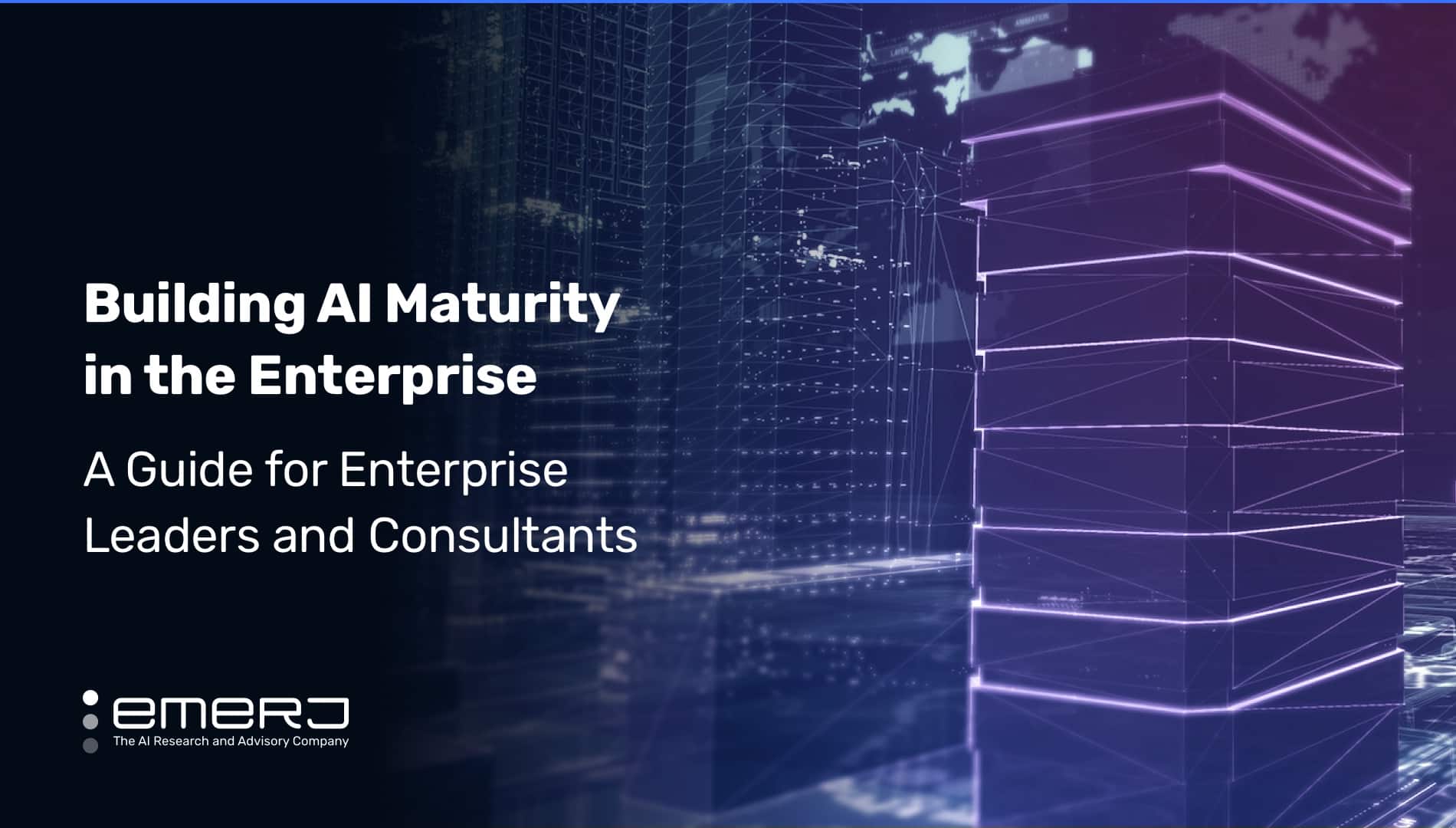
This article was a request from one of our Catalyst members. The Catalyst Advisory Program is an application-only coaching program for AI consultants and service providers. The program involves one-to-one advisory, weekly group Q-and-A with other Catalyst members, and a series of proprietary resources and frameworks to land more AI business, and deliver more value with AI projects. Learn more at emerj.com/catalyst.
As an AI project leader, you need to focus not only on delivering measurable near-term value through your AI projects, you also need to improve the AI maturity of the enterprise you're working with. Whether you're an internal enterprise innovation leader or an outside AI consultant, "plug and play" AI applications might be a useful place to start experimenting with AI, but they're not a path to genuine AI transformation and long-term advantage.
Smart project leaders determine where they can find near-term leverage, and they align those near-term projects with...
You've landed on exclusive content for Emerj Plus Members
Emerj Plus Membership
In-Depth Analysis
Consistent coverage of emerging AI capabilities across sectors.
Exclusive AI Capabilities Matrix
An explorable, visual map of AI applications across sectors.
Exclusive AI White Paper Library
Every Emerj online AI resource downloadable in one-click
Best Practices and executive guides
Generate AI ROI with frameworks and guides to AI application