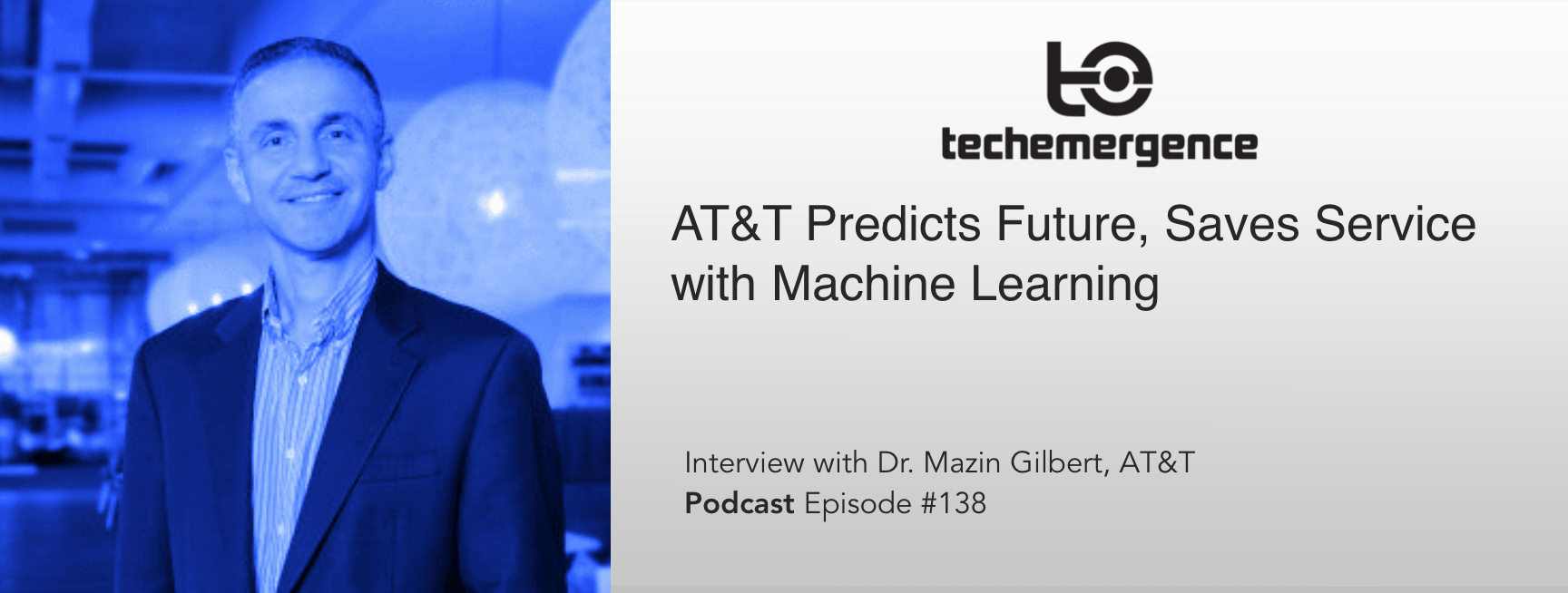
Episode Summary: We’ve featured a number of artificial intelligence researchers on the show, but today we switch gears and dive into the business side of the industry. In this episode, Dr. Mazin Gilbert (who earned his PhD in Engineering) breaks down AT&T’s efforts to make more intelligent systems large-scale. How do they train their network to route traffic through the right nodes on holidays, when certain areas of traffic are overloaded? How can a system know, based on signals from hardware, which pieces might be going bad and need replacing and send out a message to alert the company? Making a network ‘aware’ is a large challenge, but Mazin gives an insider’s perspective as to how AT&T uses machine learning technologies in order to remain profitable.
Guest: Dr. Mazin Gilbert
Expertise: Research & development in industry; human-machine communication
Recognition in Brief: Mazin Gilbert is Assistant Vice President of Intelligent Services Research at AT&T Labs. He has a Ph.D. in Electrical and Electronic Engineering, and an MBA for Executives from the Wharton Business School. Prior to AT&T, Dr. Gilbert worked in the R&D industry at British Telecom and BBC, and in academia at Rutgers University, Liverpool University, and Princeton University. Dr. Gilbert is a well-known technology leader and an international speaker in the areas of communication, intelligent systems and big data. In his current role, he oversees AT&T’s advanced technology in areas of multimodal and multimedia interfaces, machine learning, big data business intelligence and software platforms, and intelligent services. He has published over 100 technical publications in human-machine communication and is author of “Artificial Neural Networks for Speech Analysis/Synthesis”. He holds over 100 US patents.
Current Affiliations: AT&T; Fellow of the IEEE
Next-Generation Communication: Why AT&T Uses Machine Learning
Machine learning is revolutionizing the world as we know it, both in and out of the digital realms, and is predicted to expand to a $2 trillion market by 2025 (as reported by Julie Bort in Business Insider). A data-crunching tool that far surpasses human capabilities, machine learning algorithms are used in tandem with the IoT to create smart appliances and security systems, to identify spam by email providers, produce targeted ads to consumers, and even predict wait times at urgent care and emergency rooms. Companies and universities are coming up with novel ways to use this technology every day.
AT&T is a prime example of a Fortune 500 company that has initiated a major transformation in the communication services industry, transforming its network into what Mazin Gilbert calls a “software defined network.” Gilbert, assistant vice president of Intelligent Services Research at AT&T Labs, describes this as when a “network becomes a software layer riding on commodity cloud hardware; it’s how we’re able to rapidly create cloud service. Machine learning and AI are playing a bigger role in our future, giving us the ability to create the systems in our network that help make it learn and repair by itself.”
When parts of a communications network fail, customers get aggravated. AT&T is hard at work implementing and exploring AI technologies that will quickly identify where break points and down places are in hardware and software, and help to repair those automatically in autonomous ways. “That’s what matters to a user,” says Mazin, “reliability and security in a network.”
In the business of communication and entertainment, it’s all about connecting people with an end device, whether that be a phone, a car, a home sensor, a TV, or some other device. The network becomes the air and the live plug behind the devices, says Gilbert. “This is where machine learning plays a role, when devices do go down, when hardware goes down, we’re using machine learning in predicting what hardware, what machines could potentially go down in the next days, weeks, months; predicting helps us to optimize and route traffic, so that when the impact happens, customers won’t be impacted.”
Smart Systems that Predict, Prevent, and Up Profits
There are many factors that drive failure in the cloud or physical or virtual machines, and most have to do with the environment. Many of those factors can be predicted by both lightning-speed historical analysis and pattern recognition, as well as machine signals. For example, there are patterns of heavy traffic during holidays. AI systems can also pick up signals that they’re receiving from machines—including vehicles in their repair fleet—that indicate potential oncoming failure (similar to our inferences when computers start to run slowly). Right now, the company’s systems are trying to collect a lot of signals and then trying to make predictions in how to identify and optimize traffic.
This ability to predict and prevent, an ability we might align with a type of thinking, is “what’s exciting about machine learning and AI, it’s not about something happening right now; when something happens, or happened, it’s too late…it’s exciting to create an autonomous and intelligent network where we foresee and predict these events from happening before they become disasters,” says Mazin.
Another way that AT&T uses machine learning is analyzing data pulled from contacts, chat, and voice operations. Its ML system is able to process data to make predictions, and do this in near real-time, in turn providing intelligence to managers and supervisors who look and monitor to identify anomalies. Managers and supervisors can ask, “Were my customers happy or not? And if I put them on hold, did that make them unhappy? Did my agent solve their problem the first time? We’re using machine learning to determine customer sentiment, why they called, will they call again…it’s capable of a large number of predictive capabilities at scale,” says Mazin.
As the company continues to explore how AI can be used at scale to improve the customer service, there are two major aspects to consider, says Gilbert. One is scale, since the company is looking at how to use these systems across data centers and traffic sources. The second is “revolution of compute and data,” which has to do with looking at events closer to real-time (as opposed to weeks or months) and identifying anomalies at data centers much more quickly.
Today, much of the data being analyzed by the AI systems in place are provided to a large spectrum of managers, who are then using this information to take actions. Machines learn simultaneously during these exercises. “They can’t be creating predictive models of large data that are static, because they become obsolete, this is one of the challenging things; the machine has to continuously learn every day of the week, because patterns and language and problems of traffic are always changing,” explains Mazin. AT&T is actively trying to close the loop between machine and human intervention, by actively searching out opportunities where a machine can take the action without bringing in a manager.
AT&T also recently partnered with IBM to leverage Watson’s cloud technology, which will give the company’s business customers the ability to tap into the IoT and glean their own signals necessary for prevention and maintenance of their machines. AT&T will also use this data to build more robust machine learning models and produce libraries and open-source technology for more precise predictions and preventive actions against machine failure. AT&T’s business clients also benefit from the company’s machine learning and advanced analytics technology, AT&T Threat Intellect, in defending against security threats across the AT&T network.
“We are using this new technology and data that we have to improve our products and services in ways we couldn’t do before, to be able to ingest and process this volume of data, in close to real-time, requires tremendous compute, storage, bandwidth – this has changed. Now, we’re able to do all this simultaneously at scale and come out with a decision very rapidly,” says Gilbert.
Image credit: LinkedIn