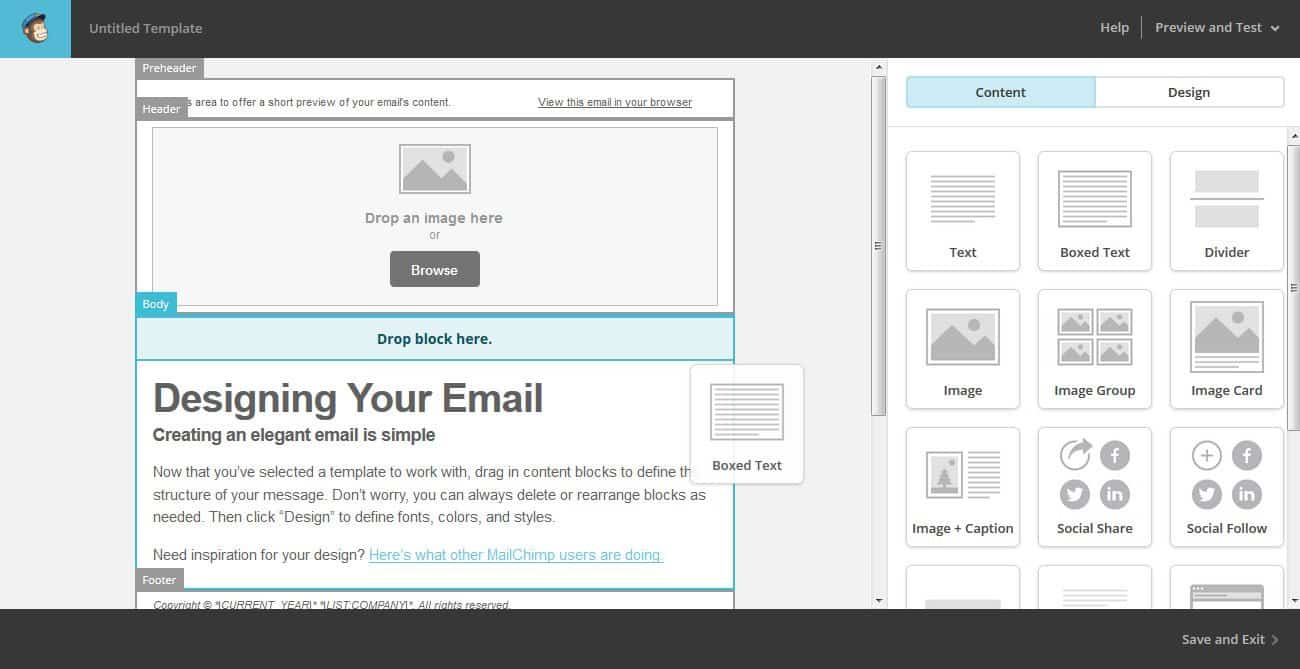
Over time, people figured out ways through trial and error to hide the complicated processes behind the scenes so users could simply drag-and-drop email or click a button to build automated campaigns without having to know a line of code. Nowadays, people without a tech background can feel their way through automation systems with a couple of tutorial videos, but it took more than 15 years to get to that point.
Hype Traps Small Businesses Need to Avoid When Looking at Machine Learning Solutions
Chatbots
Chatbots are an application of machine learning that seem to be getting a lot of traction across industries. The healthcare industry, for one, is an early adopter of the technology. However, chatbots still have the same restrictions for small businesses as other types of AI iterations. Chatbots still need a certain amount of clean data and a certain set of skills to train and use them. An out-of-the-box chatbot that provides real value is simply not available.
It is important to note that chatbots are only practical when used within well-defined and narrow limits. Use cases show the value of understanding the limitations of chatbots, especially for small businesses. They are most likely to provide robust ROI when used less aggressively.
For example, a business with many incoming queries could use a chatbot to respond to the first two tiers of questions before routing to the right human. Even then, it may not provide strong ROI for smaller companies. For the most part, a lot of chatbots are toy applications.
Toy applications are those built specifically to cater to people that want AI for its own sake and are for the most part a trap. Many companies adopt AI for purposes other than to solve a business problem. Fashion brands adopt chatbots because they saw a press release about their competitors using one, and so they feel they should have one, too.
Vendors are more than willing to encourage this type of thinking. However, evidence of really robust and strong positive ROI with chatbots is not something that is ubiquitous right now.
When I interviewed Conversica’s Chief Scientist, Sid J Reddy, he said:
For a company to be successful in building conversational systems, they need to be really good at doing natural language processing and machine learning…We don’t have a model that understands everything for every context…a particular model can only do well based on the examples it has. So for that reason, different companies focus on different intents and entities.
That said, the concept of a chatbot is still powerfully attractive. A fully-automated service would be ideal for both businesses and customers. However, it would take a lot of money, time, and effort on the part of the business to implement it at the present state of chatbot development. It is simply more trouble than it is worth for most small businesses.
Marketing Language
Vendors will often market themselves as offering machine learning solutions, when in fact they are only capitalizing on the AI hype. I previously discussed three rules of thumb for cutting through the AI hype in one of my favorite pieces on this site. Marketing language often includes buzzwords for their own sake, touting the company’s solution as if it’s going to magically solve a business’ problems as if it were an AI tool.
As previously stated, most AI solutions are not tools, and vendors tend not to work with companies that do not have large volumes of clean data and in-house data scientists to run through the integration process.
Takeaways For Small Business Owners
AI vendors would love to convince small businesses they can integrate AI solutions into their workflows, especially when small businesses are an enormous market, but unfortunately, AI solutions are not viable for small businesses in 2018.
This is because small businesses have several disadvantages when it comes to implementing AI. For example, if a business of any size doesn’t already have a room of capable data scientists, many AI solutions are not going to be reasonable investments for that business. AI software requires a firm understanding of the science and a large sum of venture money behind it because it’s not often going to deliver results to businesses on day one of its implementation.
Some enterprises are only recently starting to hire AI and data science talent so they can make use of AI to solve their business problems, and small business likely won’t be able to do this.
Small businesses make up a huge part of the market, which makes them an attractive target for AI vendor companies. However, most artificial intelligence and machine learning systems are still too complex and expensive to be accessible to small businesses.
The only way that AI will become readily accessible to small business owners is to commoditize it to such an extent that it becomes consumer-friendly. To make that happen, it has to start from the top. Big companies have to find an AI solution profitable so they will invest in it, and in turn, vendors can continue to develop that solution to the point that it becomes a something that anyone can use. That is how machine learning will become accessible to small businesses.
Header Image Credit: JPMorgan Chase