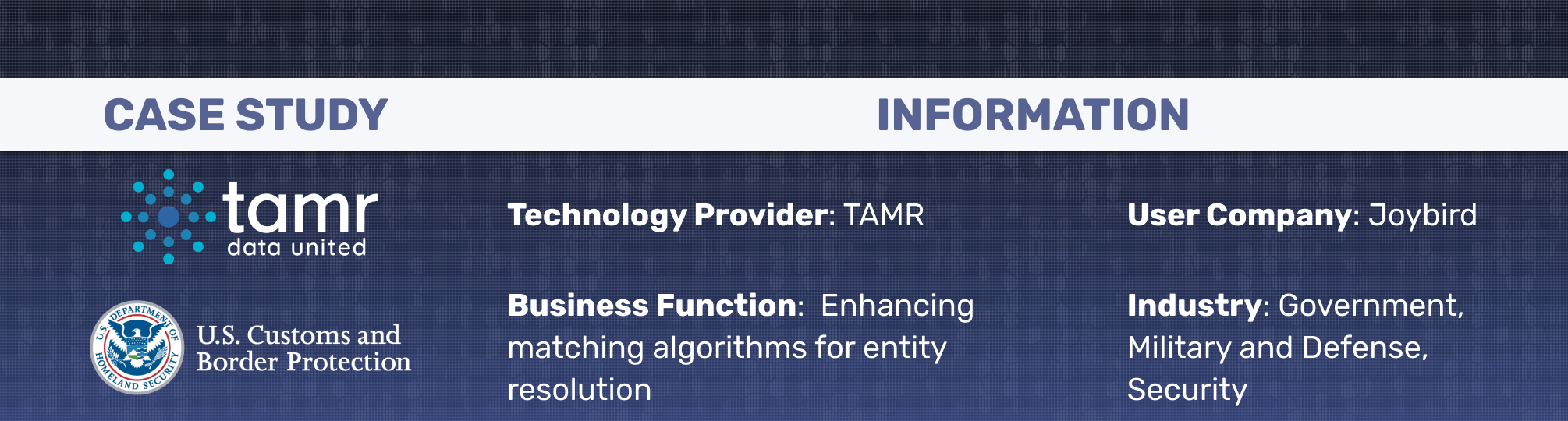
Tech Provider: TAMR – a company that automates the organization of a company’s enterprise-wide data through a machine learning-driven plus human-guided solution
Client: U.S. Customs and Border Protection (CBP), part of the United States Department of Homeland Security (DHS)
Industry: Government, Military and Defense, Security
Function: Enhancing matching algorithms for entity resolution
Tech Provider Contact Person: David Templeton
Problem
The U.S. Customs and Border Protection (CBP) agency was looking to develop and release the ‘Global Travel Assessment System (GTAS)‘ as a consequence of the UN Security Council Resolution 2178 on Foreign Terrorist Fighters. GTAS was designed by CBP to be an open source platform that can receive and store standard air traveler information (Advanced Passenger Information (API) and Passenger Name Record (PNR)) enabling real-time risk-modeling. Specifics on the details of CBPs requirements are listed below:
- Using biometric data available from API/PNR to match passengers against an existing database.
- Discrete passenger identity matching from API and PNR data.
- Using historical data to build personalized profiles for passengers over time
Actions Taken
According to Ari Schuler, Director of Analytics Integration at U.S. Customs and Border Protection:
“We wanted to expand our ecosystem particularly in the areas of visualization, predictive models and entity resolution”
The CBP collaborated with Tamr to enhance the entity identification and matching algorithms for GTAS. Michael Gormley, federal team lead, Tamr claims that the company used human-guided machine learning to address CBPs requirements in the following steps:
- Tamr’s machine learning platform was developed to resolve passenger identity from massive datasets of API or PNR data
- The ‘training’ of the ML platform is aided by continuous input from region-specific subject matter experts in the travel domain
- The output is in the form of a decimal probability of match, which is used by human managers to make informed decisions.
We corresponded with David Templeton from Tamr’s communications team and he explained the features of the integration in more detail as follows:
“Tamr’s machine learning platform was implemented to resolve passenger identity from massive datasets of passenger (API) and reservation (PNR) data. Tamr was also used to compare the passenger data to a curated list of known individuals that is maintained by the government.
The ‘training’ of the ML platform can be aided by continuous input from region-specific subject matter experts in the travel domain. The system generates match probabilities from 0 to 1 that can be used by human managers to make informed decisions.”
He adds that during the human-guided ‘training’ process, which typically takes a day or two, users provide guidance by way of a UI that gives them specific yes-or-no questions. For this particular collaboration, Tamr provided a technical project lead and was closely supported by members of the CBP staff. Tamr also claims that their system can match most travelers in less than 5 seconds using the GTAS.
Results
At the time of writing, Tamr declined to share any metrics related to improvements achieved through AI in the collaboration with the CBP.
Transferable Lessons
The takeaway for business leaders here is that current AI is very capable in tasks involving matching or identification of an individual from existing structured massive datasets. What this essentially means is that in industries like in the insurance or healthcare industries, where there exists access to structured and curated public datasets, similar AI integrations are viable today.
For example, in the insurance industry, one transferable lesson here would be identification of new business opportunities by matching a pre-set profile with customer information databases. In the healthcare domain, possible diagnostics can be arrived at by matching symptom data to patient feature datasets or in identifying the at-risk individuals based on past historical records