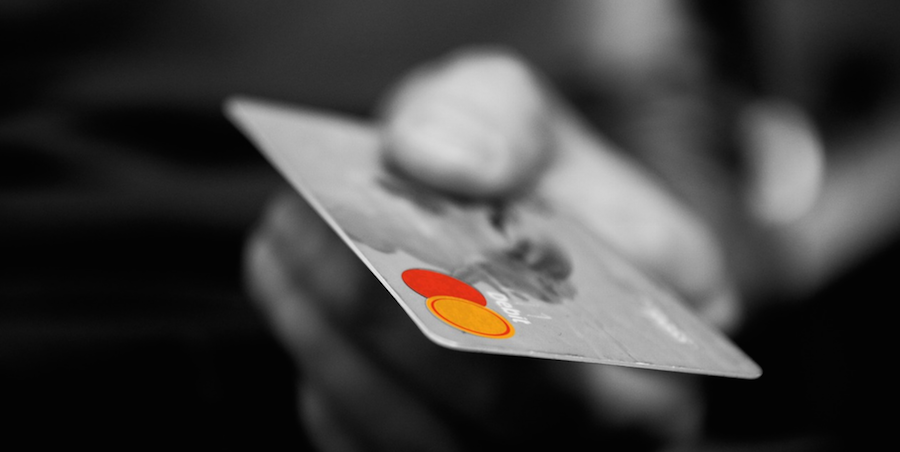
In 2015, fraud losses on credit, debit, and prepaid cards issued worldwide reached $21.84 billion, according to a Bloomberg report. By 2020, Bloomberg predicts that this could grow by a rate of 45 percent.
From our research, we have found that both banks and merchants are looking into AI applications which could protect their customers. In this article, we will discuss seven companies which claim to leverage AI in order to prevent, detect, and defend against credit card fraud. The listed applications fall into the following two clusters:
- Applications for Merchants and Retailers
- Applications for Issuing Banks
In providing this industry application report, we aim to paint a clearer picture of the landscape of solutions that detect and prevent credit card fraud. By shortlisting potential applications below, we hope this report helps business leaders explore whether deploying a preventive solution is right for their business.
For Merchants and Retailers
IdentityMind Global’s eDNA
To help merchants, financial services consultancies and payment service providers distinguish fraudsters from customers, IdentityMind Global says they have developed a machine learning-driven software called electronic DNA (eDNA) which the company claims can establish a customer’s digital identity. The eDNA does this by collecting more than 50 data points about a potential customer, such as email, phone, location, passport number and technology devices used. These details define an individual when they transact online and are updated as the customer evolves.
According to the company, the solution allows merchants and banks to add more security layers to the authentication or risk process if more checks are needed. This enables the eDNA to analyze and identify the identity’s reputation score or risk profile.
The company says machine learning algorithms search the world wide web for the customer’s recent online activities such as payment behavior, social media, social security, IP location, device activity and billing address. The more data points the algorithms gather of an individual, the lower the risk factor.
This 3-minute video explains how the solution identifies and correlates the attributes of an individual or company to establish trustworthiness.
A component of the solution called IdentityLink establishes relationships between identities to determine if these are permutations of the same fraudster or collaborators in fraud, the company reports. For instance, a potential fraudster could list different name variations when trying to open accounts: Jessie Jefferson, Jess Jeffreys, J. Jefferson, Jeff Jesse, etc. The algorithms will see patterns in the data points gathered such as the IP address or devices from where payments are done, the bank accounts these names are associated with, and the payment behavior.
Establishing these relationships between identities also helps build a customer’s reputation score and is the basis to approve or disapproved transactions, according to the company.
According to a recent case study from the company, Goldmoney claims to have used IdentityMind to automate its know-your-customer (KYC), anti-money laundering and fraud prevention activities. Goldmoney said that since using the application, it has achieved “hockey-stick” growth, gone public with $2 billion in client assets and approved new customers from more than 150 countries.
To start KYC’s process, the user first fills out a form with basic identity information based on physical documents provided by the potential fraudster. The user activates the system to search third-party organizations for details about the individual such as bank accounts, social security, and tax identification number associated with that individual. If that applicant is proven to have used another person’s data and tried to apply for an account, the system will mark his application as suspicious.
IdentityMind’s Chief Technology Officer (CTO) and Co-Founder Kieran Sherlock graduated from Trinity College in Dublin with a Bachelor’s degree in Computer Science. Other leadership with AI backgrounds could not be found and were not listed on the company’s website.
SiftScience
SiftScience’s similar solution for merchants called Digital Trust Platform claims to use machine learning to find a variety of nuanced data sources to determine the trustworthiness of a customer, and continuously updates this profile. This may allow merchants to monitor and be alerted of fraudulent activity such as chargebacks, fake accounts, spam, account takeover and referral fraud in real time.
Among the online activities and other details the platform checks are user purchases, transactions and orders; email, billing, shipping and IP address; payment methods; custom data and technology devices used. It also gathers information and analyzes highly-detailed behavioral patterns such as browsing patterns, keyboard preferences and screen tilt.
SiftScience claims that its machine learning models have learned from data collected in real-time over the past six years, from thousands of third-party sites and apps. The data is used to determine if an individual is suspicious or not.
According to the company, if an individual places a suspicious order on an eCommerce website, a SiftScience human analyst be notified. They are then able to see the individual’s auto-generated profile showing the data associated with the individual’s cards, such as a history of purchases, IP addresses where the card was used, recent online orders and addresses physical associated with their account, according to the company.
Using these signals, the system assigns the individual a risk score from 0 to 100 which is displayed to the analyst on this profile page. A score of 100 shows the highest risk, according to SiftScience. A labeling mechanism allows the analyst to click a button to decide whether they believe a user is fraudulent or not. The company claims that the human labeling is sent back into the platform to increase its future accuracy and better its algorithms.
In the 3-minute video, a SiftScience’s solutions engineer demonstrates how the product claims to investigate a customer by checking online activities within a date range, removing or adding fields, or checking a customer’s risky signals.
One client, Mercari, is a Japan-based mobile peer-to-peer eCommerce marketplace. When the company expanded to the U.S., it said it discovered that this market saw more fraudulent behavior. According to SiftScience’s case study, Mercari said they needed a solution that could prevent fraud and reduce chargebacks by evaluating buyer and seller behavior.
When choosing SiftScience, the Mercari team did not need to update SiftScience machine learning model with historical data, but used live data instead to assess its accuracy. The Mercari team planned to give the models one month to train on the data.
Two weeks into integration, Sift Science claims that it was turning out accurate scores. Within one month, Mercari claims it was confident of allowing the system to automatically ban high-risk orders. Within three months of using SiftScience, Mercari’s chargeback and fraud rates dropped by 60%.
SiftScience’s clients also include Open Table, Traveloka, and Touch of Modern.
Alex Paino, the technology lead at Sift Science, holds a Bachelor of Science in computer engineering and mathematics from the University of Missouri-Columbia. He also claims to have taken graduate-level courses in machine learning. In total, SiftScience says it employs nine software engineers focused on machine learning.
Jumio
Jumio offers NetVerify, which the company claims can detect and prevent credit card fraud in real-world transactions by leveraging machine learning, biometric facial recognition, and computer vision, according to the company website. The company explains that human review is needed as an added verification layer, granted the reviewers have the experience, expertise, and training to see recognize patterns.
The solution claims to combine ID card verification, identity verification, and document verification, which the company says establishes the real-world identity of a consumer. The company says the software can scan an ID to determine that a customer is who they say they are, while also catching fake IDs.
For example, if an individual applies for a credit card in-person, the individual could be asked to show a government-issued ID card. The credit card issuer would then scan the ID with Netverify’s smartphone app camera. The issuer is also prompted to take a photo of the individual’s face and upload it onto the Netverify platform. According to Jumio, the software will attempt to match the face on the ID card to the individual’s photo.
Jumio claims that its biometric facial recognition system, with eyeball tracking, can catch the most minute facial movement and features to deter would-be fraudsters. The system can also recognize any attempt to tamper with the ID such as cropping a piece of an image or ID.
The company says that Netverify is targeted at industries including financial services, travel, retail, gaming, telecom, crowdfunding, and home or ridesharing.
This short video shows how a merchant can upload the application on a mobile phone and use it to verify the identity of the customer by doing an ID scan and real-time selfie.
Jumio claims that this technology could increase checkout completion by reducing false declines. The company also claims it recognizes IDs from 200 countries and has verified more than 120 million identities in web and mobile transactions including KYC, anti-money laundering, and General Data Protection Regulation (GDPR) guidelines.
The company also reports that it has gained a massive database of fraudulent transactions and IDs that enable it to detect fraudsters from merchants and bank systems. This database also trains the computer vision algorithms of the software to enable it to recognize trends.
Before implementing Jumio, online gaming company 888.com said they used a system that required gamer applicants to upload an image of their government-issued ID directly to 888.com. Verifying the images and approving memberships took up to 72 hours.
“The manual process was time consuming and there was concern that we were losing customers due to them losing patience with the process.” – Russell Medley, Director of Fraud and Risk Management at 888.com.
Jumio claimed it has hired a staff to manually process the documents. Today, 888.com says applicants have the option to submit via the Jumio solution, using personal webcams to photograph their images and IDs. Jumio claims individuals will get an approval decision within two minutes.
Jumio CEO Stephen Stuut holds a Master of Science from the College of Optical Sciences, University of Arizona, and an MBA from the Wharton School at the University of Pennsylvania.
Labesh Patel is both Chief Technology Officer and Chief Scientist. He earned his Masters of Science in Electrical Engineering degree from Stanford University and Bachelor of Technology from the Indian Institute of Technology in Kanpur.
FeedZai
Feedzai’s is a fraud detection company that claims to work with data and fraud insights from the merchant’s and banks’ systems to create detailed risk profiles of customers. Feedzai claims that can be done in milliseconds as an incident or transaction is happening. Among the data points Feedzai says it gathers are IP addresses, geographic locations, past transactions and patterns of abuse such as hacking accounts and changing passwords.
The company claims that the solution’s machine learning capabilities eliminate manual analysis and rules coding as it builds from each transaction in real-time, as well as expedites and streamlines the decision-making process. The company website states that merchants can review cases and transactions with Feedzai’s scoring engine from the interface and online activity monitoring console.
One of Feedzai’s clients, a US retail bank, required a risk assessment solution that could sift through new online account applications and approve customers smoothly. Before Feedzai, the client said manual reviews resulted in false declines of good customers by as much as 50 percent. Since implementing the Feedzai solution, the bank has reported a 70-percent rise in application approvals. The company also claims that the application helped reduce false positives by 10 times although did not provide specific numbers. The case study also reports that new data streams now integrate within weeks.
Managing overall product development, technology strategy and implementation is CTO Paulo Marques who holds a doctorate in informatics engineering from the University of Coimbra.
The short video below explains that Feedzai is used at the checkout where credit card transaction is processed, whether it is online or in real life. During processing, the software uses AI and machine learning to find patterns in data points and user behavior associated with the credit card account, to predict fraud.
The algorithms go through data within the merchant systems to analyze online risk factors. Once the algorithms find patterns and establish the risk, the system blocks the order. Risk scoring capabilities give merchant analysts about decisions about the authenticity of orders.
While Feedzai did not identify clients on its website, however, the company notes that investors include Citi Ventures and Deloitte.
Solutions for Issuing Banks
Iovation
Iovation says its solution for issuers is founded on device intelligence, a technology that determines device profiles to potentially stop fraudulent transactions from occurring. Called FraudForce, the solution is described as having the ability to detect within about 100 milliseconds which personal devices, such as smartphones and computers, may pose a risk to the business, enabling them to stop the fraud before it happens.
The company reports that FraudForce collects information about the device through cookies and web beacons the moment a customer visits the bank’s website. The solution investigates where the device is being used from, what it has been used for recently, and if it has an abnormally high amount of online activity. This is part of the solution’s evaluation for fraud patterns and recommends a denial, approval or review of the transaction. The company claims that this process happens in about a tenth of a second.
The company offers another solution, called SureScore, which it claims to analyze millions of transactions and attribute permutations to predict the outcome of a first-time transaction. This outcome comes in the form of a trust or risk score, which is determined by machine learning algorithms that detect behavioral, contextual, relational and attribute patterns from devices and accounts, the company reports. In effect, the application alerts users whom to trust and whom to be wary of.
According to CEO Greg Pierson, 500,000 reports of abuse in a month are fed into the SureScore system, which in turn finds links to an individual’s device. The AI system then provides feedback. He also explains how the system watches out for internal fraud, activities that are done by the bank’s own employees from behind their firewall. Additionally, according to the website, SureScore is trained by accessing more than 35 million fraud and abuse reports contributed by Iovation’s network of 3,500 fraud analysts.
When a visitor enters an eCommerce site — and approves the cookies — the company says that SureScore machine learning algorithms immediately work to track the account or device’s IP address. The company claims it also can identify patterns and user behaviors. The algorithms then look for relationships between a visitor’s devices or account and those of suspected or known fraudsters.
If the algorithm finds similar attributes within its database of online transactions and confirmed fraud incidents, the user is given a high-risk score. If no patterns are detected, the user is given a high-trust score. The website reports that this identification and bank notifications happen in real time.
In this 3-minute video, the CEO explains how technology devices represent an individual and how these devices are used by Iovation’s clients.
In an Iovation case study, Forrester Consulting, a financial services issuer of private-label credit cards and bank cards, was experiencing higher fraud rates within its internet channel. The Forrester needed a solution that could reduce the number of false-positives while uncovering the fraudsters. The company chose Iovation fraud prevention solution to identify devices, according to Iovation’s case study.
The report claims that the organization reduced losses from fraud by “identifying fraudulent applications early and reducing the number of false positives.” The report also claimed that the company improved operational efficiency by “reducing time spent diagnosing potentially fraudulent applications.”
Iovation claims it was better able to target high-value customers and achieved a risk-adjusted, two-year return on investment (ROI) of 321 percent within six months and saved more than $8 million.
CTO Scoot Waddell holds a Bachelor of Science degree in electrical engineering from Yale University and a Master of Science in the same field from Purdue University. John L. Taylor, who manages of analytics and data science within the company, specializes in statistical analysis, data mining and machine learning. He studied towards a Master of Science in Logic and Computation at the Carnegie Mellon University, but did not complete his degree.
Some of the company’s clients include Orbitz, Citibank, U.S. Bank, Intuit, and Enova.
Biocatch
Biocatch is a behavioral biometric solution that identifies online fraudsters by their actions such as level of familiarity in the application process, navigation and data input when they visit an ecommerce site. To prevent real-time fraud, issuers embed this technology onto an application or website, where it conducts continuous authentication by collecting and analyzing more than 500 attributes once the user logs in.
From the analyst station, the system flags unusual behavior such as the would-be frauster’s familiarity in the onboarding process because they have repeated experience in creating an account in the site. They are also familiar in navigating the site and have unusually advanced computer skills such as using keyboard shortcuts and function keys, compared with average computer users. Analysts also note potential fraudster’s low familiarity with the data they attempt to enter, making them slower to type the data.
In 2017, a global credit card client deployed the BioCatch solution to reduce the number of false declines. The client faced challenges from fraudsters who use stolen personal information or fake identities to apply for credit cards. Before Biocatch, the client used static methods of verifying identity – personal data, device ID. Deploying BioCatch added another layer of security as the software used behavioral biometrics technology in the online application process to distinguish legitimate users from fraudsters.
The case study reports that the user experience remained smooth while BioCatch detected the fraudulent applications that other solutions did not flag. The result was 33 percent fewer false declines, 50 percent more accurate fraud alerts than existing solutions, and 100 percent alerts either confirmed as fraud or highly suspicious.
This video demo shows how Biocatch analyzes behavioral patterns of the customer, such as which hand is being used, the way information is being input or how the site is navigated. The company claims that even if a fraudster captures a customer’s identity, the solution will conduct continuous authentication in real time.
The human analyst will be able to see the behavior, classify it as a fraud, ID the fraudster’s geographic location and note the type of device used. The analyst can also review the recorded video of the fraudulent session, detailing the fraudster’s touch and swipe behavior to observe differences between the real customer and the fraudster.
According to the company homepage, the solution can also be used by merchants, gateways, processors, card associations, and acquirers.
Biocatch CEO Howard Edelstein, who holds Master of Science in electrical engineering, from Stanford University, wrote on LinkedIn, “Why shouldn’t there be a better way to know it is really you in this dark world of “online” identity? Not just by what personal questions you can answer correctly, (what you know), or by a token (what you have), but rather by who you are, as modeled by the behavior you exhibit while doing everyday things.”
Avi Turgeman is the Chief Technology Officer who leads behavioral biometrics, machine learning, deep learning and artificial intelligence developments in the company. He holds a Bachelor of Science degree in Physics and a Master of Science degree in Philosophy of Science. Avi also has 17 patents to his name related to devices, systems and methods for cybersecurity.
Decision Intelligence
Global financial institutions such as Mastercard also developed an in-house solution called Decision Intelligence, described as a real-time decisioning solution that applies thousands of data points and modeling techniques to each transaction to expedite the approval of genuine customers.
This video shows how Decision Intelligence claims to match the customer’s historical purchase behaviour before approving the credit card purchase. Among the data points the solution checks are customer value segmentation, risk profiling, location, merchant, device data, time of day and type of purchases.
To cite an example, a customer enters an eCommerce site and places an order for a pair of running shoes and a hydration vest. Decision Intelligence’s machine learning algorithms check data from merchants and Mastercard’s database to find patterns and determine whether this purchase is consistent with the buyer’s usual online behavior.
According to the company, the algorithm looks through data points such as purchase type, time, location, and purchase cost to see if the customer’s patterns are familiar. It also checks other attributes such as IP address, device ID, email and phone number.
The application also then checks merchants’ systems to find out if the customer is assigned a risk or trust score. If the algorithm shows no patterns consistent with fraud, the system will approve the purchase. If not, the company says it may deny the purchase. The company claims that this assessment process happens in real time across the systems.
Concluding Thoughts
A number of startups are able to offer their solutions to other financial organizations. For instance, Feedzai, Identity Mind Global, and Mastercard also offer their applications to acquiring banks who address merchant risks. These AI companies claim that their technologies create a good experience for model merchants and keeps away fraudulent ones.
The startups claim that their software gives the acquiring banks a centralized way to track merchant activity in real time, ability to continuously assess compliance risk, and exposure from the bank’s analyst stations. The clients also receive alerts and escalations triggered by suspicious activities by the merchant, according to these companies.
In summary, a common trait of the above credit card fraud detection and prevention applications is the ability to build and analyze digital identities. These identities are often based on data points or attributes gathered from multiple sources. For the above companies, these basic attributes include email, phone, geographic location, passport and personal devices used.
Aside from these data points, these AI-powered solutions also claim to collect biometric data such as facial features and eye movement; technology device attributes such as screen touches and swipes, keyboard strokes and tilt of the screen, and locations where the device is being used; online transaction behavior such as time of day, browsing patterns; as well as transaction history such as types and amounts of orders and shipping and billing address. These companies claim that attributes will continue to build up as the customer’s online activities grow.
Despite these benefits, a common theme that persists is continued human supervision to make the solutions more efficient and effective. Human analysts are still needed to investigate fraudulent patterns.
As the technology suggests, machine learning is still that — learning and evolving. As Jumio CEO Stephen Stuut, said in an interview “When we introduce a new technology, we still have the human interaction, and then if it works, we’ll back up with machine learning but keep looking over it.”
Header Image Credit: Pixabay