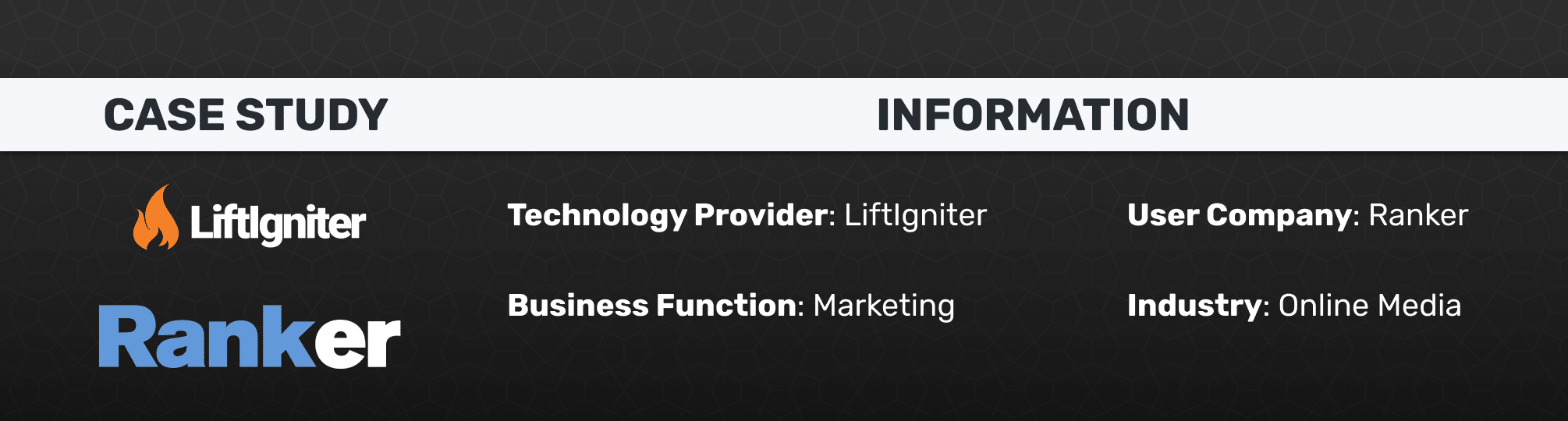
Tech Provider: LiftIgniter
Client Company: Ranker
Client Company Description: Ranker is an online destination for broad, opinion-based, crowdsourced rankings of everything.
Industry: Online Media
Function: Marketing
Problem
Ranker’s visitors participate in developing the lists by coming up with definitive rankings across various topics (from politics to film and beyond). The company faced the problem of knowing what content to serve to which particular user in order to improve engagement. With thousands of threads and topics, the tasks was immensely challenging. While the firm values the advertising revenue that can be generated from a specific session, it also
Actions Taken
Ranker began by implementing LiftIgniter into a single website “widget” or module (optimizing content in a specific section of the site). After testing, the firm implemented LiftIgniter throughout the site, across mobile, desktop, and video content.
LiftIgniter is integrated with a javascript beacon. Via the beacon, LiftIgniter claims to collect all the information needed to deliver their service:
a) User related information and signals, and
b) Data about the “content” itself (articles, videos, images, items to buy, ads).
LiftIgniter’s machine learning models begin building out correlations in real-time after the integration is complete. LiftIgniter claims that they are generally able to build relevant models within 7 days of a completed integration.
In the initial training period, Ranker started to making API calls to LiftIgniter’s servers for every impression on their site. The API returns a JSON array of a rank ordered list of the items to show to that individual user on the Ranker site.
LiftIgniter’s Adam Spector states: “That API to JSON process happens for every single click as our model learns and updates in real-time for that user and for all other users on the site.”
Results
Liftigniter powers Ranker’s related content modules. For example, Liftigniter found a connection between “Ranker’s Best Board Games” list and an “Anime on Netflix” page, whereas Ranker’s internal logic would have always shown a card games list alongside that page.
With LiftIgniter’s help, Ranker’s related content clicks increased by about 50% during their 7-day post-trial period, and over time with at least 75% after further optimizations. It wasn’t clear (or wasn’t included in the data we collected from LiftIgniter) how these metrics translated to other metrics like: Average time on page, average pageviews per user.
LiftIgniter claims that Ranker did a clear A/B test and quantified the LiftIgniter results and saw a significant ROI, which including a very low total cost of ownership (limited maintenance efforts, no need to add in extra tags, no need to build out a data science team).
LiftIgniter also claims that Ranker also implemented a number of business rules that are important to helping users navigate the site and make sure that certain types of content are shown to each user when and where it’s relevant.
LiftIgniter’s Adam Spector says that the company guarantees a 20% minimum improvement in related content clicks for it’s customers.
Transferable Lessons
Recommendation engines are more and more becoming a standard for eCommerce and media online. In the future, it’s likely that nearly all blogs or eCommerce stores will have some kind of real-time recommendation to “nudge” users towards the next conversion step (whether that be a first purchase, an email submission, an additional page view, etc).
It’s important to note that recommendation engines are predicated on data, and volume of user interactions. Web properties or apps with very few visitors or users are generally less likely to benefit from personalization than properties with more engagement. Over time, however, niche-specific personalization and recommendation engines may make personalization accessible to smaller sites – even if they don’t have enough data to train the system themselves.
For example, a personalization vendor (such as LiftIgnitor or a competitive firm) who works with many sports blogs may develop processes and systems for recommending sports content – and these “best practices” may be transferred to smaller sports blogs who don’t have very much of their own data to train the recommendation system with.
Such a “transfer” of learning will allow smaller firms to benefit from the technology – and also promotes a “winner-take-all” dynamic for media personalization, as the firms with the most robust and well-trained algorithms may have significant and lasting advantages over competitors. At present, we don’t see very much evidence for this “transfer” of recommendation in either eCommerce or media, but we suspect this will be somewhat inevitable in the coming years.