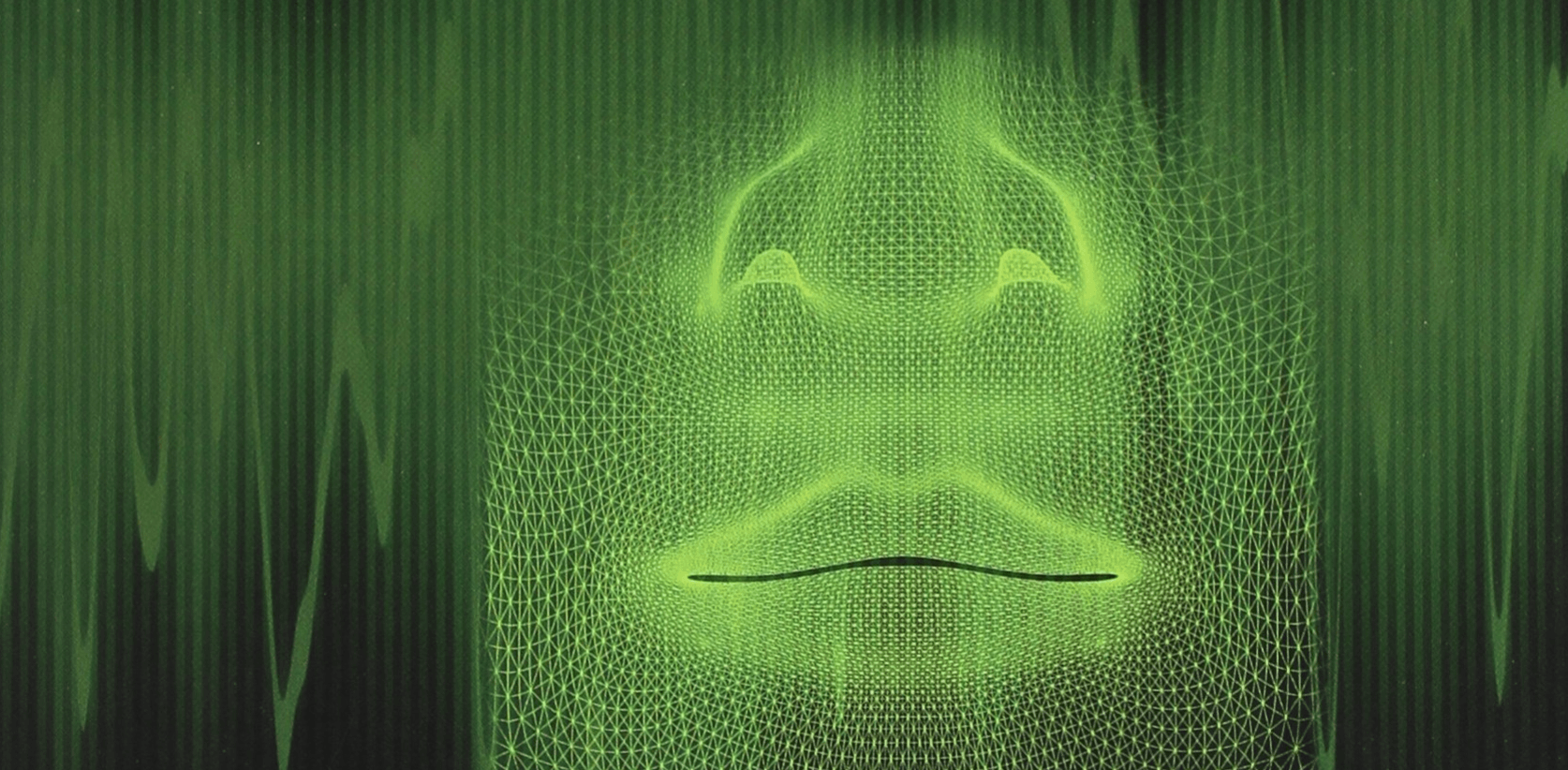
A 2017 Tractica report on the natural language processing (NLP) market estimates the total NLP software, hardware, and services market opportunity to be around $22.3 billion by 2025. The report also forecasts that NLP software solutions leveraging AI will see a market growth from $136 million in 2016 to $5.4 billion by 2025.
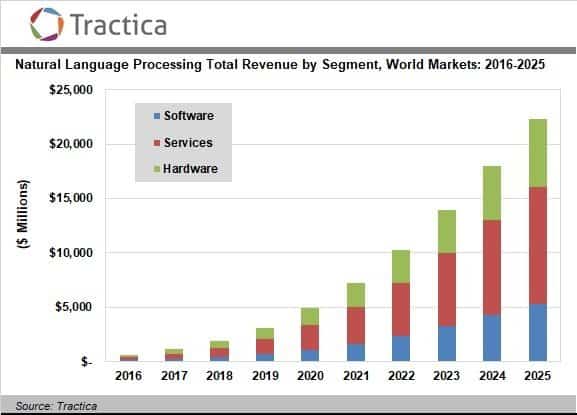
In order to shed more light on the growing applications of NLP solutions, Dan Faggella, the CEO of Emerj, converses with Vlad Sejnoha, the CTO of Nuance Communications, an organization offering AI and NLP solutions in voice, natural language understanding, reasoning and systems integration.
Vlad Sejnoha has been the Senior Vice President and CTO at Nuance since 2001. He holds a Masters degree in Electrical Engineering from McGill University. Vlad has been working in the field of NLP and speech recognition for over 30 years and holds 22 patents to date. Vlad also heads the company’s external research relationships, including Nuance’s five-year collaboration with IBM Research.
In this podcast interview, Vlad shares his insights with Dan on the current applications and future possibilities of NLP, particularly across the banking, healthcare, automotive, and customer service.
Subscribe to our AI in Industry Podcast with your favorite podcast service:
Current Applications of Natural Language Processing
Customer Service
Vlad says that some of the current virtual assistance solutions using NLP serve as intelligence augmentation.
Ideally, in such applications, a customer’s first request is intercepted by the AI, such as Nuance’s virtual assistant Nina. For example, a banking customer service system integrated with Nina uses the AI to answer some of the basic transactional queries such as opening an account or figuring out the best account type for a customer. For more complex queries, Nina redirects the customer to a helpline number or the appropriate landing page.
The video below describes some use cases of Nina’s assistance with banking.
A Nuance–Swedbank case study depicts the real-world application of Nina. The case study estimates that, by 2018, Swedbank customers’ primary choice of contact will be digital channels, such as the website help chat bot, emails, and social media. Therefore, the bank wanted to ensure that all customer queries could be handled via self-service on said digital channels so that the contact center agents could focus on sales rather than fielding simple but repetitive customer service inquiries.
The case study claims that 58% of Swedbank’s customer base was banking digitally, such as through Swedbank’s Mobile Bank. The bank’s 700 contact center agents were handling 3.6 million customer interactions per year, which included more than two million basic transactional queries, 500,000 emails, and 10,000 social media interactions.
The integration of Nina with Swedbank’s contact centers allowed its customers to search for information and answer basic transactional questions for themselves. The case study cites that the bank’s customers can ask freeform questions that Nina—accessible via a text box on Swedbank’s homepage—answers in a conversational tone.
For example, if a customer wants to order foreign currency, Nina either redirects the customer to a relevant web page or branch office, or asks clarifying questions such as which country’s currency the customer wants to order. Apart from being the customers’ first point of contact, Nina also reportedly helps the bank’s contact center agents with quick information searches for answering customer queries.
The case study claims that Nina handled over 30,000 conversations per month with a 78% “first-contact resolution” within its first three months of deployment. About 55% of these conversations did not require the customers to take further actions, such as calling the contact centers. According to the case study, Nina can now handle 350 customer questions and answers.
In the below video, Martin Kedback, the then-Head of Business and Development at Swedbank (currently acting as the Chief Product Owner), describes in detail the positive business impacts of Swedbank’s partnership with Nuance’s Nina.
Another existing application of Nina is its integration with Coca-Cola’s customer service department. In the video below, Michael Bowers, Director of Contact Center Operations at Coca-Cola in Atlanta, shares his views and business impacts of Nina on Coca-Cola.
According to the video, Nina was integrated at two places with Coca-Cola: on the My Coke Rewards and Ask Coca-Cola pages. Nina reportedly had 15,000 and 30,000 conversations a month on these pages respectively. Robert Weideman, Executive Vice President and General Manager for the Enterpirse division at Nuance, claims in the video that Coca-Cola saw a 40% reduction in voice calls after its integration with Nina.
Below is another demo video of Nina personalized as Chip for Coca-Cola conversing with a customer on the My Coke Rewards page:
Automotive
Nuance offers automotive virtual assistants, connected to major automotive OEMs like BMW, Audi and others.
One press release on Nuance’s partnership with BMW describes this connected car technology, the Dragon Drive AI, as “hybrid voice and natural language technologies derived from deep neural networks.” According to the press release, this Dragon Drive AI is essentially a conversational AI that is powered by Nuance’s hybrid embedded-cloud voice recognition, natural language understanding (NLU), and text-to-speech solutions.
The press release also states that the Dragon Drive AI enables drivers to access apps and services through voice commands, such as navigation, music, message dictation, calendar, weather, social media. For example, it is possible to command the AI to send a text message right from the car, like “Text Roger I will be 15 minutes late to today’s meeting,” or “Get me directions to Armando’s Pizza in Cambridge.” This technology supports 29 languages, including English, French, Italian, German, Spanish, Mandarin, Cantonese, Portuguese, Japanese, Korean, Arabic and more.
The below two videos demonstrate Nuance’s Dragon Drive connected car AI technology in the BMW 7 series.
Vlad elaborates that Nuance achieves accuracy in speech recognition by using multiple speakers and microphones that “create beams steerable by software that targets you [the driver] vs. others,” adding that Nuance’s technology produces “accurate transcripts from noisy environment in car cockpits.”
One of the major considerations of this connected vehicle technology, Vlad says, is the interoperability between different AI systems.
According to Vlad, there are two hard problems to solve in this scenario: identifying the right software to delegate commands to and communicating to the system in the language it understands. Nuance builds semantic automotive APIs that interact/interoperate with other software. These semantic APIs have a list of available services/software that can be registered with Nuance to help them understand the actions and languages of the registered software for interoperability.
In the past we’ve covered AI assistant applications in banking, airlines, recruiting, and more – and it be interesting to see if more automotive use-cases emerge in the years ahead as Vlad seems to predict.
Healthcare
Nuance provides various NLP solutions for the healthcare domain, including computer-assisted physician documentation (CAPD) and clinical document improvement (CDI) solutions. Physician documentation is part of medical records that contain patient clinical status, such as improvements or declines in patient health. CDI is the process of improving such healthcare records to ensure improved patient outcomes, data quality and accurate reimbursement.
This Nuance–United Health Service (UHS) case study summarizes an existing application of Nuance’s healthcare AI solution, Dragon Medical One. UHS wanted an advanced documentation capture tool to enable quick documentation of the patient story in real-time—one that could also be integrated with the electronic health record (EHR).
According to the case study, Dragon Medical One enables physicians to dictate progress notes, history of present illness, etc. and plan further actions directly into their EHR. Nuance CAPD reportedly offers physicians real-time intelligence by automatically prompting them with clarifying questions while they are documenting. However, to minimize obstruction during caregiving, Dragon Medical One asks clarifying questions in specific circumstances, such as possibilities of different diagnosis or a different piece of medical information that the physician should consider.
The case study reports that “UHS realized a 12% increase in case mix index (CMI) across the cases where physicians agreed with the CAPD clarifications, and updated their patient’s documentation accordingly.” UHS also reportedly achieved a 69% reduction in transcription costs year-over-year, resulting in $3 million in actual savings.
Before delving into the future B2B possibilities with NLP, Vlad has some important advice for businesses contemplating NLP adoption.
Vlad’s Advice For Businesses Considering NLP Technology Integration
Vlad has three important points for businesses to consider before integrating existing NLP technologies.
- There are a lot of AI and NLP applications in the market. It is very important to choose the appropriate applications that attempt to solve business problems with sufficient technology and provide value in measurable ways.
- Businesses have to consider the overall organizational readiness for embedding new technologies in existing workflows.
- Finally, businesses must have adequate and relevant data for training machine learning algorithms for accurate outputs. Vlad is of the opinion that the most successful AI companies first get their data right.
Future Possibilities with NLP
In order to advance existing NLP technologies, Vlad thinks that the businesses today could either:
- Attempt to make AI more human like, which is a hard task now. (For example, making a virtual assistant more conversational) or
- Proliferate existing AI technologies. (For example, extending automatic image captioning to healthcare and other applications to get a better understanding of images)
Here are some of the future possibilities of NLP that Vlad discusses in our interview:
Healthcare
Vlad talks about Nuance’s vision for a “medical ambient intelligence” using NLP technologies in healthcare. It is not uncommon for medical personnel to pore over various sources trying to find the best viable treatment methods for a complex medical condition, variations of certain diseases, complicated surgeries, and so on.
Information discovery and retrieval: A plausible application of NLP technologies here could be real-time information discovery and retrieval. That is, the healthcare AI solution will be able to understand the medical terminology and retrieve relevant medical information from the most reputable sources in real time. “This does not mean that the AI is inventing treatments,” Vlad says, “but enabling easy access to the right data at the right time.”
Diagnostic assistance: Another near-term and practical NLP application in healthcare, according to Vlad, is diagnostic assistance. For example, a radiologist looking at a report could take the help of AI to pull up diagnostic guidelines from the American College of Radiology database. The AI system will periodically ask the medical examiner clarifying questions to make appropriate, relevant diagnostic suggestions.
Virtual healthcare assistant: These NLP capabilities could be extended further to create an intelligent healthcare AI assistant. This AI medical assistant will understand conversations using NLU models enabled with medical vocabulary. Trained on medical terminology and data, it would be able to listen and interpret conversations between a doctor and a patient (with consent) so that it can transcribe, summarize the conversation as notes for future reference, and even create structured draft reports (which could take hours to manually create). This would minimize the manual labor of healthcare personnel so they can invest their time in catering to patients.
Image classification and report generation: Extending existing NLP technologies such as automated image captioning to healthcare AI systems would be extremely useful in report generation from images or X-rays. The AI would be able to understand medical images and electronic health records. It could then “post-process” them using deep learning based analytics running in real time and offer prognosis or predict certain medical conditions, such as the potential risk of renal failure.
Vlad believes that tying up all the above potential NLP applications in healthcare would be difficult because the systems are heterogenous (a wide variety of different software from different vendors) in the medical field.
Personal Virtual Assistance
Vlad says that most current virtual AI assistants (such as Siri, Alexa, Echo, etc.) understand and respond to vocal commands in a sequence. That is, they execute one command at a time. However, to take on more complex tasks, they have to be able to converse, much like a human.
Real-time vocal communication is riddled with imperfections such as slang, abbreviations, fillers, mispronunciations, and so on, which can be understood by a human listener sharing the same language as the speaker. In the future, this NLP capability of understanding the imperfections of real-time vocal communication will be extended to the conversational AI solutions.
For example, to plan a series of events, a user will be able to converse with the AI like he would with a human assistant. Vlad gives a common example of a colloquial command:
“Hey, I want to go out to dinner with my friend after my last meeting on Thursday. Take care of it.”
The AI would be able to comprehend the command, divide the complex task into simpler subtasks and execute them.
To achieve this, the virtual assistant would have to consult the calendars of both the user and the friend to determine a common time when they are both available, know the end time of the last meeting on the specified day or date, check the availability of restaurants, present the user with the list of nearby restaurants, etc. The user would be able to review the AI’s suggestions and amend it, after which the AI can create the event in the user’s calendar.
Automotive
Vlad views the automotive industry, particularly cars, as a “preeminent AI platform.” Automotive is a fast-moving consumer tech area, and car OEMs are evolving fast. Vlad is convinced that cars will be increasingly used as autonomous robots whose transportational capabilities could be augmented with other onboard computational capabilities and sensors. He states
“…this [futuristic connected vehicle technology] will not just be a transportation assistant or an autonomous driver but it could also be the user’s personal DJ, concierge, mobile office, navigator, co-pilot, etc.” For example, the user could tell the car to stop at a flower shop on the way home, and the car will have to figure out how to do that.
The user should be able to do this while the music is playing or when the co-passengers are talking (either to their virtual assistant or among themselves). Vlad says that Nuance’s current technology has the ability to “tease the audio out of all this [noise].”
Another example Vlad provides that could make his vision a possibility in the future is that of a car AI being able to independently communicate with the driver’s home AI systems to delegate certain commands it can’t carry out by itself.
For instance, the car assistant should understand the user’s command to open the garage door on arrival. The assistant should also “know” that it is not directly equipped to carry out this command. Therefore, it would identify and interact with the appropriate AI software that can open the garage door. In fact, Amazon recently announced that BMW will integrate Alexa into their vehicles in 2018, which will give the drivers access to their Alexa from their cars through voice commands.
Customer Service
In the customer service field, Vlad believes that advanced NLP technologies could be used to analyze voice calls and emails in terms of customer happiness quotient, prevalent problem topics, sentiment analysis, etc.
For example, NLP could be used to extract insights from the tone and words of customers in textual messages and voice calls that can be used to analyze the frequency of the problem topic at hand and which features and services receive the most complaints, etc. Vlad elaborates that using clustering in NLP for broad information search, businesses can coax out patterns in the problem topics, tracking the biggest concerns among customers, etc.
Further, these technologies could be used to provide customer service agents with a readily available script that is relevant to the customer’s problem. The system could simply “listen” to the topics being addressed and mesh that information with the customer’s record.This means that customer agents needn’t spend time on putting callers on hold while they consult their supervisors or use traditional intraweb search to address customers concerns.
Some Concluding Thoughts
Vlad’s future vision of NLP applications conjures an AI world that is an ecosystem unto itself with a vast improvement in the interoperability between different AI systems. This means, a user will be able to communicate or interact with his/her home AI system right from their vehicle or workspace. Many such voice-controlled NLP systems have already made their way into the market, including apps that help control smart machines like washing machines, thermostats, ovens, pet monitoring systems, etc., from a phone or tablet. Below are some of the insights from the future vision of Vlad’s:
Human-like virtual assistants: Virtual assistants will become better at understanding and responding to complex and long-form natural language requests, which use conversational language, in real time. These assistants will be able to converse more like humans, take notes during dictation, analyze complex requests and execute tasks in a single context, suggest important improvements to business documents, and more.
Information retrieval from unstructured data: NLP solutions will increasingly gather useful intelligence from unstructured data such as long-form texts, videos, audios, etc. They will be able to analyze the tone, voice, choice of words, and sentiments of the data to gather analytics, such as gauging customer satisfaction or identifying problem areas. This could be extremely useful with call transcripts and customer responses via email, social media, etc, which use free-form language with abbreviations and slangs. This ability will also be put to good use in gathering intelligence from textual business reports, legal documents, medical reports, etc.
Smart search: Users will be able to search via voice commands rather than typing or using keywords. NLP systems will increasingly use image and object classification methods to help users search using images. For example, a user will be able to take a picture of a vehicle they like and use it to identify its make and model so they can buy similar vehicles online.
Subscribe to our AI in Industry Podcast with your favorite podcast service:
A Glossary of NLP Terms
Natural Language Processing – A branch of artificial intelligence that helps computers understand, interpret and manipulate human language. NLP draws from many disciplines, including computer science and computational linguistics, in its pursuit to fill the gap between human communication and computer understanding. (Source: sas.com)
Natural Language Generation – How computer programs can be made to produce high-quality natural language text or speech from computer-internal representations of information or other texts. (Source: inf.ed.ac.uk)
Sentiment Analysis – The detection of aGtudes enduring, affectively colored beliefs, dispositions towards objects or persons. Sometimes referred to as: “Opinion extraction”, “Opinion mining”, “Sentiment mining”, or “Subjectivity analysis.” (Source: web.stanford.edu)
Semantic Analysis – A theory and method for extracting and representing the contextual-usage meaning of words by statistical computations applied to a large corpus of text. The underlying idea is that the totality of information about all the word contexts in which a given word does and does not appear provides a set of mutual constraints that largely determines the similarity of meaning of words and set of words to each other. (Source: lsa.colorado.edu)
Syntactic Analysis – The goal of syntactic analysis is to determine whether the text string on input is a sentence in the given (natural) language. Syntactic analysis can be utilized for instance when developing a punctuation corrector, dialogue systems with a natural language interface, or as a building block in a machine translation system. (Source: fi.muni.cz)
Conversational User Interface – A conversational user interface is the ability of artificial intelligence-supported chatbots to have verbal and written interactions with human users. (Source: searchcrm.techtarget.com)
Chatbot – A chatbot (sometimes referred to as a chatterbot) is a computer program that attempts to simulate the conversation or “chatter” of a human being via text or voice interactions. A user can ask a chatbot a question or make a command, and the chatbot responds or performs the requested action. (Source: searchcrm.techtarget.com)
This article was sponsored by Nuance Communications. For more information about content and promotional partnerships with Emerj, visit the Emerj Partnerships page.
Header image credit: The Voice in the Machine