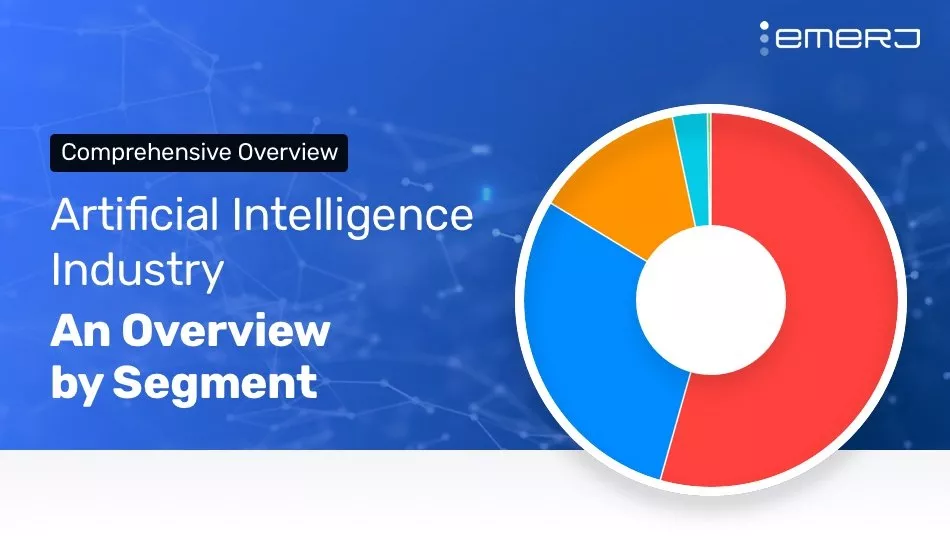
Today’s artificial intelligence market is not easy to quantify. Besides the lack of consensus on a coherent definition for “artificial intelligence” as a term, the field’s nascent stage of development makes it difficult to carve out silos or hard barriers of where one industry or application ends, and another begins.
In one of our more popular recent articles, we aimed to derive a valuation of the artificial intelligence market, based on current market research and our own insights. In this week’s article, I’ve set out to determine more of a “lay of the land” of the AI industry, including it’s various segments and application areas.
If you’re interested in how developments in machine learning and AI might impact your own company or business, then keeping an eye on trends of industry and application growth is pertinent; we hope that this article will be a good jumping off point to some of the most thought-out assessments of AI and it’s “segments” as we could collate from the web. We’re unable to ensure accuracy of all of the data sets and research from other companies and researchers, but we’re happy to put them on display here for you to dig into and make sense of.
This article serves as a living overview of existing efforts by media, research firms, and others, who have attempted to move from the eagle eye’s view of the AI industry to categorizing technologies under the grand umbrella. Older breakdowns and analyses begin at top of the article and move down to newer trends. In cases where a company or individual has published annual updates of the same breakdown, we’ve kept those breakdowns together for ease of comparison. We end by pulling together what we believe to be key meta-trends and insights from these ongoing analyses.
BCC Research Smart Machines Market Report (May 2014)
BCC Research presents their growth forecasts for 2019-2024 (in millions) below:
The graphic above is from the Siemens’ corporate blog. Numbers in parentheses are in millions:
- Autonomous robots: 31% (3,582 – 13,927)
- (including self-driving vehicles)
- Digital assistants: 30% (2,175 – 8,075)
- (e.g., Siri, automated online assistants)
- Neurocomputers: 22% (1,590 – 4,685)
- Embedded systems: 19% (877 – 2,095)
- (machine monitoring and control systems)
- Expert systems: 12% (7,055 – 12,433)
- (e.g., medical decision support systems, smart grid)
It is interesting to note that BCC predicts the highest aggregate 5-year growth rate in the area of digital assistants, which seems to corroborate with our own 5-year AI trends executive consensus.
O’Reilly State of Machine Intelligence 1.0 (December 2014)
Bloomberg’s Shivon Zilis breaks down the “Machine Intelligence Ecosystem” into a number of categories and sub-segments:
Zillis’ first attempt breaks down the machine intelligence “landscape” as follows:
Core Tech
- AI
- Deep Learning
- Machine Learning
- NLP Platforms
- Predictive APIs
- Image Recognition
- Speech Recognition
Rethinking Enterprise
- Sales
- Security/Authentication
- Fraud Detection
- HR/Recruiting
Marketing - Personal Assistant
- Intelligence Tools
Rethinking Industries
- AdTech
- Agriculture
- Education
- Finance
- Legal
- Manufacturing
- Medical
- Oil/Gas
- Media/Content
- Consumer Finance
- Philanthropies
- Automotive
- Diagnostics
- Retail
Rethinking Humans/HCI
- Augmented Reality
- Gestural Computing
- Robotics
- Emotional Recognition
Supporting Technologies
- Hardware
- Data Prep
- Data Collection
O’Reilly State of Machine Intelligence 2.0 (December 2015)
Shivon’s updated 2015 graphic lists dozens of companies, though it seems clear that many more had to be left out due to limitations of the size of this graphic. In this case, we see a different and distinct set of segments than in other research graphics, as well as the inclusion of non-profits (i.e.: OpenAI) and open source technologies (i.e.: Caffe).
The full breakdown of categories above are listed below:
Agents
- Professional
- Personal
- OS Interfaces
Autonomous Systems
- Air
- Ground
- Sea
- Industrial
Enterprise
- Security / Fraud
- HR / Recruiting
- Sales
- Marketing
- Customer Support
- Internal Intel
- Market Intel
Platforms
- Research / AGI
- Full Stack
- Machine Learning
- Industrial IOT
- Audio
- Vision
- Data Enrichment
Industries
- AdTech
- Agriculture
- For Good
- Retail Finance
- Legal
- Materials & MFG
- Healthcare
- Education
- Transport & Logistics
- Investment Intelligence
Tech User Tools
- Data Science
- Machine Learning
- Open Source
Notable quotes:
The two biggest changes I’ve noted since I did this analysis last year are (1) the emergence of autonomous systems in both the physical and virtual world and (2) startups shifting away from building broad technology platforms to focusing on solving specific business problems.
O’Reilly State of Machine Intelligence 3.0 (November 2016)
Key differences noted in Zillis’ Machine Intelligence 3.0 version are more pronounced and refined, as technologies and the industry continue to advance:
- Enterprise is further segmented into Intelligence (which we might define as open-ended insights) and Functions (tools that serve an end cause).
- The Platforms and Technology User Tools in version 2.0 were consolidated, updated, and refined into an emergent category — Technology Stack — which also makes room for the subcategories hardware and development.
- Healthcare notably earned its own category, separate from industry.
- Again, it’s safe to say that noted companies are not all-inclusive but meant to provide an idea as to some of the bigger and more established companies in each of these spaces.
The full breakdown of categories above are listed below:
Enterprise Intelligence
- Visual
- Audio
- Sensor
- Internal Data
- Market
Enterprise Functions
- Customer Support
- Sales
- Marketing
- Security
- Recruiting
Autonomous Systems
- Ground Navigation
- Aerial
- Industrial
Agents
- Personal
- Professional
Industries
- Agriculture
- Education
- Investment
- Legal
- Logistics
- Materials
- Retail Finance
Healthcare
- Patient
- Image
- Biological
Technology Stack
- Agent Enablers
- Data Science
- Machine Learning
- Natural Language
- Development
- Data Capture
- Open Source Libraries
- Hardware
- Research
Notable quotes:
For the first time, a “one stop shop” of the machine intelligence stack is coming into view—even if it’s a year or two off from being neatly formalized. The maturing of that stack might explain why more established companies are more focused on building legitimate machine intelligence capabilities.
and
Machine intelligence is different from traditional software. Unlike with big data, where you could buy a new capability, machine intelligence depends on deeper organizational and process changes.
CB Insights Various AI Reports (2016)
CB Insights is a New York-based research firm with under 200 employees, specializing in tech intelligence from various sources, to include venture capital, startups, patents, partnerships, and new media. They seem to have created some of the most relevant graphics and information about the broad AI market.
I’ve organized a number of their graphics, along with coinciding links to their reports and data platform.
In the graphic above, CB Insights highlights the AI acquisitions and fundraising deal frequency across various industries. Their own research shows healthcare topping the list, with marketing / advertising and business intelligence trailing only slightly in 5-year deal volume.
Noteable quotes:
AI in healthcare accounted for 15% of all equity deals to artificial intelligence startups in 2015. Smart money VCs have backed companies including Lumiata, SigTuple, Deep Genomics and twoXAR.
The graphic above displays AI funding activity from major investment firms over the last 5 years. CB Insights shows Intel, Google, and GE topping the list. The data in this illustration seems to be derived from the number of companies funded rather than number of dollars funded (though there is likely a correlation between the two). Knowing who is investing in what companies (and which technologies) is useful knowledge for other industry leaders who want to understand where the “smart money” is finding a home in the AI market.
The graphic above showcasing well-funded AI startups is particularly interesting because it seems to demonstrate that “long shot” AI platforms and core technologies have garnered significantly more investment than companies focusing on specific technology problems of the next few years.
In the days of the internet, there were battles between Netscape, Yahoo, Microsoft, and others, in order to determine who would “win the web.” It might be said that Google came out as the winner of web search and consumer web applications broadly speaking. Investors are interested in investing the same kind of game-changer technology in AI, which seems to give broadly-focused companies like Sentient Technologies and Vicarious Systems some of their allure.
It seems prudent to keep an eye on where these aspiring AI “industry standard” companies focus their efforts, as those domains will almost invariably gather more momentum and excitement as this nascent field looks to new leaders vying to become global AI platforms / solutions for direction and focus.
Nvidia’s Report on GPU Usage (January 2016)
Graphics processing unit (GPU) is a specialized electronic circuit, designed to rapidly manipulate and alter memory to accelerate the creation of images in a frame buffer intended for output to a display. Nvidia is one of the world’s leading GPU manufacturers, and they have chronicled and visualized the growth of GPU-related sales into various industry segments, as seen below:
While GPU usage is by no means a causal influence on the applications of AI in a given industry, it provides a certain amount of context on industry growth (Nvidia claims to have sold GPUs to nearly 100 times more companies in 2015 than in 2013, a significant leap). Even in 2015, we can see that higher education (which we can presume to imply university research) is the largest consumer of Nvidia’s GPU technology.
In order from greatest to least percentage of GPU industry users:
- Higher Ed
- Internet
- Life Sciences
- Development
- Finance
- Media & Entertainment
- Government
- Manufacturing
- Defense
- Automotive
- Gaming
- Oil/Gas
It can be presumed that the shrinking relative percentage of sales to “Higher Ed” will continue as more companies in all sectors begin adapting machine learning into their regular processes.
Notable quotes:
In just two years, the number of companies NVIDIA collaborates with on deep learning has jumped nearly 35x to over 3,400 companies. Industries such as healthcare, life sciences, energy, financial services, automotive, manufacturing, and entertainment will benefit by inferring insight from mountains of data.
Comet Labs AI and Robotics Startup Landscape (February 2016)
Comet Labs is a venture fund focused specifically on artificial intelligence-oriented technologies, and they’ve done a good deal of their own homework in assessing the industry at large. Their own effort to map the landscape of AI and robotics startups can be found below:
The synopsis of their brief findings can be found below:
While this graphic does draw attention to various discrete industries and companies, it seems to be a bit more broadly focused and includes a number of startup but also a handful of companies like Yaskawa (founded over 100 years ago), Apple (Siri) and Nvidia, which don’t seem to belong in a graphic labeled as “startups.”
That discretion aside, the article also doesn’t make it clear how many companies were assessed, or how and why the industry delineations were drawn out (where is “natural language processing” or “assistants” or “marketing / advertising”?)
The graphic does help draw attention to some of the major application areas of AI (and mirrors Comet Labs’ own logo), but doesn’t appear as comprehensive or to draw conclusive enough insights about the current “industry breakdown” to warrant serious merit.
In the Comet Labs article in which those graphics were featured, there are interesting and useful examples given of the ongoings of specific startups within the various sectors highlighted.
(Note: You can listen to Emerj’s interview with Comet Labs’ Managing Director Saman Farid on our podcast).
VentureScanner AI Industry Assessment (March 2016)
The following AI Sector Map breaks down into 13 broad categories, with the total number of active companies in that sector in parentheses:
The results from VentureScanner’s March 2016 report are as follows:
- Deep learning/machine learning (general) (123 companies)
- Deep learning/machine learning (apps) (260 companies)
- NLP (gen) (154 companies)
- NLP (speech rec) (78 companies)
- Computer Vision/Image Rec (gen) (106 companies)
- Computer Vision/Image Rec (apps) (83 companies)
- Gesture control (33 companies)
- Virtual personal assistants (92 companies)
- Smart robots (65 companies)
- Rec engines and collaborative filtering (60 companies)
- Context aware computing (28 companies)
- Speech to speech translation (15 companies)
- Video automatic content recognition (14 companies)
Other charts on the VentureScanner report page show funding by AI category (by far most are in machine learning (ML) apps, followed by NLP); venture investing in AI (most in ML apps, followed by NLP); AI total funding annually (accelerating since 2010); average funding by AI category (most in ML apps, followed by smart robots and gesture control); average age of technology by AI category (speech to speech translation oldest, followed by gesture control, video content recommenders, and speech recognition); among others.
A total of 910 companies were accounted for in this report. VentureScanner’s same “sector analysis” for the previous year counted 633 companies.
Emerj Executive Consensus on AI Trends (June 2016)
Our own recent AI industry research involved polling over 30 AI startup founders and company executives (with firms as small as six people and as large as 600). Our first consensus question asked about AI consumer technology applications in the coming five years. A sample of the full consensus graphic can be found below:
The vast majority of companies interviewed had nothing to do with chat bots or personal assistants, yet over a third of all executive responses expressed confidence in chat bots as the most influential consumer AI technology in the coming give years.
It’s important to note that the question of technology trends was presented in an open-ended fashion, and categories (such as “smart objects / environment”, “virtual agents”, etc…) were applied after analyzing individual responses.
Other Vertical Breakdowns
There are a number of other attempts to value and properly segment AI verticals. Below are a few worth considering:
Markets and Markets breaks out AI verticals into the following main categories in their 2020 AI Forecast:
- Media & advertising
- Finance
- Retail
- Healthcare
- Automotive & transportation
- Agriculture
- Law
- Oil & gas
- Others
Tractica’s recent AI Applications in Enterprise report breaks down technology forecasts as follows:
- Cognitive Computing
- Machine Learning
- Deep Learning
- Predictive APIs
- Natural Language Processing
- Image Recognition
- Speech Recognition
- Other AI Technologies
Takeaways on Artificial Intelligence Market Segmentation
The amount of reliable information about the AI market is less than ideal, and far less quantified than more mature and established markets. Regardless, there is still insight to be gained, and below are outlined some of the most important takeaways from an assessment of previous efforts to segment the aAI market.
1 – Healthcare, Marketing, and Finance Consistently Appear as Areas of AI Focus
CB Insights claims that healthcare has been the domain of greatest deal flow in AI. Google’s DeepMind is honed in on healthcare, IBM set it’s sights on healthcare years ago (and continues to burrow into that market), and many of the biggest “broad AI” players like Ayasdi are jumping into the healthcare market.
Healthcare also offers a kind of noble “flavor” that other application areas do not. AI companies who begin by working on Wall Street may be perceived as simply profit-driven, or possibly helping the wrong party, while a company devoting itself to curing disease or improving treatment (even if for the exact same profit motive) may be viewed in a different light. It’s my contention that companies like DeepMind who are interested in moving towards strong AI will have to move forward with “friendly” steps into noble fields like medicine in order to dispell some of the fear around progress towards machines that may (one day) become more intelligent than humans.
Marketing and finance also represent huge areas of AI focus. Sentient Technologies’ Aware software promises to deliver better conversion rates for eCommerce vendors, and Cortica‘s myriad applications for eCommerce and marketing will be fleshed out in the coming months and years.
All three of these commonly targeted AI segments – health, marketing and finance – involve a tremendous amount of high-volume information, and all three segments are nearly infinite in size. I’m of the belief that the convoluted sales cycles and market forces in health care will lead to finance, eCommerce, and marketing leaping ahead in terms of relative AI adoption and innovation, though only the future will tell. What seems certain is that these three fields will be among the biggest domains of focus for AI firms, and these application areas are themselves likely to spawn many scientific innovations in AI itself.
2 – Segmenting Markets is Qualitatively Messy, but Might Clear Up in Time
Determining segments is a high-level art and science; it is a creative task that requires a well-informed perspective. A market can hypothetically be “sliced” in an infinite number of ways (depending on one’s purpose), but accurately boxing applications into specific “verticals” involves a lot of grey area. Consider the following:
- CB Insights does not have “natural language processing” (NLP) listed in their vertical breakdown of AI deals, though there are a great many companies working on NLP itself, not just NLP for eCommerce, but for smart homes, etc…
- AI can be an entire business (RocketFuel) or a LOT of what a company does (Yahoo) or some of what a company does (IBM) – how much AI does a company or deal need to be directly involved in order to get placed in an “AI Industry” category?
- CometLabs’ industry breakdown doesn’t mention marketing / finance at all, despite the fact that this is one of the most prevalent application areas of AI today and in the near future
- The same company could easily be called “eCommerce” by one analyst, “Marketing” by another, and “both” by yet another, an issue that is terribly difficult to work around with an increasingly large number of possible segments
This is not to say that any of the completed AI industry landscapes are “wrong” (I happen to like CB Insights’ breakdown as a good general breakdown, and BCC has a nice simple segmentation as well), but that fuzzy edges will always be present in this kind of work, and that a consensus across research firms is unlikely (indeed, a consensus within any one research firm seems unlikely).
The “grey area” around and within a specific AI segment may make it more valuable to focus on one industry at a time, firmly identifying the grey areas and the direct applications, without having a thousand points of “fuzzy” overlap between 12 or 20 discrete industries. For example, if one is just analyzing “AI for Business Intelligence,” one can construct a relatively rigorous set of classifying rules to determine what does and does not get couched under the header of “AI” and “Business Intelligence.” Much of our future work here at Emerj will involve specific industry deep dives of this kind.
3 – No One Knows What Will “Take Off,” and This Influences the Behavior of the Industry
At a recent VentureBeat conference, Robert Stephens (former founder of Geek Squad) mentioned that the world of AI chat bots is at the phase that the internet was at in 1994. We don’t know were applications will pick up, or take off, and in many fields, use cases are still taking shape as “experiments” and not as direct and succinct drivers of business value.
Hence, many companies are “feeling out” where to apply these technologies (going from “AI platform” to solving specific business problems). Conveying the value of AI has proven difficult for many vendors. Companies like Sentient Technologies have confusingly broad demo videos (see their explainer video here) that could leave one wondering, “What will this do for my business?” IBM has even been criticized for it’s inability to convey the value of Watson to clients.
This “not knowing what will be a big deal” has – I believe – resulted in a number of observable behaviors and patterns in the AI industry:
- Consistently huge bets in the future “Google of AI”, ‘industry standard’ AI platforms (that battlefield is still wide open, and it’s uncertain how it will congeal, or if it will continue to fragment over time)
- Companies with broad application areas beginning to explain their technology in “non-PhD” language, and in terms that programmers and CEOs can understand (a good example here is Ayasdi – their technology is tremendously complex but they explain their value proposition and use cases in this explainer video and this use case video)
- AI vendors and large company executives are both looking to the “cool kids” (hottest new AI companies with tens of millions in funding, and the biggest AI tech firms like Facebook, Google, Amazon) for determining future trends, and where they should go next. Since many AI vendors and large organizations don’t have “traction” in terms of real ROI, they must look to where they believe traction to exist.
4 – Specifics Up for Grabs, but Optimism Abounds
Despite the disagreements about specific industry or domain application and adoption, the rumble on the tracks (from all directions) seems to liken AI not to a specific tool for a few specific jobs, but as an entirely different (and in large part unimaginable) paradigm of work, research, and productivity.
Twenty years ago, Bill Gates locked himself away to contemplate “the internet” and decide how important it would be for Microsoft’s future. Thank goodness he took action. Today, Microsoft’s CEO and Google’s CEO have both expressed their extreme commitment to bringing AI into the core of their business and their plans for growth. It’s not just Silicon Valley companies on the bandwagon anymore, and we could never have prepared for the exact roll-out of massive changes brought about by the internet. Similarly, it is unlikely that we’ll be able to foresee many of the most powerful applications of AI in business and personal life – but we can keep our ears to the tracks.
[This article has been updated as of April 5, 2017 to reflect new research and industry progress in the field.]