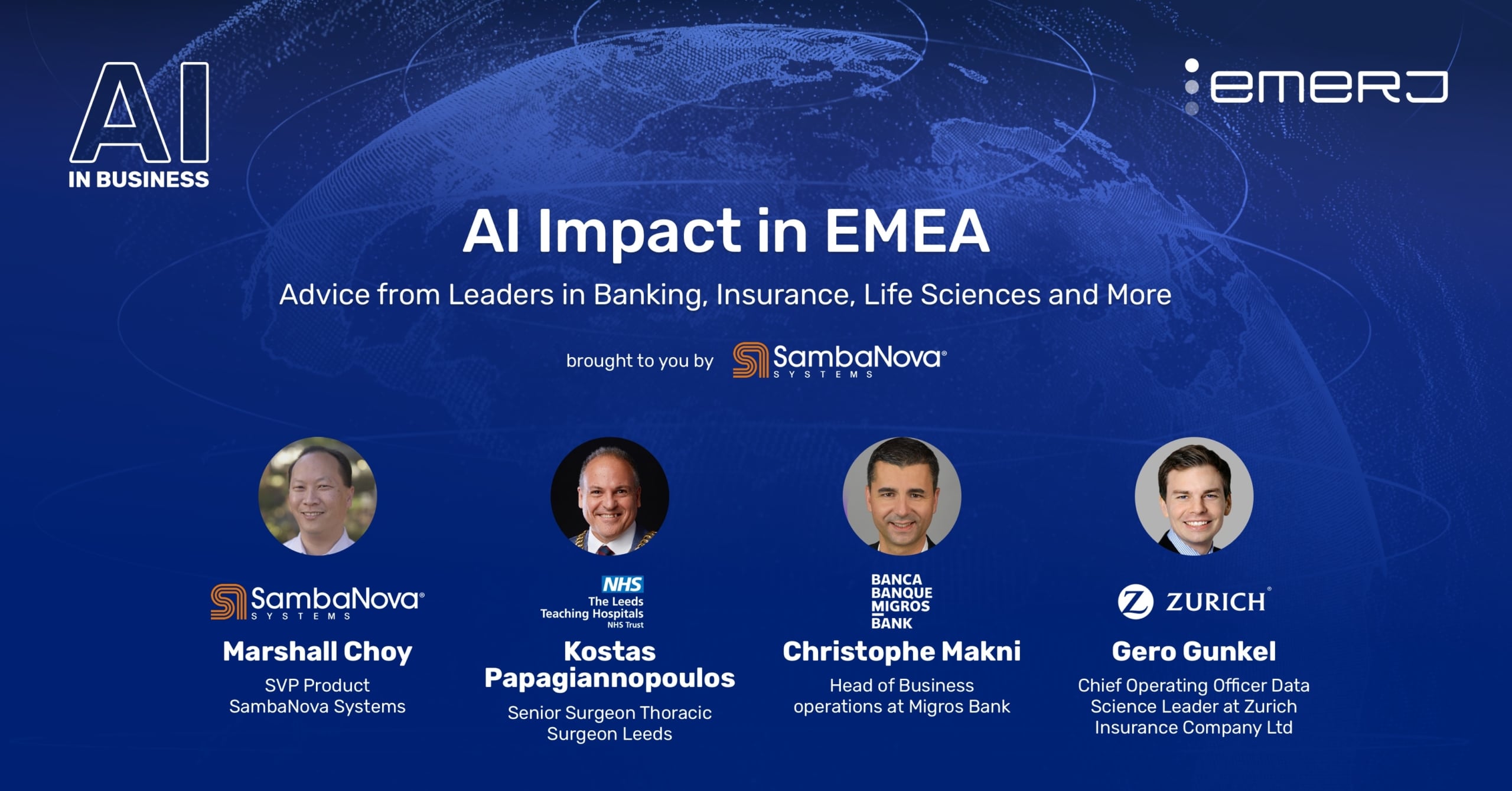
This article is sponsored by SambaNova, and was written, edited and published in alignment with our transparent Emerj sponsored content guidelines.
Learn more about our thought leadership and content creation services on our Emerj Media Services page.
Different cultures, industries and markets have varied ways of thinking about AI.
For these reasons, a large part of the focus of the “AI is Here” series within Emerj’s AI in Business podcast consists of looking at unique challenges, use cases and adoption trends seen by leaders from across a range of industries and regions.
A great example of one such use case exists in the prevalence of large language model (LLM) applications across what are called “language-heavy industries” — banking, finance, and healthcare to name a few.
In the following breakdown of conversations with industry leaders, we not only highlight impactful AI use cases but also closely examine the unique business dynamics taking place beneath AI adoption strategies.
Every episode featured in the “AI is Here” podcast series focuses on where AI is impacting businesses and industries every day, no matter the location. In this particular rundown, our featured expert guests come primarily from across the EMEA regions to offer a diverse cross-section of perspectives.
Yet what they primarily offer are five actionable insights for enterprise professionals on the following AI adoption strategies and how well they work best in their their corner of the global economy:
- What makes an AI adoption successful
- AI’s impact on patient care
- Implementing AI in finance
- How natural language processing (NLP) is changing insurance
- How knowledge graphs can “wake up” latent data sources
Throughout all five episodes, these enterprise leaders emphasize the importance of:
- Finding use cases that drive enthusiasm in early projects
- Having the long-term vision necessary to reach AI “transformation”
- The fundamentals of getting management buy-in
Emerj would like to thank our guests for sharing their knowledge and perspectives on AI adoption. In the episode summaries below, enterprise leaders can find a breakdown of these insights and how best to frame their application to the widest possible range of industries and contexts.
AI Impact in EMEA – Expert Insights Across Industries
EPISODE 1 – What Successful AI Adopters Are Doing Differently
This series kicks off with SambaNova’s Senior Vice President of Product Marshall Choy, with a global perspective distinguishing between organizations that become successful AI adopters and those that don’t.
When it comes to being a successful adopter of AI, it all starts with a coordinated, company-wide approach. Small groups of what Marshall calls AI “flag wavers” within a company aren’t going to lead to successful, routine AI adoption. What’s needed instead is collaboration, organization, and, most importantly, education about what AI is and what it can do to drive business value – especially at the executive level.
Marshall also discusses his perspective on AI trends and evolution, and where he’s seeing the implementation of large language models (LLMs). The commonality among industries leveraging LLMs is usually that they are speech, text, and/or document-heavy. In particular, banking and financial services are traditionally thought of as “language-heavy Industries”, in Choy’s words, where professionals are looking at AI use cases in both the front end (call centers) and the back end (risk and compliance) that streamline workflows.
According to Choy, these changes in workflows are leading to shifts in thinking across these “language-heavy” industries toward single large language models as the foundation of organization-wide transformation through AI. In a recurring theme between many of our conversations featured today, Choy emphasizes that such transformation is only possible with long-term strategic thinking from the early phases of the project
EPISODE 2 – Guiding the Patient’s Treatment Journey with AI
Guest: Kostas Papagiannopoulos, M.D. – Thoracic Surgeon, Leeds Teaching Hospitals; Honorary Senior Lecturer, Leeds University
Kostas Papagiannopoulos, M.D. of Leeds Teaching Hospital elaborates on a particularly promising use case for AI in the medical arena: treatment management. He discusses how proper data gathering – in concert with AI – can overcome many challenges subjective and widely variant patient data. Dr. Papagiannopoulos also elaborates on the importance of coaxing answers that can be translated into data inputs, such as training data for machine learning.
Getting answers from data is about eliminating bias and potential errors, doing a thorough analysis, and guiding people into giving answers that translate into data. He gives an example case of patients receiving care for breast-related conditions; by simplifying the questionnaire for people of different socioeconomic and educational backgrounds, he was able to obtain the objective data to input into the AI model his team needed.
By first taking in the right data at the right points and accounting for human biases and subjectivity, an AI solution that guides something as complex and dynamic as a treatment regimen becomes a very real possibility.
EPISODE 3 – Creating a Culture of Innovation for AI
Guest: Christophe Makni – Head of Business Operations, Migros Bank
Christophe Makni of Migros Bank discusses how financial organizations can greatly increase their odds of successful AI implementation by encouraging leadership buy-in and education.
As someone that has worked in evaluating and implementing AI in the at-times-stodgy world of the banking industry for many years, Christophe gives cogent insights on what successful financial enterprises are doing – and it all starts with leadership.
First, organizations must ensure that leaders have a fundamental understanding of AI and its capabilities. Then, they must have the courage and vision to invest in AI and see the differences that it can make. Without this buy-in from leadership, there’s little to no progress.
Christophe also weighs in on the efficacy of Europe’s approach to AI and the way new European Commission rules emphasize working groups, or teams of AI experts from peer organizations who share insights with one another. He also talks briefly about the onus of new strict EU regulations governing data use and how to overcome them.
EPISODE 4 – The Importance and Impact of NLP in Insurance
Guest: Gero Gunkel, COO, Zurich Customer Active Management (ZCAM), Zurich Insurance
Gero Gunkel of Zurich Insurance talks about the great and wide impact of NLP in the insurance space via a couple of use cases.
NLP is used in process automation to read, process, and analyze text documents. An emerging use case for NLP is serving as a sort of “digital analyst,” using AI to scan the document and actually write up a document summary.
Gero discusses the importance of the latter use case in depth, as such functionality requires an intricate output produced by advanced, unsupervised AI in the form of a generative model. Gero also talks about the accessibility of NLP in insurance and the future of NLP applications in this space.
EPISODE 5 – Using Knowledge Graphs in Target Discovery
Guest: Krishna Bulusu, Director of Early Computational Oncology, AstraZeneca
A biologist turned director of a department at one of the largest drug discovery companies, Krishna Bulusu of AstraZeneca provides fascinating insights on how knowledge graphs are “waking up” latent data to form valuable insights.
Knowledge graphs are very useful in every stage of the drug discovery journey. This includes identifying and validating disease-relevant proteins at the first step: a process called target discovery. Knowledge graphs are particularly useful at this juncture because they provide a wonderful common platform for bringing together divergent data types and understanding their relationship.
A tool that is capable of potentially uncovering relationships in data where none previously existed or is hard to find becomes tremendously useful in the drug discovery process, given the enormous quality and complexity of data required.