Meta Platforms Inc. (herein, “Meta”), was known as Facebook up until 2021. Mark Zuckerberg states that the new brand embodies his strategic plan to create a “metaverse” for its customers using AI and VR technology.
In 2021, Meta reported a net income of approximately $39 billion on revenues of just under $118 billion. Currently, Meta is traded on the NASDAQ (symbol: FB) and has a market cap of approximately $497 billion. Sources vary somewhat regarding the number of employees at Meta, though current estimates from reliable sources place the number somewhere around 70-77,000 workers.
In this article, we’ll look at how Meta has implemented AI applications for its business through two unique use cases:
- Removing Offensive Content – Meta uses machine learning and natural language processing to screen for and remove offensive material such as sexual and violent content.
- VR Immersion – Meta claims to use computer vision algorithms to track user movement in real-time for its Oculus product.
We’ll begin by examining how Facebook has purportedly focused on using AI to remove offensive content.
Use Case #1 – Removal of Offensive Content
Ever since the Facebook-Cambridge Analytica scandal in the 2010s, Facebook has been under heavy scrutiny by federal regulators, privacy and security authorities and advocates, and other concerned parties.
But it isn’t just user privacy that is of concern. Jerome Presenti, Meta’s VP of artificial intelligence, says removal of what the company calls “harmful content” such as that which contains sex, violence, and other inappropriate material is also considered a priority.
In a podcast interview, Pesenti discussed some of the purported uses of AI in this regard. Pesenti claims that Meta is analyzing text and image data for harmful content using what seems to be natural language understanding (NLU) and computer vision models. One method by which Meta is supposedly using NLU and computer vision to combat harmful content is a machine learning application called “few-shot learning,” or FSL.
Below is a video describing Meta’s FSL model:
Meta claims that its FSL solution can be trained with limited or no training data. The company states that it uses multimodal data retrieved from past users. The model is trained in sequence on three types of data: billions of generic and open-source language examples, data that Meta has labeled harmful in the past (please see disclaimer below), and condensed text that explains company use policy.
The company claims that its FSL models “adapt more easily” to rapidly evolving content and take the appropriate corrective measures, e.g., content removal. The company claims it does this by “starting with a general understanding of a topic” and then using “fewer labeled examples to learn new tasks.”
Please note: The information contained in the next two paragraphs are merely to relay facts to our audience, not to decry Facebook for its labor practices.
Before proceeding, it must be mentioned how Facebook is likely continuing to label data. In a 2019 article in the Washington Post, it was revealed Facebook and other social media companies outsource “tens of thousands of jobs around the world to vet and delete violent or offensive content.” In another article, it’s reported that many of these workers operate in poor working conditions while receiving a lack of mental health support in a job that requires them to review explicit and potentially traumatic material all day.
The above is noteworthy because prior to implementing its AI-based solution for identifying and labeling harmful content – and perhaps even after – mass labor practices such as those in the Philippines and elsewhere was/is one of the main ways that Facebook managed to keep such content off its platform. To be clear, it is possible that Facebook continues to possibly subject overseas labor to potentially substandard labor conditions in labeling harmful content data.
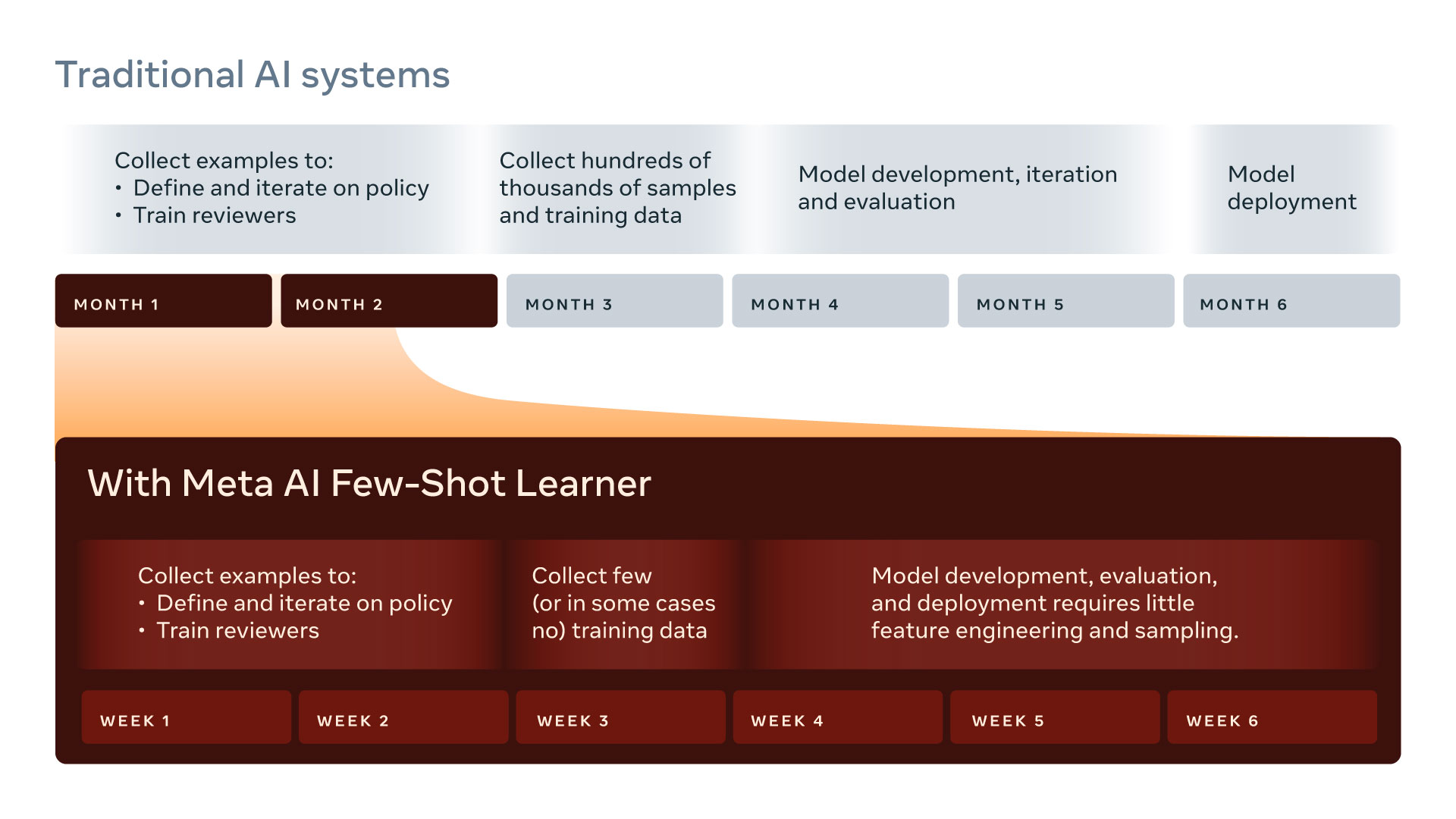
Meta claims to overcome the complex problem of removing rapidly-evolving harmful content by using proprietary self-supervised learning techniques and more efficient FSL models. The company asserts that its model drastically speeds up training data collection and model development, interaction, evaluation, and deployment.
Regarding outcomes, the company claims that its FSL solution reduces the required time to teach the model new and harmful content from six months to six weeks. The FSL solution also purportedly works in more than 100 languages, learns from image and text data, and strengthens existing AI models already deployed to detect harmful content. The new model also outperforms “various state-of-the-art few-shot learning methods” by an average of 12 percent.
Use Case #2 – VR Immersion
According to Facebook, virtual reality (VR) and augmented reality (AR) for their Oculus Quest headsets depend upon “positional tracking that [is] precise, accurate, and available in real-time.” Moreover, the company claims that this positional tracking system must be compact and energy-efficient enough for a standalone headset.
Meta claims that its “Oculus Insight” (also called its “insight stack”) machine learning models leverage the latest computer vision systems and visual-inertial simultaneous localization and mapping, or SLAM. SLAM is used to track the position of the user’s head, while constellation mapping is used to track head movements. Other applications that use SLAM include autonomous driving and mobile AR apps.
Meta claims that the Quest relies on three types of sensor data, each producing a different output. First is image data from 4 ultrawide cameras in the headset, which generates a 3-D map of the room and pinpoints boundaries and landmarks. Next is rotational velocity and linear acceleration data from the inertial measurement units in the headset and controllers, which tracks head and hand movements. The third is infrared LED in the controllers, which are detected by the headset cameras, enhancing the unit’s ability to track controller movement.
Below is a video that gives an overview of the technology embedded in the Oculus Quest:
Regarding product outcomes, Meta claims that it was able to:
- produce the “first all-in-one, completely wire-free VR gaming system” in the Oculus Quest using these algorithmic models.
- create the first consumer AR/VR product that uses “full untethered” (unwired) six-degree-of-freedom (6DoF) movement alongside controller tracking.
- reduce energy use by two orders of magnitude over consoles.
- render real-time, high-end graphics at framerates that are either comparable to or higher than PC and console games.
Finally, concerning revenue impact, Meta earns money from sales of both the headset and purchases in the Oculus Quest store, where it sells apps and games for the platform. Regarding the former, various sources cite the Oculus Quest 2, the company’s most recent product, as the best-selling on the market.
In terms of quantitative outcomes, Meta claims in its 2021 earnings report that spending on its Oculus Quest store (where the company sells VR games and apps) had increased significantly – from $12 million to $51 million from 2020 to 2021. The company claims that total consumer spending in the store has exceeded $1 billion since the launch of the first Quest headset in 2019.