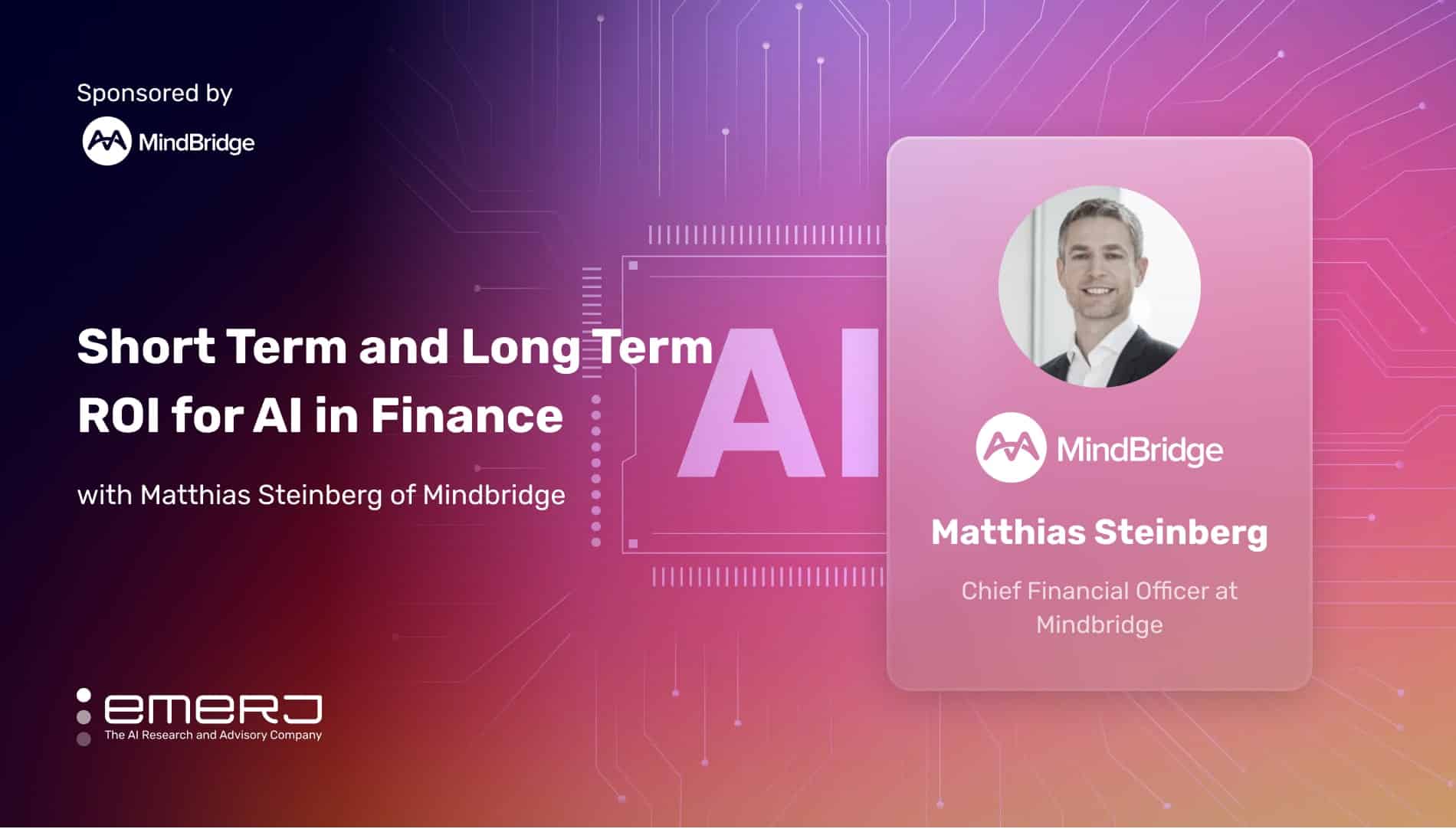
This interview analysis is sponsored by MindBridge and was written, edited, and published in alignment with our Emerj sponsored content guidelines. Learn more about our thought leadership and content creation services on our Emerj Media Services page.
In today’s financial landscape, the adoption of AI is proliferating, but this technological shift presents significant challenges for financial departments and workflows in every enterprise. A 2023 report by the Canadian Office of the Superintendent of Financial Institutions(OSFI) highlighted the office’s observation that financial institutions across the world are increasingly incorporating AI to optimize operations. Yet, many enterprises across industries need help with governance, data management, and ensuring explainability in their AI models.
Their guidelines on deploying these operations are drawn from executive surveys like that of the Institute of International Finance, which noted in 2020 that only 35% of global financial institutions claimed to have established a centralized specialist team, such as an AI Governance Council, that helps align the organization with data-based goals, assessing use case value, prioritization, and approving new AI models.
The game of catch-up makes for an uneven landscape of adoption across enterprise departments. It complicates effective AI integration in the long term, making it difficult to ensure transparency, explainability, and data management. The Superintendent’s report also emphasizes that, while many institutions are working to build AI governance frameworks, these frameworks often require significant cultural shifts within the organizations as more departments begin to use AI.
However, understanding how AI delivers ROI is vital for decision-makers to scale these systems across the enterprise effectively. On a recent episode of the ‘AI in Business’ podcast, Matthias Steinberg of MindBridge offers a vision for AI adoption for financial teams that maximizes short- and long-term returns.
Matthias advises financial leaders that the best way to scale AI in financial teams and workflows is to ensure that deterministic, first-generation AI capabilities can embed themselves in predictable systems, effectively laying the groundwork for scaling up to new generative AI (GenAI) capabilities later. In the process, he identifies key use cases that help navigate specific scaling challenges for extracting accurate, measurable, and long-term value from AI in the enterprise.
In the following subsections, we break down two actionable insights from Steinberg’s conversation on the podcast with Emerj Senior Editor Matthew DeMello about tracking ROI from both short- and long-term use cases:
- Delivering quick wins with deterministic AI use cases first: Laying the groundwork for further GenAI adoption by targeting high-frequency processes first — typically in repetitive workflows and detecting anomalies in financial data — for training machine learning models so their insights can enhance long-term automation systems as they scale through the enterprise.
- Ensuring long-term AI scalability and value: Investing in agility for AI frameworks through effective change management and preparing teams for higher order workflows as data governance, explainability, and regulatory standards evolve.
Listen to the full episode below:
Guest: Matthias Steinberg, Chief Strategy Officer at MindBridge
Expertise: Corporate Finances, Automation, Long-Term Strategic Planning
Brief Recognition: Matthias Steinberg is a leading voice in AI-driven financial transformation, serving as Chief Strategy Officer at MindBridge. His career spans leadership roles at top financial institutions, where he spearheaded the adoption of AI-driven solutions to enhance audit quality, streamline decision-making, and optimize business operations. Prior to joining MindBridge, Steinberg held executive positions at global firms such as Ernst & Young and KPMG, where he led initiatives in financial auditing and risk management. His in-depth expertise has been instrumental in helping organizations leverage AI to solve complex financial challenges, making him a recognized thought leader in the field.
Delivering Quick Wins with Deterministic AI First
Steinberg opens the conversation by emphasizing that the ability to automate processes and streamline operations are becoming critical enterprise capabilities in financial workflows across industrial sectors. He mentions a “tension” between financial teams’ desire for repeatable results, and the more probabilistic nature of GenAI systems, now at the peak of their hype cycle.
Inherent in that “tension” are escalating challenges corporate finance teams face in managing large amounts of data in a time-efficient manner, an essential discipline for the enterprise deployment of AI long-term. These market forces drive enterprises to adopt AI first because of the technology’s ability to process and analyze vast datasets quickly and with higher accuracy than manual processes, which provides organizations with immediate operational benefits.
While some versions of GenAI are applicable to financial workflows, Matthias makes a widely-noticed exception that, because these systems don’t handle numbers very well, GenAI is not advised as a front-door use case for many financial workflows.
Deploying first-generation deterministic AI technologies like machine learning for analyzing enterprise data creates a cycle unique to the beginning of the enterprise AI adoption process: leveraging rules-based, efficiency-related use cases first to expedite the adoption of AI through the enterprise later. Steinberg goes on to explain that, in understanding what kinds of AI need to be deployed first to scale effectively through the enterprise with financial workflows as a beachhead, short-term ROI is often realized through deploying intelligent document processing to automate routine accounting tasks such as:
- Invoice processing
- Financial reporting
- And data reconciliation
By leveraging AI for anomaly detection, finance teams can identify suspicious transactions or irregularities much faster than manual methods. These use cases include fraud detection and span anti-money laundering and know your customer (AML and KYC) efficiencies as well.
According to Steinberg, early detection mitigates potential fraud risks and prevents large-scale financial losses — the features of a textbook short-term use case. Recent research from Cambridge University’s Data & Policy Journal also notes AI systems have enabled companies to respond to fraud across a growing digital payment ecosystem, detecting fraud attempts with increased sophistication.
These AI-driven efficiencies lead to cost savings and allow finance professionals to focus on higher-order strategic decision-making. He also underscores that industries adopting AI first for anomaly identification, in particular, are seeing rapid, tangible results within the first year of deployment.
Steinberg underlines that what is often forgotten about the nature of these workflows is their near-instant payoff, which helps financial institutions identify suspicious transactions and prevent fraudulent activities before they cause significant damage.According to a report from Deloitte, AI-enabled fraud detection systems reduced fraudulent activity by up to 37% in organizations that adopted the technology. These more deterministic, anomaly detection-related efficiencies allow businesses to secure quick wins while continuing to scale AI applications over time.
However, underneath these benefits are the measurable long-term value drivers that business leaders should focus on for measuring ROI in these systems to help them scale through the enterprise. First among these longer benefits that Steinberg mentions is that their inherent data exhaust is perfect for feeding machine learning models to extract greater efficiencies and insights from essential enterprise data. He draws particular attention to the fact that AI’s ability to analyze massive amounts of transactional data in real-time makes it far more effective than other traditional rule-based systems.
Steinberg then touches on how these use cases also offer other, less traceable long-term benefits like boosting customer confidence. By protecting their clients from fraud and demonstrating a commitment to security, financial institutions also foster greater trust and loyalty among their customers. These proven gains, in turn, enhance the institution’s reputation and competitive positioning in the market.
Throughout this portion of the discussion, he stresses that — whether in anomaly detection use cases or elsewhere — organizations focused on effective scaling of these systems should, as a general rule, begin their AI adoption journey by first identifying processes that are manual and time-intensive, as these are the low-hanging fruits of simple application of these use cases in deterministic technologies, often taking the form of some automation.
Steinberg advises that, by first automating processes that are high-volume and repetitive, organizations can see immediate benefits. However, he laments that leaders are often too bearish on driving these capabilities through various enterprise functions “to the extent that they actually could for their benefit.”
Ensuring Long-Term Scalability and Value
While short-term ROI is crucial, Steinberg then offers a vision of AI’s long-term potential in corporate financial workflows that’s even more transformative in scope. He cautions executive listeners that AI adoption is a constant evolution, not a one-stop process. Deployed with more patience for ROI, even simple deterministic applications of AI enable predictive analytics, advanced financial modeling, and long-term risk assessment for financial teams. These functions generally center around allowing organizations to stay ahead of market trends and shifting economic landscapes.
The vision he offers here is of a truly revolutionized enterprise management system where these functions are updated in real-time for business leaders in a way that will feel and look like “a daily view of the integrity and risk in all of their relevant financial flows.” He compares that financial tools at such a point of development will look much like how cybersecurity risk tools appear today.
However, Steinberg stresses that to be successful in building such a real-time financial operations dashboard, AI must be embedded closer to the core functions of the enterprise to unlock the benefits from these longer-term use cases. That scale of embedding means integrating both capabilities and insights from AI systems into strategic planning, compliance, and investment analysis in turn.
A potent challenge to the long-term scaling of AI in the enterprise that Steinberg highlights in the context of use cases is the fear of job displacement that often accompanies AI adoption. While automation and AI can indeed optimize tasks that previously required manual labor, there is an ongoing debate about how these technologies will affect employment in the finance industry.
Steinberg advises leaders to focus on scaling a sense of responsibility and purpose in hand with increased automation. Rather than eliminating jobs, AI in finance can augment human work by taking over routine, repetitive tasks, allowing professionals to focus on higher-value activities such as strategic decision-making and client engagement.
Where we see finance teams and their work start to blur with teams in cybersecurity and procurement, it’ll be all the more important to keep employees focused on the tasks that contribute directly to enterprise value — no matter how often those tasks and business goals may change. Elsewhere, other high-value activities to be expected from core corporate teams leveraging automation will include anticipating changes in workflow based on new data processes and new expectations from customers.
Steinberg emphasizes that using that argument to demonstrate the agility necessary to scale AI across different functions within an organization is key to unlocking long-term ROI. As AI models learn from more data, they become more accurate and offer deeper insights over time, particularly in long-term use cases like investment analysis and financial forecasting.
These insights can often mean drastic changes in the day-to-day of any truly data-driven organization, changes that will mean incredible support, resources, and planning from division leaders. Such planning is often described as change management. In touching on change management as an enterprise discipline, Steinberg tells Emerj’s executive audience that associated stresses will be acutely felt by finance teams.
“It makes sense to allocate time and some resources to be on the forefront of what’s happening,” he says. “You want to make sure, as a finance team, you’re always working on initiatives that test AI tools for your processes and for your finance department.”
The reason for these stresses is that the rewards could not be more promising. A recent McKinsey study found that companies leveraging AI at scale through their organizations can increase their cash flow by 120% within five years.
Confronted with these and other scaling trends and challenges, Steinberg stresses that the businesses that integrate AI into their long-term financial strategy and commit to continual refinement of that strategy will reap the most significant benefits. That elongated process of refinement will mean aligning AI with governance protocols to ensure compliance and regularly training teams to maximize the technology’s value.