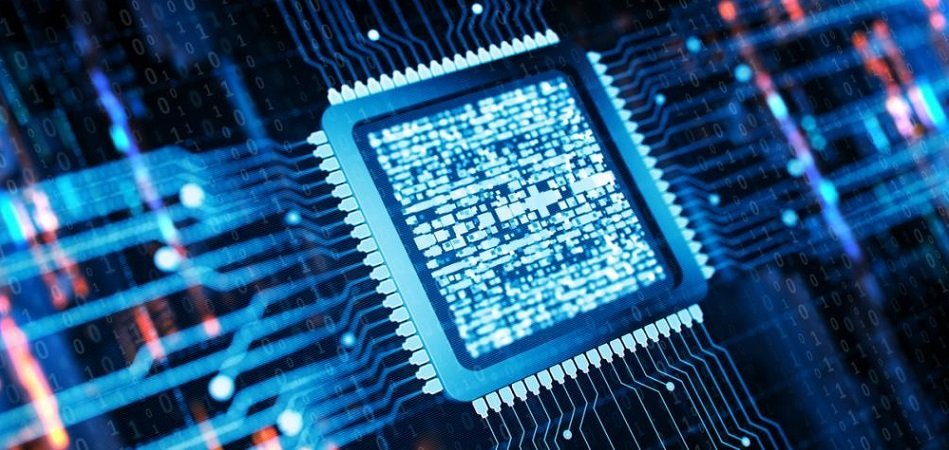
AI hardware is a fast-growing interest among tech media, and there is a lot of opportunity for computer hardware developers when it comes to building chipsets for AI. That said, margins for AI chipsets can differ wildly depending on the use-case for which they’re being built.
Despite this, enterprise customers looking for AI hardware are looking for more than just performance, and marketing certain aspects of the chipset and their support software may give some hardware startups an advantage over giants like Intel and Nvidia.
AI systems typically involve powerful computing requirements, far beyond what a regular laptop might be able to process. This is where graphics processing units (GPUs) commonly used to render video games seem to have emerged as accidental leaders in the AI space.
The type of computing required in AI projects is largely “parallelized,” and rendering video game graphics inherently requires a somewhat similar underlying processor that can execute parallelized mathematical computations.
Today, “GPU” is a commonly used term in AI circles. Large tech giants like Google, Amazon, and Microsoft have bought a large number of these GPUs to train their AI models.
Hardware for AI can be classified into two categories based on where the chipsets are being applied:
Training Operations in AI
Hardware for training operations in AI includes AI chipsets designed to help train AI models using massive datasets. The ideal objectives are to extract the maximum performance from a chipset and reduce the time taken to train the AI algorithm. “Hyperscale” training operations are at the moment only being applied by the large Fortune 500 tech giants that are trying to get their AI products out to market before their competitors.
Customers looking for hardware for training operations are looking at metrics of performance, power consumption, and cost in that order. Large hyperscalers are spending millions in this space, and the margins for manufacturers are fairly large.
Inference Operations in AI
Hardware for inference operations in AI includes AI chipsets that might power smartphones, intelligent cameras, robots, and autonomous vehicles. The objective of semiconductor fabricators with inference chips is to achieve decent performance at very low power consumption, low cost, and low physical space.
These chipsets have the potential to be more commoditized and are made for specific applications. The margins for manufacturers building inference chips is very low.
The State of AI Hardware Today
We spoke to Ed Nelson, Senior Conference Director of the AI Hardware Summit, an annual event taking place in Silicon Valley, September 17 – 18, 2019, about the AI hardware market today and how it might change in the next two years. According to Nelson:
The AI hardware market is so nascent at the moment. We are at the point where the market exists as a bunch. There are a few large semiconductor companies that have general purpose architecture GPUs that handle a lot of the AI workload today…Training operations in most AI projects today are run on Nvidia GPUs, while Inference operations are largely done on Intel CPUs. A lot of the high performance chipset startups either haven’t had customers yet, or are in pilots and have not been rolled out widely yet.
The AI hardware market is highly nascent, and although there are clearly large players, such as Nvidia and Intel, occupying a significant portion of the market, there has also been an influx of high-performance AI chip startups with their own products. That said, there seems to be no concrete evidence that products from these new AI hardware startups are significant improvements over those from Nvidia and Intel in terms of costs, performance, and flexibility.
This also shows how nascent the market actually is. The first wave of results from customers having used AI chipsets from startups will be coming out this and next year, which might give the market a clearer picture of whether the dominance of the large semiconductor manufacturers can be challenged.
Not Just Performance: Alternative Considerations for Purchasing AI GPUs
Chipset manufacturers large and small publicize and market the performance of their AI products. According to Nelson, however, for the largest customers of these chipsets, including server vendors and startups, performance is only one piece of what they’re actually looking for.
The factors that may play a larger role from the perspective of these buyers might be the cost-effectiveness, flexibility, and level of software support that comes along with a purchase. Companies like Nvidia have built reliable and mature software support ecosystems over the years that seem to be very important to buyers. Enterprise customers may be used to interacting with Nvidia software interfaces, for example; there may be a training cost associated with purchasing GPUs from another brand.
In addition, enterprise customers that use GPUs to build products have to consider how they’re going to price their products. Nelson gives the example of a self-driving car manufacturer. Some GPUs built for machine vision can cost over $10,000. The burden of that cost lies on the consumer, as a self-driving car equipped with six of these chipsets is already starting at $60,00.
Customers also want these chipsets to be resistant to the larger changes in the development of AI techniques. For example, the introduction of Generative Adversarial Networks (GANS) was a fairly major development, and GPUs built exclusively for GANs may be at a disadvantage when the next leap in AI techniques arrives.
AI model-specific chipsets might be less desirable because they could become obsolete before they enter the market if a better machine learning model is developed during that time. Reprogrammable architectures, such as FPGAs, are popular for this reason.
AI Hardware Giants Versus AI Chipset Startups
The debate around whether the dominance of the larger manufacturers will continue is still too early to settle. At the moment, some models might take months to fully train. One can expect that developments in this space are geared toward bringing that time down to the order of a few hours. The race among the larger companies to be the first product to market seems to be fueling the developments in AI hardware without any cost restraints.
The newer AI startups might likely focus on inference chipsets with Application Specific Integrated Circuits (ASICS) for AI inference applications that offer higher efficiency and lower costs than the larger manufacturers.
The smaller margins in this space might mean a difficult marketspace for these startups because the R&D budgets of the larger manufacturers are significantly larger. In addition, they are likely better equipped to handle the volatility of the market.
At the same time, there are still challenges for even companies like Nvidia to overcome. Nvidia seems relatively locked into their existing GPU architecture. Although there are a lot of common operations required in graphics rendering and AI, it is certainly not as simple as just using certain features of the GPU.
At the moment, customers of AI chips from Nvidia are also paying for some features of GPU that do not inherently complement AI processes. It might be too early to tell how this could impact the AI hardware market in the future.
Google is still buying large volumes of GPUs despite having their own GPU products. As such, one can expect that there will be a lot more development in AI hardware in the next two years. Although, at the moment, the market is too nascent and too focused on general purpose computing. In the future, however, the state of AI hardware will likely transition to domain-specific computing in the long term.
Meet Nelson and keynote speakers such as; John Hennessy, Alphabet Inc, Lip-Bu Tan, Cadence Design Systems, Naveen Rao, Intel AI and representatives from Synopsys, Flex Logix, Groq at the AI Hardware Summit on September 17 – 18, 2019.
Kisaco Research says the summit brings together the entire ecosystem, defining the AI hardware industry for the foreseeable future.
This article was sponsored by Kisaco Research, and was written, edited and published in alignment with our transparent Emerj sponsored content guidelines. Learn more about reaching our AI-focused executive audience on our Emerj advertising page.
Header Image Credit: Government CIO Media & Research