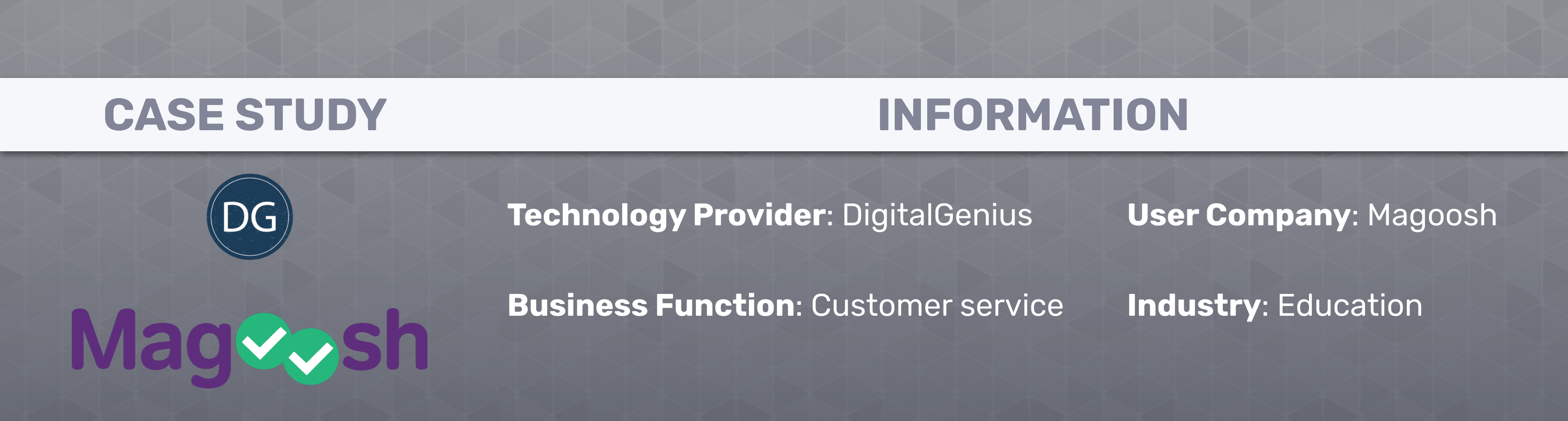
Technology Provider: DigitalGenius is an artificial intelligence solutions provider for customer service operations.
User Company: Magoosh provides online educational tools for students preparing for standardized tests, such as GRE, GMAT, etc.
Industry: Education
Function: Customer service
Problem
Magoosh’s support staff comprises two teams of 50 agents: A community support team for handling account inquiries, and remote tutors to handle in-depth questions for specific tests. Magoosh uses Zendesk to handle their customer support requests. It has over 900 macros on ZenDesk, which are pre-written, standard responses to common questions asked by the company’s customers.
The support staff found it difficult to search or discover these macros for offering timely customer help, which they believed to be negatively affecting their customer satisfaction scores on responses to questions about standardized tests. Part of this searchability problem was the enormous number of macros which took a lot of time to search through and manage.
Actions Taken
The DigitalGenius AI Platform was integrated with Magoosh’s Zendesk console. DigitalGenius trained a deep neural network to analyze incoming customer inquiries based on historical customer logs – learning how Magoosh’s support staff replied to various incoming inquiries.
DigitalGenius then automatically suggested the most relevant macros for new customer inquiries so the support team member does not spend time searching for macros or manually composing new responses to common customer inquiries. DigitalGenius claims that its AI Platform achieves this automatic macro suggestion by using deep learning models to extract the meaning and context of incoming inquiries and predicting the expected response. In addition, the platform has a historical response search feature, which the support staff can access.
When asked about this historical response search feature, …. Of DigitalGenius told us:
“The historical responses feature looked for historical tickets in which customers asked similar questions to the one agents were working on. We built the search ourselves, using our own search algorithms. And beyond that, we have a different UI to the Zendesk search, including the ability for historical response searches to take place in the app sidebar, so agents don’t have to navigate away from the page.
The coolest feature is that we prioritized historical tickets that had the highest CSAT. When agents searched from historical responses, we displayed to them whether that ticket got a high or low CSAT rating….so we think our feature promoted the best answers.”
The platform also reportedly predicts the relevant metadata about the case, such as tags, inquiry type, priority and other case details. With this information, it is able to analyze and route cases to the appropriate team. For example, if the incoming query is an account inquiry, the platform routes the request to the community support team, and for in-depth educational queries, it routes the requests to remote tutors – eliminating the need for a “human filter” to handle all tickets.
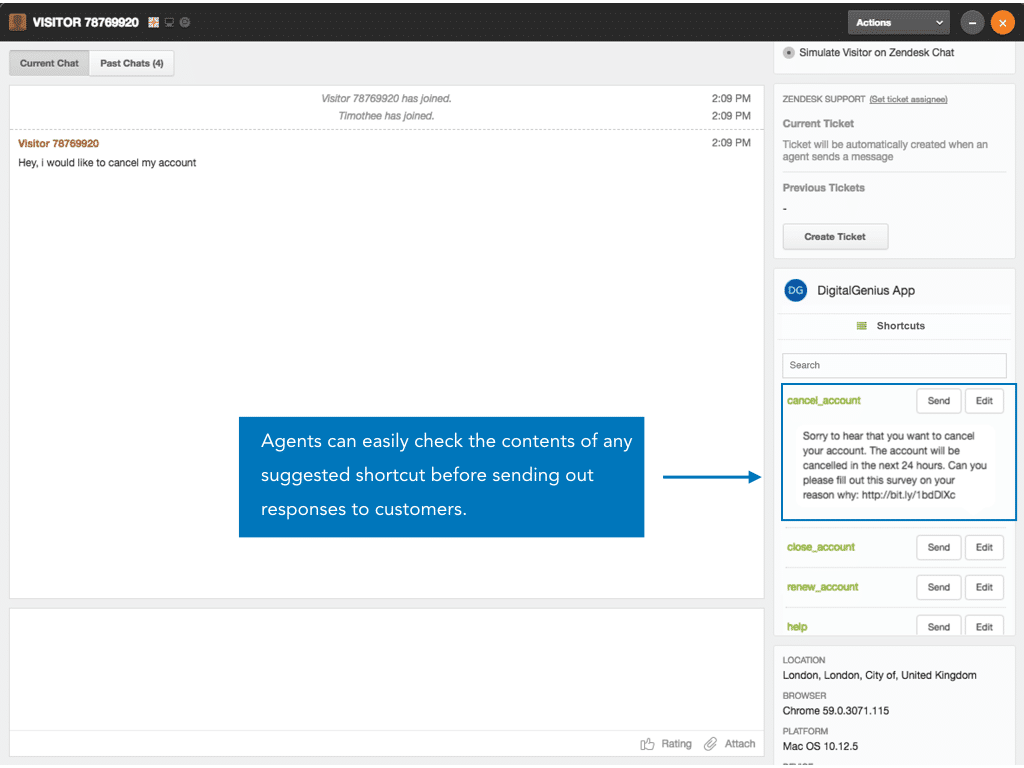
Results
According to DigitalGenius, about 83% of all customer tickets are supported by the Digital Genius platform integrated with Magoosh’s Zendesk. The company also claims a 92% accuracy in case tag predictions (tags are used within Zendesk for case categorization – for example, “refunds” might be a tag for that particular kind of customer issue). This improvement happened over an initial 6-month period with Magoosh, which Juan describes as a “learning segment” – stating that new and updated projects and underway with Magoosh now.
Asked for clarification on what it means to have 83% of messages “supported” by DigitalGenius, Juan replied:
“Supported in this context means that DigitalGenius AI has assisted Magoosh with 83% of their tickets – whether this is classifying them, suggesting the right macro or automating a response. The remaining 17% escaped our current AI capabilities, and had be dealt with manually in order to provide the best possible answer and avoid a potential wrong answer to a customer.”