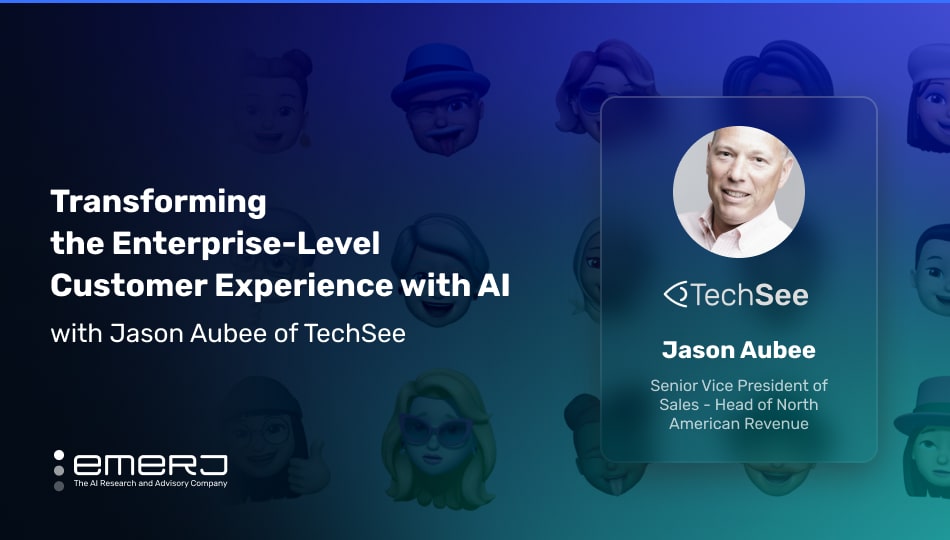
Contact centers play a crucial role in delivering exceptional customer experiences (CX) across industries, both B2c and B2b. As customer expectations elevate, organizations increasingly leverage AI to streamline operations and enhance customer service. According to recent reporting in the MIT Technology Review, enterprises generally deploy AI to transform contact centers, usually taking the form of intelligent virtual assistants to obtain predictive customer insights as well as for dispute and fraud management.
Additionally, Deloitte reports that contact centers face pressures from rising volumes, changing consumer preferences, and economic uncertainty – making investments in AI and automation critical. However, the potential for complete optimization in these business functions remains to be explored, given that the majority of contact centers and many CX depend solely on audio as a means of communication.
Now that nearly all mobile phones have camera and video capabilities – and enterprises have the data infrastructure to adequately capture visual data – the possibility of integrating visual CX data into AI-powered contact center solutions has enormous potential to revolutionize customer interactions on top of the enormous gains already brought on by machine learning and newer generative applications of the technology .
Emerj Senior Editor Matthew DeMello recently spoke with Jason Aubee, Senior Vice President of Sales and Head of North American Revenue at TechSee on the ‘AI in Business’ podcast to discuss how integrating visual data in contact centers will come to represent a step-level improvement in B2b CX workflows.
In the following analysis, we examine three critical insights from their conversation:
- Enhancing understanding with visual communication: Incorporating visual elements into customer interactions to expedite issue resolution and improve comprehension.
- Implementing dynamic AI interactions: Utilizing advanced conversational AI with large language models to create flexible, adaptive customer interactions that go beyond scripted workflows to address customer needs with agility.
- Optimizing customer interaction with sentiment analysis: Leveraging ‘episodic memory’ to analyze sentiment and success metrics from previous interactions that continually refine and improve the knowledge engine, ensuring responses are aligned with successful outcomes and evolving customer expectations.
Listen to the full episode below:
Guest: Jason Aubee, Senior Vice President of Sales, Head of North American Revenue, TechSee
Expertise: Business development, Marketing Strategy, Direct Sales
Brief Recognition: Jason Aubee is the Senior Vice President for Sales at TechSee. He has previously worked with NICE, CafeX Communications, and Carousel Industries. He studied Aerospace, Aeronautical, and Astronautical Engineering at the University of Southern California.
Enhancing Understanding with Visual Communication
Jason opens the discussion by talking about the limitations of traditional contact center strategies, particularly in terms of communication channels and sensory modalities. He begins by emphasizing the narrow focus of current approaches that rely solely on audio-based communications that, at best, may transition to text-based interactions, which restrict the ability to gather information effectively.
He argues that humans don’t experience the world with just one sense and emphasizes the importance of expanding the media formats in which contact centers can collect information. By introducing visual data and sensors into the conversation, Jason suggests that the contact center can broaden its understanding and address issues more efficiently. He uses the analogy of a picture being worth a thousand words, stating that in the context of their work, a picture (or at least some kind of visual information) can save considerable time compared to verbal descriptions.
“That’s kind of like a little idealism; everyone uses [the phrase,] ‘a picture worth 1,000 words.’ Well, in context in our time, it’s 1000 words in seven minutes,” Jason tells the executive podcast audience.
He further illustrates his point, particularly denoting the impact visual data streams will have on B2b and service workflows, with a personal anecdote about troubleshooting a Wi-Fi issue with a customer whose son called the company on her behalf. Despite the son’s initial questions, it took considerable time to identify the root cause of the problem because of the limitations of verbal communication. However, by widening the perspective and considering visual cues, such as checking if cables were adequately plugged in, the issue was resolved more quickly.
Jason further elaborates on the concept of modality within the context of omnichannel communication in contact centers. He explains that while omnichannel typically involves various text-based communication channels like chat, SMS, email, and social media, modality goes further by increasing the number of senses involved in communication.
He emphasizes that by incorporating multiple senses, such as hearing, seeing, and reading, contact centers can deliver information more effectively to both agents and customers. For instance, instead of verbally describing a solution, agents can send visual content like animated pictures to convey instructions more efficiently.
Moreover, Jason introduces the concept of bi-directional notation, where visuals can be exchanged between agents and customers, allowing for more accessible communication and understanding. Developing such an approach could involve actions like circling or pointing to specific elements within visuals to direct attention.
Implementing Dynamic AI Interactions
Jason then discusses the future of contact center automation and the role of advanced technologies such as metahumans, avatars, and more intelligent bots. He explains that the traditional scripted workflows of conversational AI are evolving with the introduction of large language models, moving away from rigid “if-then” concepts towards more dynamic and flexible interactions.
He describes an example scenario where a customer interacts with an interactive avatar named Sophie, powered by a large language model and multi-modality capabilities. Sophie engages the customer in a natural conversation, guiding them through setting up a new device step by step.
Most importantly, Jason highlights that pre-scripted workflows don’t constrain this interaction; instead, they adapt dynamically to the customer’s needs and questions, even extending to upselling additional products or services seamlessly. He exemplifies this point in an anecdote describing the entire workflow in which a customer, described as a ‘home user,’ orders a brand new device from the TechSee website and calls their contact center to troubleshoot its use:
“So he basically says, ‘I opened this, and I don’t know how to use it,’ as the conversation starts. [The Sophie avatar] shows him how to take it out of the box, put it in the filters, get it connected, get it started, and in a very fluid way, transitions to, ‘By the way, now that is working. I don’t see it on my Google Home network.’ That is not a scripted workflow in today’s conversational AI; that is not even a scripted workflow that you give an agent because that starts a brand new conversation of, ‘Hey, we got you working. Now you want to know how to attach it to something we don’t even offer?’
[Sophie] says, ‘Great! I know how to do Google Home. Let me see what you’re looking at.’ [She] sees the screen, identifies which button to use, how to attach it, how to link it… Then the end user just clicks through the process in Google’s world – not even the end product manufacturers world – and then we hand-off to an upsell at the end.'”
– Jason Aubee, Senior Vice President of Sales, Head of North American Revenue at TechSee
Jason emphasizes that, unlike human agents who may be hesitant to engage in upselling for fear of upsetting the customer, AI-driven systems like Sophie can handle such interactions naturally and without reservation.
He notes that deploying AI systems can provide a smoother customer experience where upselling opportunities can be presented in a more accessible, less intrusive, and conversational manner, ultimately enhancing customer satisfaction while increasing revenue.
Optimizing Customer Interaction with Sentiment Analysis
Jason goes on to explain the core principles behind the cognitive engine they’ve developed for their service delivery. He breaks it down into two types of memory, semantic and episodic memory:
- Semantic memory refers to the ability to recall facts, concepts, and numbers. In a call center context, semantic memory would include information from manuals, FAQs, training materials, and other documented knowledge necessary for handling customer queries efficiently.
- Episodic memory, on the other hand, involves learning, storing, and recalling information from experience. In the context of a call center, this represents applied content and tribal knowledge—the insights gained from previous interactions and experiences.
Jason provides an example to illustrate the difference between semantic and episodic memory. Semantic memory would dictate that a particular alarm means a battery replacement, along with specific details about the type of battery needed. Episodic memory, informed by machine learning from past interactions, might suggest additional steps, such as providing the exact battery to the customer, along with instructions on how to replace it based on previous successful resolutions of similar issues.
He further discusses how they utilize sentiment analysis and success metrics such as NPS (Net Promoter Score) or survey scores to enhance their knowledge engine and improve customer interactions.
By analyzing the sentiment and success metrics from previous calls, they can identify patterns of successful interactions and feed this information back into their knowledge engine. This process allows them to optimize their responses and adapt to different customer scenarios more effectively.
‘”So we take all of your intrinsic interaction data – that is an absolute goldmine that a lot of customers don’t use – to say, ‘Hey, we’ve done this 100,000 times. What was the best outcome, what was the worst outcome, and how do we look for the causes?’ That’s what we’ve been doing forever with analytics. What we’re saying is, ‘Only give us the best. We’ll teach this system to only give the best results, and to do it in such a way that if the best results evolves, so does the response.’ You don’t have to recode it, you don’t have to do it. It learns dynamically through the interactive process.”
– Jason Aubee, Senior Vice President of Sales, Head of North American Revenue at TechSee
Jason, in the end, discusses the evolving expectations of their customers and their approach to providing customer service. He explains that they don’t limit their customers’ thinking when it comes to utilizing their capabilities. While many players in services and call center spaces initially focused on AI generative capabilities to standardize customer interactions, they’ve found that their customers are continually expanding their expectations and desires.
Their challenge now lies in keeping up with their customer’s evolving needs and desires. Rather than just being a solution provider, customers want companies like TechSee to become brand ambassadors who can address a wide range of issues, even if they’re not directly related to their product or service. Jason gives an anecdote about Zappos, where a customer service representative went above and beyond by ordering pizza for a customer in need during a conversation about ordering shoes. Such a level of personalized and proactive assistance is something that only unassisted humans have traditionally been capable of.
He insists that TechSee’s goal is to provide a similar level of human-like interaction through their digital capabilities. They aim to offer conversational AI capable of dynamic responses that can pivot based on the shifting nature of the conversation, all while maintaining control over the brand message and delivering exceptional service. While customers understand that they’re interacting with digital systems, they still expect a level of service that feels both human and personalized.