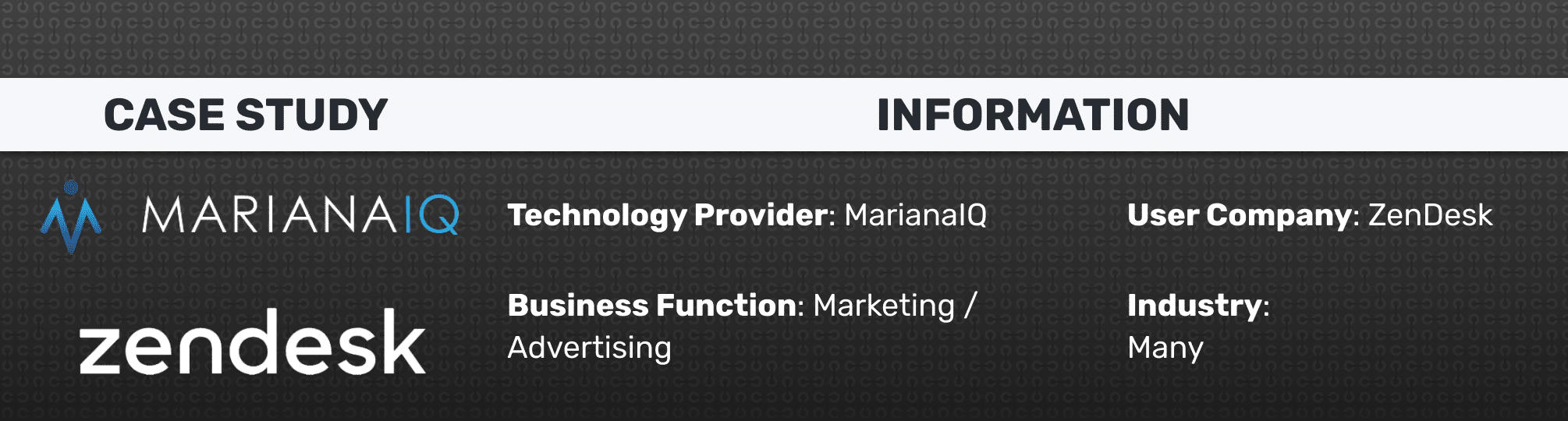
Technology Provider: MarianaIQ
User Company: ZenDesk
Industry: (Many)
Application: Marketing / Advertising
Problem
ZenDesk realized that it was having a problem with consistently generating ROI on pay-per-click (PPC) and search engine marketing (SEM, like Google AdWords). The company felt that it’s target audiences were often too broad to convert well, resulting in higher cost to acquire a customer through PPC and SEM channels. The company was basing much of it’s ad spend around marketing personas that it believed to be accurate.
Actions Taken
Zendesk uploaded their historical contacts to the MarianaIQ UI, MarianaIQ appended data and created persona profiles from those. For each campaign, Zendesk chooses account and persona criteria on the UI, MarianaIQ then finds the matching audience and shares with Zendesk to target on Facebook campaigns.
Results
Paul Godfrey, Director of Engineering at ZenDesk, claims a marked rise in lead quality after implementing MarianaIQ. “We’ve effectively been able to increase lead volume by 4X” (compared to their previous content marketing efforts), says Paul. ZenDesk highlights a direct correlation between quality of a target audience and lead cost, with more tightly segmented audiences from MarianaIQ driving more results by virtue of driving down cost-per-lead.
Transferable Lessons
In an ideal world, dialing in target audiences for advertising is about more than keywords and geo locations, and tools that provide more specific audiences can aide in improving ROI per advertising dollar. One of the primary promises of machine learning lies in recognizing matters in data that humans would be unable to detect. Large ad networks allow for this already.
For example, Facebook allows advertisers to upload an email list of customers in order to target a “look-alike” audience. Facebook finds the profiles tied to the uploaded email lists, and finds patterns in the behaviors, likes, dislikes, and commonalities among those profiles. These patterns can be mapped across Facebook’s wide inventory of accounts, giving an advertiser a broader reach to similar people on Facebook. This simply wouldn’t be possible without machine learning – and one can suppose that these segmentation technologies won’t exclusively remain the purview of digital giants like Facebook.