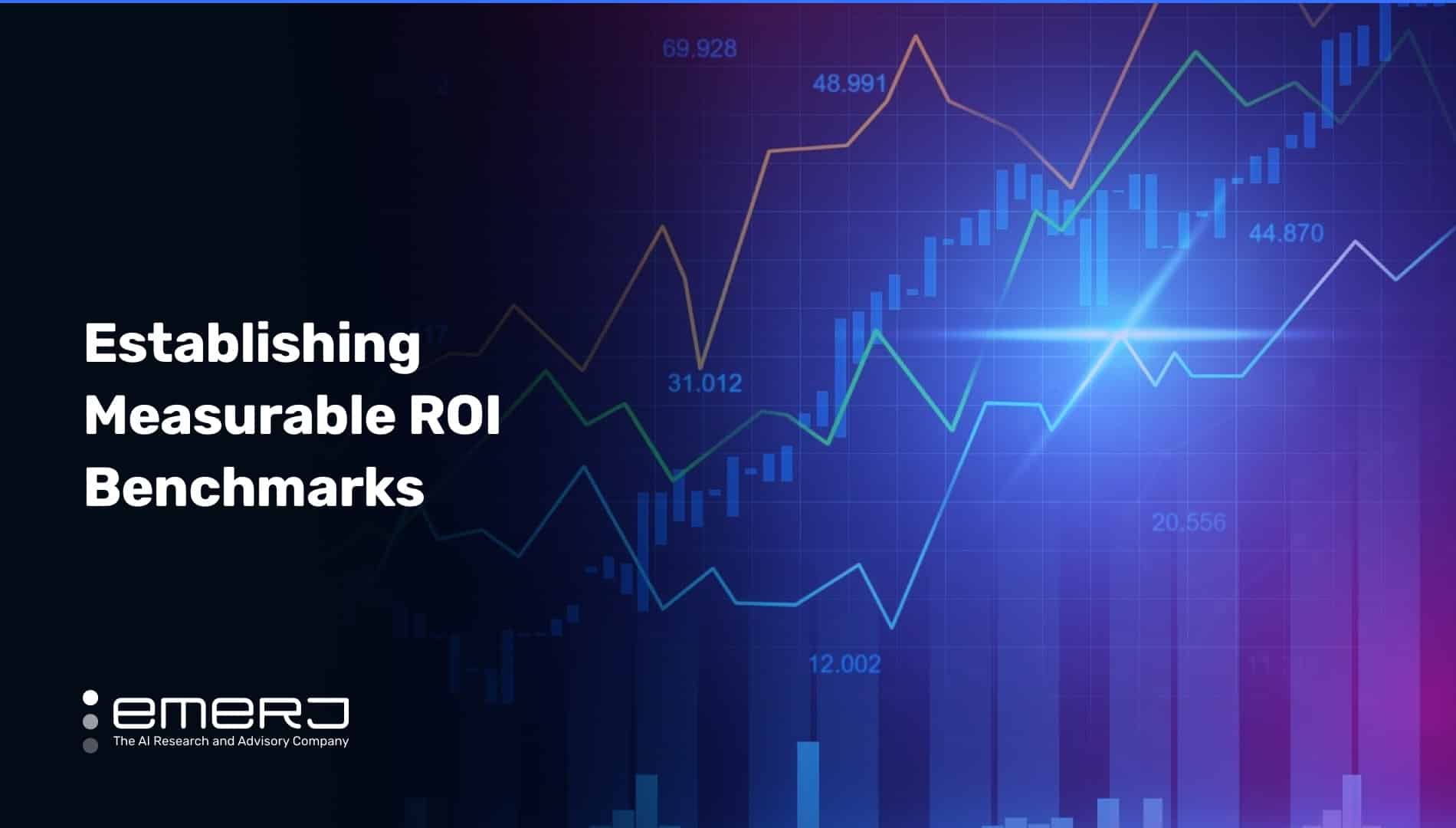
This article was a request from one of our Catalyst members. The Catalyst Advisory Program is an application-only coaching program for AI consultants and service providers. The program involves one-to-one advisory, weekly group Q-and-A with other Catalyst members, and a series of proprietary resources and frameworks to land more AI business, and deliver more value with AI projects. Learn more at emerj.com/plus.
Setting the right expectations for a measurable return on investment (ROI) is crucail for AI project leaders.
Don’t promise enough of the kind of ROI your decision-maker wants (almost always measurable or financial ROI), and the project won’t be approved.
Overpromise, and you set yourself up to almost certainly disappoint your client, and ensure that your AI project doesn’t get the resources or support it needs to turn into a deployment.
In this article, we’ll walk through a process for setting expectations about the measurable ROI of AI projects the right way, in five steps. This is an important part of the process for selling AI services that we train our Catalyst members on, and it’s something that any AI project leader should know.
The basic dynamic at play is simple:
- You need to promise enough measurable ROI benefit for the decision-maker to sign off on the project, and
- You need to deliver enough value in the eyes of the decision-maker for them to support the project – despite inevitable challenges – and see it through to deployment or expansion
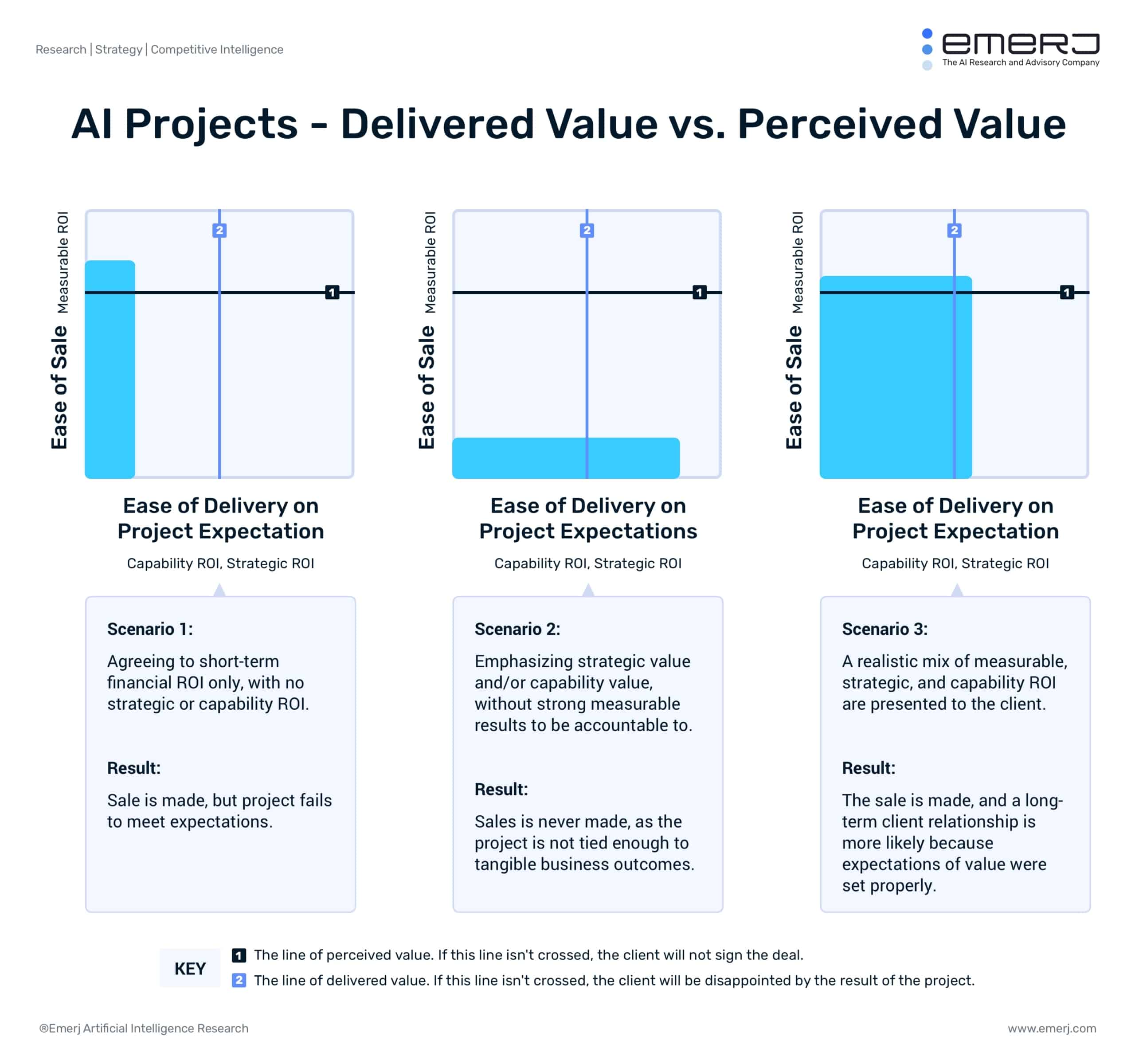
As an in-house AI project leader, this is the difference between being seen as a real AI change-maker who leads the firm to success, and someone with egg on their face. As a vendor, this is the difference between making slim margins on half-completed sandbox projects for disappointed clients, and establishing lucrative long-term transformation relationships with clients you can really deliver value for.
Setting expectations is absolutely critical.
Misinformed or disingenuous AI project leaders do the following:
- They accept whatever measurable ROI benchmark the client’s begins the conversation with, even if the client’s objectives are unreasonable, or the client’s benchmarks aren’t useful
- They ignore all talk the strategic relevance of the AI project, and allow the client to bend the entire conversation around turn-key, near-term measurable results
- They neglect to mention anything about AI maturity, even if the project will require significant transformation to infrastructure, teams, and company culture
Smart, experienced AI project leaders do the following:
- They listen to a client’s measurable ROI goals and supposed benchmarks, and use those as a jump-off point for exploration and conversation about what’s best for the project
- They always marry conversations about measurable AI ROI to the strategic relevance of the AI project (read our Emerj Plus guide Lead with Strategy)
- They frame the building of AI maturity (cleaning data, changing data infrastructure, training teams, hiring AI talent) as a benefit, not merely as a challenge – and they address maturity frankly and confidently in the best interest of the client
These dynamics apply to both in-house AI project leaders, and external AI vendors. If you’re a an internal project leader, you have to have this conversation with leadership. If you’re a vendor, this conversation happens with your prospect or your champion.
It’s not unusual for a decision-maker or prospect to genuinely believe that an AI project can reduce call center costs by 10% in three months, or entirely automate chat support in six months, or any number of other entirely unrealistic goals.
What we want to do is to organize all of the possible ideas for measurable ROI, and we want to bring the ones that genuinely make the most sense in terms of value to the client and what we can realistically deliver to the table (this requires cross-functional brainstorming, as we’ll address below).
Instead of agreeing to bend our AI project around unrealistic ROI measurements, an informed measurable AI ROI process begins with what matters most: The client’s objectives.
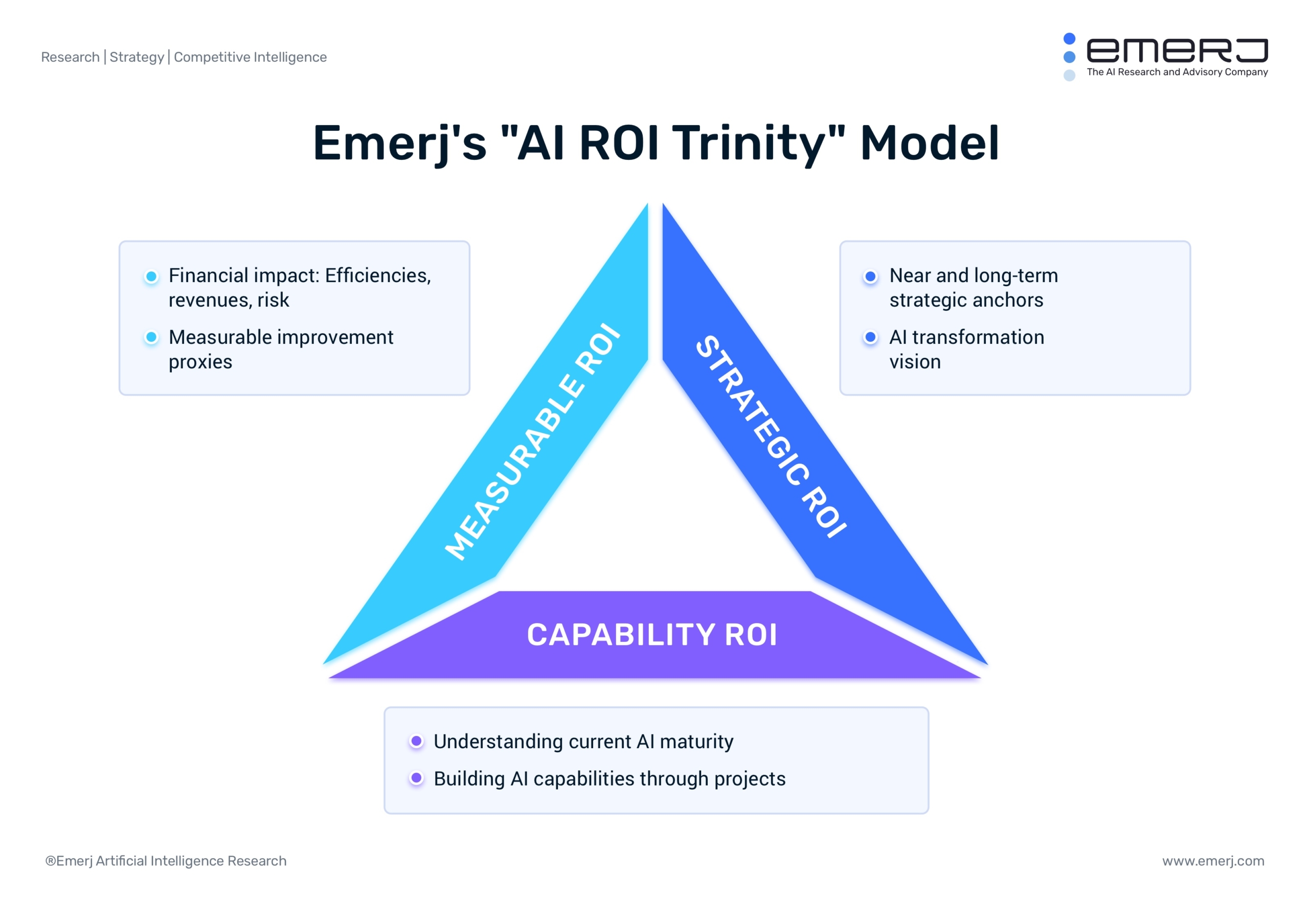
Phase 1: Determine Client Objectives
The first thing we want to do is to understand the client’s current problems and opportunities. These are the driving forces underneath their initial ROI assumptions and goals – and we need to lay them open.
We want to get a sense of what they are struggling with and what they are working or toward. We want to know:
- What immediate problems they’re trying to solve
- What pressing projects they’re working on
- What key thrusts the company has put on their shoulders to deliver on
We want to understand those near-term goals in the context of what we call strategic anchors, such as:
- 3-5 year goals
- Key differentiators
- Digital transformation vision
With these actual priorities at hand, we can start to ask the right question to help “move the needle” on the key metrics the client wants to improve.
Phase 2: Validate the AI Fit
After we have this basic understanding of the client, but before we go into figuring out what measurable ROI metric we want, we would want to determine if there is actually an AI fit. We want to determine the client’s objectives with a purported AI initiative, and if there’s reason to apply AI in the first place.
For example, the client might indicate that they want to use AI for some particular purpose, such as: To respond to customer inquiries and automate a particular loan application process, to extract information from images and influence this workflow in this way, etc…
Roughly four possible scenarios arise:
No project fit:
As a service provider or AI project lead, we may realize other kinds of AI projects we could tie to other than the client’s own ideas about AI projects, which in many cases are rather misguided. They may be embarking on toy projects (AI for AI’s own sake), or they may be looking to solve a radically ambitious goal for which AI is not an ideal fit.
In this situation, we can guide the decision-maker towards more realistic or useful AI goals that are in line with the near-term and long-term priorities that we know they’re focused on now. There is a lot of art and tact in taking someone’s bad idea and artfully allowing them to feel like they came up with a better idea – but this is “inception” approach will work much better than hamfistedly telling the decision-maker a smarter AI application idea that they should have thought of.
Example:
- The decision-maker wants to automate 100% of their customer support emails in the next 12 months. While this is wholly impossible, we might be able to respond to 30% or even 50% (depending on the type of inquiries the firm responds) of the first emails received. By gently telling the client that AI can’t handle entire, complex conversations, we can paint a realistic picture of what it can do – and line up those realistic capabilities with strategic goals that the client themselves said mattered to them.
There’s a project fit – but not with AI:
It is also possible when looking into the AI fit that the client needs a solution that is probably best done without AI. It could be a simple solution that doesn’t need to involve algorithms, training, and datasets at all. We can just build something in it. I would be a little bit wary of this scenario. I would never build an AI project when something could be written with 20 lines of code that would do a better job than AI.
In this situation, it’s best to be frank wit the decision-maker that their initial goal doesn’t require AI. It may be possible to extend the client’s goals into somethign that would involve AI, but only if it’s in the best interest of the client to do so. If the client simply needs a plug-and-play solution to a relatively simple problem, a traditional IT solution will almost certainly take less time to develop and less resources to maintain. If the client if interested in improving AI readiness and maturity, then going a step further to AI might made sense.
Example:
- A decision-maker wants to use AI to execute simple search queries on legal documents. The data set is labelled well enough to execute on these kinds of searches without machine learning. You can choose to (a) inform the decision-maker and build a simple IT point solution, and/or (b) inform the decision-maker about the further kinds of search and discovery functions that AI could unlock across the company’s unstructured PDFs and files. If solving the simple search problem is the decision-maker’s only priority, AI may not be needed. If creating fertile soil for AI-enabled search is a priority, then AI might be a viable additional project.
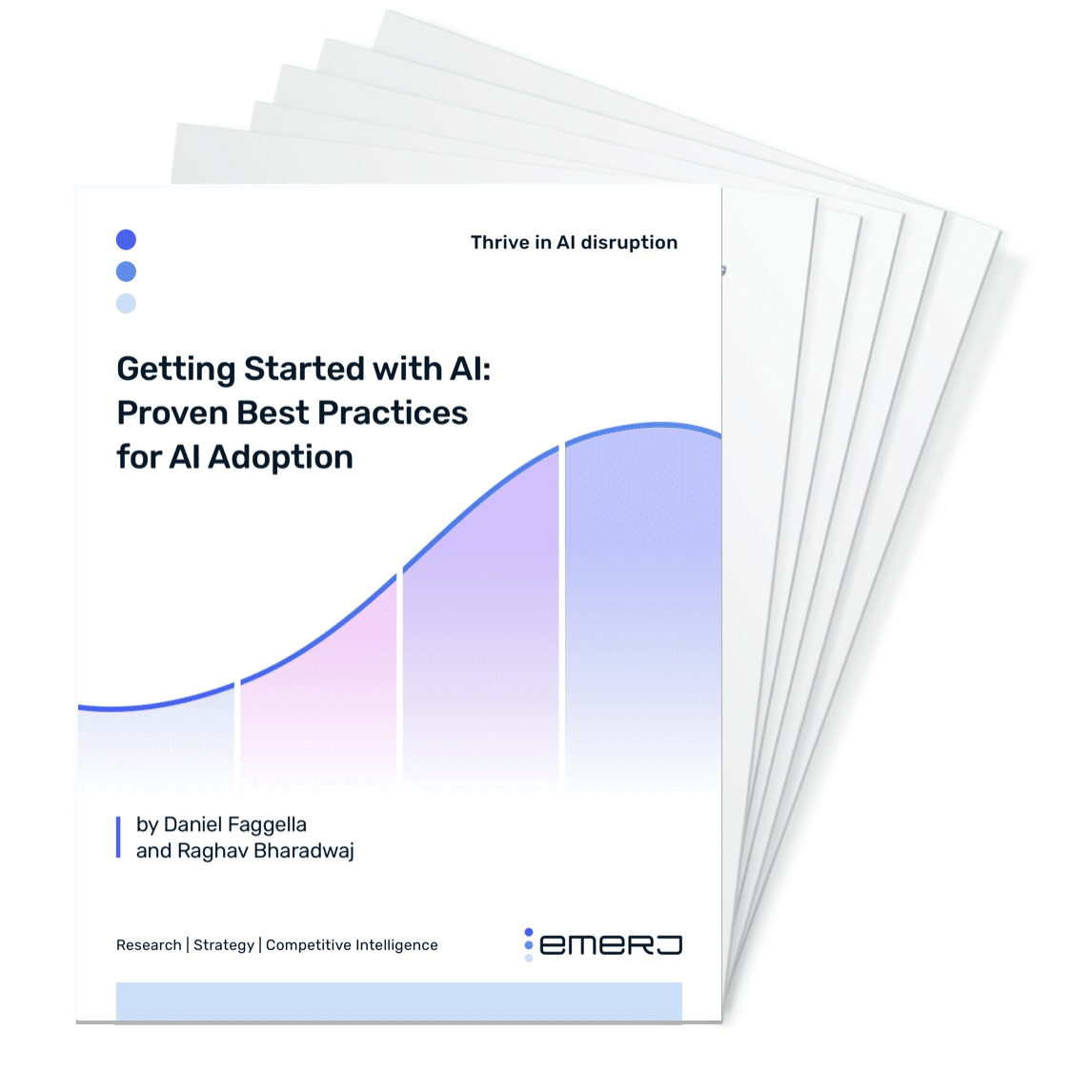
There’s a desire for AI without a tie to value:
In a third scenario, the client is interested in AI for AI’s own sake. Something like:
”Okay, we want to use AI here because it’s going to help us build some skills.”
In this situation, we can use the decision-maker’s interest and enthusiasm. Not to find a random AI project to “check the AI box,” but to open up a conversation about where genuine AI opportunities might lie. Empty motives are a source of wasted resources, and generally decrease the enthusiasm and willingness for future AI projects. Never execute on a toy project when you can anchor a buyer’s enthusiasm to a
Example: A decision-maker comes back from a conference with an interest in AI, and a (usually false) belief that their competitors are farther along in AI development, potentially leaving the firm behind. Rather than building whatever toy projects come to mind for the decision-maker, we can return to strategic and near-term priorities, and use those as openings to discover AI opportunity.
There’s an AI project fit, and a valid adoption motive:
Sometimes, a decision-maker may come to you (the vendor or AI project manager) with an AI suited project, and a valid motive for adopting that project (i.e. that AI is the right tool for the job, and/or that AI maturity needs to be built within a specific portion of the business).
In this situation, our role is more limited, and we can focus on:
- Refining the possibility-space of measurable ROI metrics (see Phase 3 below), and
- Building an action plan for AI adoption for this specific solution, and potentially
- Conveying to the decision-maker a broader AI roadmap for strategic transformation, of which this individual project will be an important part (this is particularly important for service vendors, who can hardly survive on one-off projects and need to create a vision with clients – i.e. a roadmap of future projects to work on)
Phase 3: Expand Measurable ROI Potential
Most of the responsibility for expanding the ROI potential is going to rest on the vendor, consultant, or AI project leader. The project leader will be the one that will have to come up with all the different measurable ROI, ideas, and ways of doing that.
In fact, as mentioned, they’ll often begin with rather unrealistic expectations and benchmarks of success. The decision-maker might be able to help by providing their ideas and perspective, but the responsibility is with the AI project leader to explore the possible measurable ROI metrics for the project, and the requisite benchmarks to hold the project accountable.
Exploring alternatives:
That is not to say that this does not have value to you as a consultant or project leader.
The client is just going to have some assumptions about what needles they think an AI project should move, and these assumptions are not realistic most of the time. However, this will give you at least the opportunity to get access to the economic buyer long enough to get a firm understanding of their goals and priorities. You can then reference them throughout the process of establishing measurable ROI benchmarks and say, “Mr. Buyer, you said that this was most important for you and we kept that in mind throughout the process of coming up with our measurement strategy here.”
Since you are referencing their own assumptions and goals, they cannot deny that you are on the same page. You might not use the metrics they tell you they want, but you are in a good position to play referee as you can say working towards the same goals
Aside from consulting with the client regarding their goals and priorities, we also want to have a few sessions with subject matter experts and the AI project champion on the client side.
For example, if you’re working on a fraud solution, you might be working with the VP of fraud or a couple of very high level fraud managers who really understand the process. You will want to talk to those folks to ask about their ideas of some hard metrics, or something that is objective. This could be:
“Time saved per X” – e.g. it takes us an average of eight minutes to examine an instance of money laundering, and we want to reduce that to six minutes or less.
A soft metric might be something like improving customer experience (i.e. “Improve average customer chat experience from a 3.5 to a 4.2”). While this metric doesn’t tie directly to financials, we suspect that it does in fact improve financial metrics (reducing churn rate, or potentially, reducing future customer service inquiries- i.e. manpower), even if we’re unable to directly attribute it.
The point of consulting with subject matter experts is we want to get ideas of what could be measured based on the process and how to improve the process itself. We also want to use the subject matter experts and the AI champions to validate and refine our measurement ideas based on their own experience and knowledge of the process. We are not going to spend all day to validate ideas with the economic buyer, so we are going to have to do that with the subject matter experts and with the AI champion for the project and list all possible ways that measurable ROI could be generated.
Our Generating AI ROI report is a useful resource for exploring financial and “soft” metrics in great depth. In that case, we want to be able to find any or all proxy metrics to influence goals such as customer retention or customer satisfaction. We want to list both the hard and the soft metrics and think through the perverse impacts and incentives of measurement. The more of both, the better.
Consider incentives:
When I was in graduate school, I studied goal-setting theory on motivation and there are some examples of goal setting theory in the nail factory. If you go to Google and you type in nail factory goal setting theory, you will probably see the same examples. I actually interviewed the founders of this field, oddly enough, and essentially the experiments illustrate that when you go to a nail factory and incentivize performance based on how many nails made , you end up incentivizing workers to make very small nails because then they can make a lot of them. However, if you incentivize them based on how many pounds of nails they can make in a day, then they are going to create very large nails because it takes fewer nails made to achieve the goal.
What this shows is that the way you use incentives can have perverse influences on what you encourage the human beings in the workflow to do. What you want to be able to do is think through the perverse impacts of incentives and measurement to guard against them when establishing your ROI metrics.
In the case of AI, if you are using it to attempt to reduce the time that a customer service person takes to do a particular part of the process such as looking up documents, for example, you need to think about the ways in which a stakeholder can subvert the purpose of the measure. Is there ways in which a manager responsible for reducing that time could make it look that the time spent was reduced without actually making any real improvements or even result in a negative consequence for the overall process?
Example:
A complete brainstorm should probably be done in a spreadsheet, and will look something like this:
Application: A recommendation engine for customizing the eCommerce store pages of individual users based on their behavior or past purchase behavior.
Client: Online apparel retailer.
ROI measure 1: New-visitor-to-customer ratio (currently 2.2%, aim to improve to 4%). Benchmark: Week-over-week change in new-visitor-to-customer ratio across four identified user types. Incentive risks: We may prioritize near-term sales and promotions over lifetime value and customer trust.
ROI measure 2: Average cart value per (new or recurring) user (currently ~$43, aim to improve to ~$60). Benchmark: Week-over-week change in cart value per user by country. Incentive risks: We may prioritize near-term sales and promotions over lifetime value and customer trust.
ROI measure 3: Average annual spend by user. Benchmark: We can track the customer lifetime value of all new customers, month-over-month, from the start of the use of the recommendation engine. Incentive risks: Marketers might allow the experiment to continue for too long without a strong proxy for whether it’s working or not. We need much shorter (maybe per quarter, or per month) cohort metrics to keep this annual metric accountable, and to guage progress as we go along.
Etc…
It bears repeating that this process requires data scientists, SMEs, and the AI project leader to work together – all informed by the priorities of the client or decision-maker. The percentage or dollar-value improvements above are just examples, but real examples need to be tempered by experience (the experience of technical and SME team members), not set arbitrarily.
Having a plethora of measurable ROI metrics to choose from allows us to cull down to a list that is most likely to deliver results and manage the project to a successful deployment.
Phase 4: Rank Measurable ROI Metrics
When ranking measurable ROI metrics, you need to consider various factors, the first of which is client priorities. We would want to look at both near- and long-term goals, with some emphasis on the long-term goals, and determining which are actually achievable. Some of them are going to be easier or harder to achieve.
While scores and prioritization will differ by company and by project, we recommend screening measurable ROI criteria by at least the following:
- Our ability to influence this number with our actions (it’s utility/validity as a metric)
- The relevance of the metric for the decision-maker or client (alignment with their expectations and priorities)
You will then have to determine which are measurable before and after implementing a project to establish a benchmark. In some instances, there might not be a standard available to serve as a benchmark.
Example:
A fraud analyst might go through eight different steps to examine an instance of fraud within an insurance company, and not always in order, or even go through all the steps. The individual fraud analysts will use professional intuition and understanding of fraud to check for it in transactions. That can complicate how to improve the process. If we take one part of that workflow and reduce the time it takes or make it a little easier to do that has a measurable impact, that part of the workflow might not happen in a specific order or at all. There may never be a time when we will be able to measure that metric now and in the future for all fraud analysts in that insurance company. We would have to look at each fraud analyst and adjust the metric to reflect their way of doing their job. That particular process would be unreasonable to measure.
Some things are easier to measure in a before and after sense than others. You might be able to establish some benchmarks today, after the change, or not at all. What we want to do is find obvious things that align with client priorities in the near-term as well as long-term that have achievable, measurable outcomes, and establish benchmarks so we can genuinely attribute our contributions to the results.
Phase 5: Present the Business Case
We want to be able to present our ideas so that when it comes time to bring these measurable ROI ideas to leadership. We want to make it clear when making the pitch that we have taken the client’s near and long-term priorities into account. We want to be able to say that we have used that to find all the different ways that we can achieve your goals and layered our own expertise and research in terms of measurability and achievability to develop realistic strategies to solve their business problems or issues.
It’s important this presenting ROI metrics not be framed as an extra step, a kind of “drag,” but as a benefit – as a part of how we work together with leadership to ensure a project is successful. As mentioned earlier in this article, blindly accepting the assumed ROI metrics of a decision-maker is usually a recipe for failure – and improperly set expectations.
An AI project manager’s value lies in bringing their expertise to bear, collaborating across different kinds of team members, and not only finding the best AI fit, but the best measurements of success for the project.
An AI project manager should be able to convey measurable ROI in the context of strategic ROI and capability ROI. We want to drive home that these metrics are going to help build particular capabilities to achieve strategic near and long-term goals identified by the client.
For example, if the strategic goal is to improve the web user experience and loyalty beyond that of competitors and achieve a 30% market share, the role of the consultant is to identify the most relevant critical capabilities necessary for this project. It might be data, infrastructure, data science talent, or cross-functional teams. We want to bring those up because we want to frame the challenges of AI adoption deployment as positive capabilities to build and not as hurdles to overcome.
When we come up against those friction points again, we would have already framed it as positives well ahead of time. If we don’t do that, then that can be a problem later on. Such hurdles will often result in frustration (decision-maker “Wait… we’ve been working on cleaning data for three months?! Why didn’t you tell me this would be so hard?!”).
For more details on how to structure an ROI case and frame measurable ROI along with strategic and capability ROI, see the AI ROI Cheat Sheet in our reports library.
Concluding Thoughts – and AI Best Practices for Vendors and Consultants
Measurable ROI is not a stand-alone concept, and thinking of it as such when establishing measurable ROI benchmarks will set us up to look bad to the decision-maker (and our entire team), and to ultimately not deliver the value that AI is capable of delivering. We can almost never make guarantees because measurable, strategic, and capability ROI have to work together (see the Three Kinds or AI ROI article linked above), and it is up to AI project leaders or smart AI vendors to make that clear to clients.
Catalyst is a one-to-one a group coaching program hosted by Emerj. Members get access to a full library of frameworks for making the AI business case, successfully selling AI services, and delivering value to clients.
Become the trusted AI advisory that your clients turn to, learn more about the Catalyst Advisory Program and apply online.