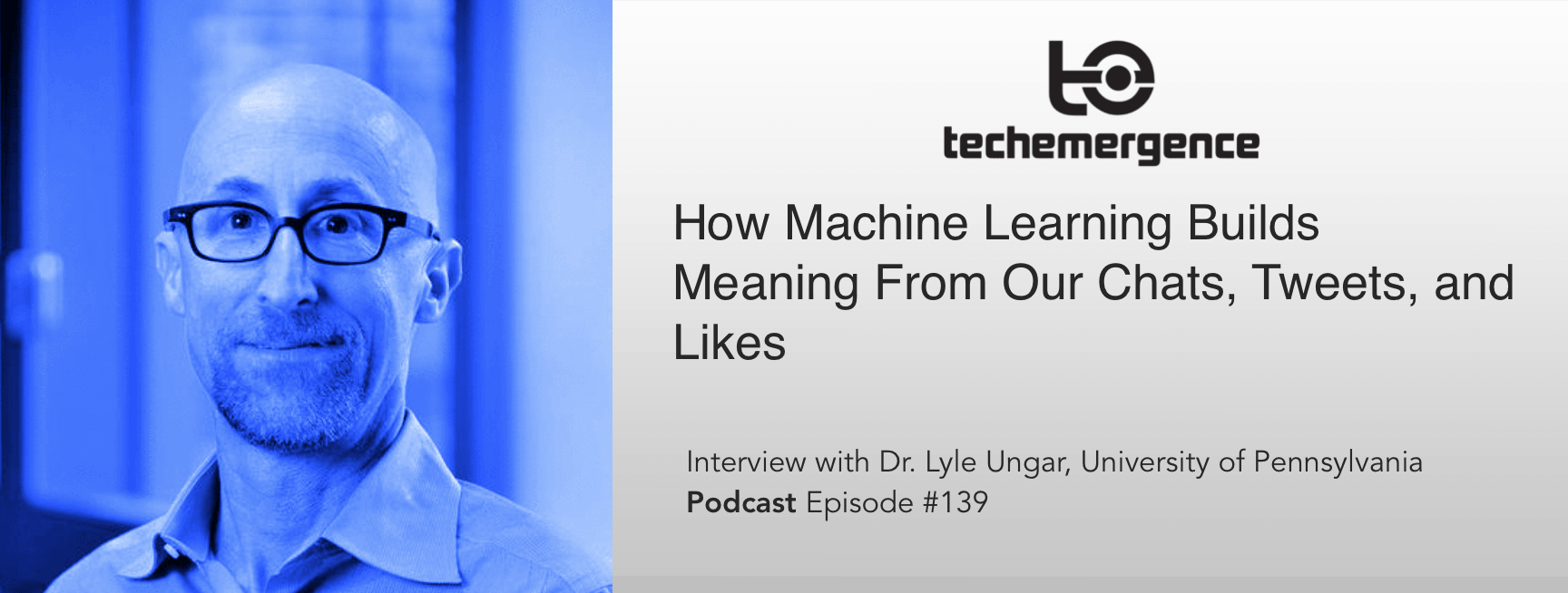
Episode Summary: There’s a small lab in Pennsylvania that may know your gender, age, and understands facets about your personality, whether you’re introverted or extroverted, for example…and it’s using machine learning to help make conclusions from social media information. For those who are raising an eyebrow, know that they’re not tapping into people’s accounts without permission. The described study is happening at University of Pennsylvania and is led in part by Dr. Lyle Ungar. In this episode, we talk about the focus of his work – on finding patterns between users and their language on social media content, and building an understanding for how this information might help individuals and communities in the future.
Guest: Dr. Lyle Ungar
Expertise: Computer and Information Science; Bioengineering; Operations and Information Management; Psychology
Recognition in Brief: Lyle Ungar is a Professor of Computer and Information Science at the University of Pennsylvania. He received a B.S. from Stanford University and a Ph.D. from MIT. Dr. Ungar directed Penn’s Executive Masters of Technology Management (EMTM) Program for a decade, and served as Associate Director of the Penn Center for BioInformatics (PCBI). He has published over 200 articles and holds eleven patents. His current research focuses on statistical natural language processing, spectral methods, and the use of social media to understand the psychology of individuals and communities.
While a student at Sloan, Dr. Ungar worked as a strategic business analyst at the Boston Consulting Group. Since coming to Penn in 1984, he has consulted for companies ranging from start-ups to Fortune 500 companies on strategic use of information technology in areas including data mining, information retrieval, online auction design, expert systems, and e-commerce.
Current Affiliations: University of Pennsylvania; Distinguished Research Fellow at Annenberg Public Policy Center
Our Social Media Language Becomes Us
The words that people use on social media can tell a lot about who they are in terms of personality, interests, and state of being. A group at University of Pennsylvania, co-led by Dr. Lyle Ungar, is pioneering a study to explore the associations between word use, personality, and well being. So far, the group has accumulated data from 70,000+ people across social media platforms, and correlated people’s language use with categories of personas – for example, “young, agreeable female” or “older, neurotic man”. “We look at the words that are used in these cases, try to profile people, and get a flavor of who they are,” says Ungar.
Aside from Facebook, which is more personal and often requires permission to access full data, the study group has also gathered thousands of tweets from Twitter, a platform that’s easier to access and allows scientists to map where tweets and tweeters originate and form geographical patterns. This type of breadth has been useful in building entire personality profiles of whole communities.
Why does creating individual and community profiles based on social media language matter? The group is still exploring the the answers and consequences of this question, but one direction is how these machine-generated (in part) profiles correlate with other statistical data, like health and well being. Ungar and his team have been able to get 2,000 people to grant permission to their Facebook profiles, as well as share access to their whole health record. Why would anyone give away so much information? The data is kept private, for use only in the study, but Lyle believes that it goes towards a meaningful purpose.
“We can now look at language and personalities associated with certain diseases, some things are relatively easy, not surprisingly the words show a lot if they’re depressed or not depressed, other things are less obvious, like what sorts of words show up with anemia or diabetes.”
Out of this gathered data, demographic patterns start to emerge; not just the typical groups based on ethnicity and class, but also more subtle demographic groups.
“We’re looking at…who are the people talking more about tattoos, who are the people talking more about kids, who are the people talking more about partying, these all correlate with lots of positive things in life, like how strong your relationships are, and some negative things in life, like how likely you are to do drugs.”
These are just examples of possible correlations, but they provide a general gist of the types of insights that this data can potentially provide.
One of the most interesting correlations that they’ve come across, says Lyle, find patterns between particular counties and correlations with heart disease, and investigating how language varies.
“What you find is first the obvious things, there’s a correlation that more black people tend to die of heart disease, more old people of course, more males, but once you control for all of that, and smoking and diabetes, what you find is that cities and counties with more angry tweets have more people dying of heart disease.”
What’s surprising, says Lyle, is that many of these “angry” cities have younger populations, with most people in their thirties.
There are various factors that feed into cause and effect, of course, but at the individual level, the data shows patterns of men who use more angry language as being more likely to have heart attacks, and at the county level, those communities tweeting about being bored and tired, for example, have more heart disease than those communities that are more excited about local events and are engaged with people within the community. Again, there is no causality in correlations, but finding such patterns gives scientists the opportunity to generate hypotheses and create studies that explore these potential relationships in more depth.
Machines May Hold Up Mirrors, Trigger Solutions
Where exactly does machine learning play into this process? The data collection and computing part is fairly simple, says Lyle; the hard part is gathering millions of tweets and extracting “the words,” which includes symbols like emoticons, and then assigning meaning to them. Individual words have different variations (I need a paper towel versus I need you, for example).
In many cases, you also need sets of words that make some sense (for example, “hot dog” does not compute without both words in context). In other words, in many cases you need to have clusters of words that machines can process. Once you ground the data down and feed it to the machine, look at the 3,000 most populated counties and the most populated words in those counties, you actually end up with a fairly small data set, explains Ungar.
“We have a whole bunch of people at Mechanical Turk, we give them some tweets, and say is this optimistic or pessimistic, is this an example of a good relationship or a bad, is this an example of someone feeling accomplishment or failure, we have many sets of ten thousands of tweets being labeled by humans, then with classic machine we use statistics, and say which words are predictive of optimism or not.”
The group has also experimented with using machine vision, which has made great advances, to identify posted images and profile pictures, adding to the data set that pieces together individual profiles. For example, machines can look for whether or not a person is smiling, identify the color spectrum and associated meanings used in the background, pinpoint if there are other people in the photo, or discern if it’s an actual photo or just an avatar. All of these identified elements help paint a more detailed and meaningful portrait of an individual and of collective communities.
How might correlative hypotheses drawn from artificially-created language profiles be helpful There are two potential levels, says Ungar. At the individual level, there exists the opportunity to feed into the emerging “quantified self” trend i.e. gleaning data from our habits over time in order to improve and more conscientious our actions. “People may opt in to say, I want the words I’m producing monitored to see if I’m turning certain people off,” says Ungar.
There are also an array of prevention and intervention possibilities that might be realized with such an approach. Medical practitioners and/or individuals themselves may be able to opt in for alerts when certain language patterns or words that arise in their comments or other social media communications correspond to certain diseases, such as pre- or postpartum depression in pregnant women.
Tracking language may also help us tune into routine habits, such as alerting us as to when we’re more likely to veer off a diet. When it comes to making real change, says Ungar, “people think about wearing a watch that measures heart beat, but it’s often less informative than the language we’re receiving and sending.” Indeed, a growing number of studies show that language does shape our perception of reality, and in turn our behaviors.
A more scaled outcome could deal with patterns of language at the macro or community level. For example, Ungar would like to see more politicians expand from a narrow interest in what affect their decisions have on the long-term economy to a real desire to know how their decisions and policies effect the welfare of the people – the quality of their relationships, for example, or whether people feel they have a purpose in life, patterns of which often emerge in language.
While some might understandably balk at the idea of having their every word monitored and tracked, the idea of using language patterns to help us make better decisions and lead more purposeful lives is a discussion worth pondering.
Image credit: Twitter