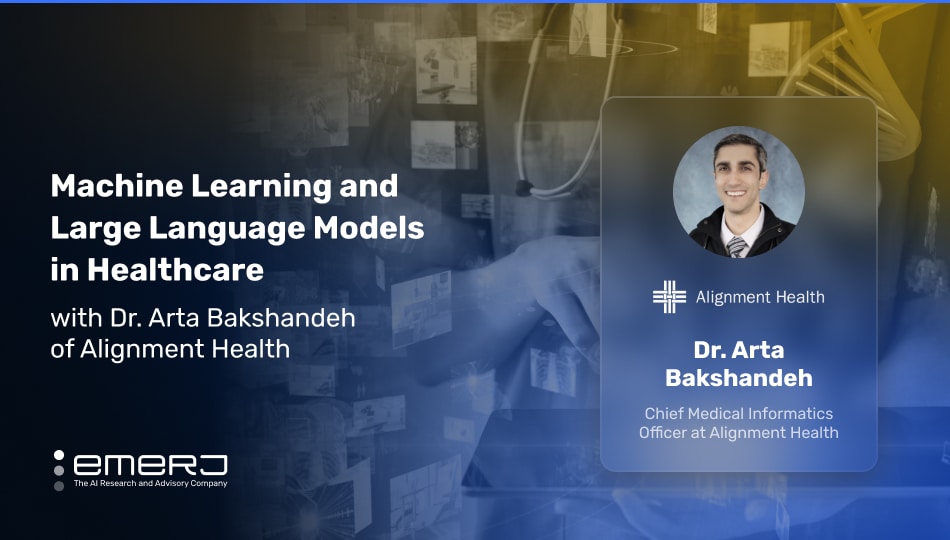
AI and machine learning have promising potential in the field of healthcare. However, there is one significant hurdle healthcare companies often encounter when trying to implement AI and machine learning. The data architecture of current legacy systems in healthcare settings prevents implementing many use cases and limits a company’s ability to benefit from these technologies fully.
A 2022 study published in the Journal of Digital Economy showed that machine learning capabilities are increased with improvements in data architecture coherence. Without an underlying unified layer that can integrate and synthesize disparate data silos, healthcare institutions will be unable to take advantage of the benefits AI and machine learning can offer.
Legacy systems might have served their purpose in the healthcare space for decades, but technological advances enable better healthcare workflows, reduced administrative burden and more. Modernizing legacy systems by eliminating data silos results in numerous benefits, including improved work-life balance for physicians.
Notably, we are a long way away from having AI serve as a substitute for clinician decision-making. Immediate use of AI in the coming years will focus on supplementing healthcare providers, allowing them the ability to scale.
Emerj Senior Editor Matthew DeMello recently sat down to talk with Dr. Arta Bakshandeh, Chief Medical Informatics Officer at Alignment Health, on the ‘AI in Business’ podcast about challenges facing the healthcare industry and how AI and machine learning can help alleviate them.
This article will focus on three key takeaways from our interview with Dr. Arta Bakshandeh.
- Eliminating data silos in hospital legacy systems: Create a pipeline across disparate silos to achieve a unified data architecture.
- Decreasing caretaker burnout: Using machine learning to reduce the administrative burden on physicians and improve patient care.
- Steering systems away from bias: Focusing on building AI algorithms that identify which might need a service that was not previously identified, rather than focusing on building systems that deny patients from services.
Listen to the full episode below:
Guest: Dr. Arta Bakshandeh, Chief Medical Informatics Officer, Alignment Health.
Expertise: Machine learning, Artificial Intelligence, Data Science
Brief Recognition: Dr. Arta Bakshandeh began as Senior Medical Director at Alignment Healthcare nine years ago before taking up his current role as Chief Medical Informatics Officer. Dr. Bakshandeh also serves as an Assistant Clinical Professor at Keck School of Medicine of the University of Southern California.
Eliminating Data Silos in Hospital Legacy Systems
Bakshandeh explains how legacy systems prevent hospitals from being able to take advantage of AI and machine learning. He described how healthcare institutions are creating millions of data points every day that are relegated to siloed systems.
Bakshandeh offers, “And if you have a data architecture, which then creates a pipeline across each of these silos, that will then allow you to harness a lot of AI and machine learning and data activation, as we like to call it.” He indicates that this is what Alignment Health accomplished through their Alignment’s Virtual Application (AVA) system.
Bakshandeh describes the system that Alignment Health created, which they called AVA. The cloud-based system consists of over 35 applications. He explains how it contains a unified data layer where data goes through a normalization process after ingestion.
Rules are applied at the next layer. Through a communication channel in the cloud ecosystem, healthcare systems can scale up and down as they need data.
The AVA system has powered more than 1 million personalized care interactions. It does so by first unifying member data sets and then applying more than 160 AI models.
Some benefits of AVA include:
- Improved clinical outcomes by alerting care teams about potential interventions
- Predicts care alerts, including risk for hospitalization and disease propensity before early warning signs result in an emergency
- Increased ability to deploy timely, effective and coordinated care
- Bakshandeh goes on to explain that while the system was initially built for clinicians, it is now used for entire healthcare operations, including:
- Quality case management
- Utilization management
- Houses Alignment Healthcare’s CRM
He further explains how such architecture would not be available if not for advances in how cloud ecosystems are able to use APIs.
Bakshandeh also elaborates on the disconnect that still currently exists in hospital systems by describing his experience of visiting a patient recently in the hospital. The hospital had one particular electronic health record (EHR) system, so he had to physically walk to the emergency room to log into their specific EHR system to review what happened there.
Additionally, the outpatient EHR system was a third and entirely different system. This fragmentation highlights why an integrated data architecture is so important to streamline processes in healthcare settings and facilitate efficient patient care.
Decreasing Caretaker Burnout
Bakshandeh explains how we are far away from being able to replace humans in healthcare simply by using AI. He further emphasizes how it’s necessary to use AI and machine learning in the right ways to help scale in order to address the shortage of clinicians. By removing the administrative burden, physicians can spend time with patients in more meaningful ways.
A significant benefit to healthcare professionals from improved workflow is that they will have a better work-life balance. Bakshandeh explains how the average clinician spends 10 hours every week looking for data or charting. This additional focus is overwhelming to many physicians.
COVID-19 accelerated the already-existing doctor shortage; close to 20% of healthcare workers left their jobs during the pandemic, leading to enormous costs. The combined annual burnout turnover costs exceed $15 for doctors and nurses.
He expects that AI and language models are going to do a lot of the work that physicians used to be doing, freeing up physicians to focus on high-quality interactions with patients.
Bakshandeh explains how AI can function as a data scientist on a physician’s shoulder, freeing up the physician to provide direct patient care. He explains how AI can notice something on an X-ray that a radiologist might miss because they’ve looked at so many X-rays.
Large language models (LLMs) such as Chat-GPT can be used for lower-risk areas, such as when patients have questions about their healthcare coverage. Patients benefit by receiving accurate and timely replies to their questions. By simplifying these types of administrative tasks, healthcare professionals can focus on direct patient care.
Steering Systems Away from Bias
When asked about the limitations that exist when implementing AI, Bakshandeh offers essential insight. One significant limitation he mentions is bias. Healthcare providers need to be highly aware of bias.
Implicit bias has been shown to lead to different outcomes depending on a patient’s race, gender, age and even income or insurance status. He expands on this by saying that healthcare providers should not be using AI to deny someone a service. Instead, AI and machine learning should be used to identify patients who might need a benefit that was not previously identified.
Bakshandeh explains the LACE index system and how hospital systems in the U.S. use it to determine the likelihood that a patient will be readmitted. This algorithm is used to determine who should be triaged for case management and who potentially should be followed up with either a home visit or virtual visit after being discharged from the hospital.
AI can utilize the abundance of data points created each day to identify many more attributes than the typical LACE index uses. Additional attributes can increase the accuracy of predictions related to readmission, but this is only possible with the proper data architecture in place.
AI can provide clinicians with insights they previously were not privy to. Suspicious areas on X-rays can be prioritized and brought to the top of a physician’s queue.
Bakshandeh describes a recent example of an ER physician who provided Chat-GPT with examples of patient situations he encountered that day. Chat-GPT missed the ectopic pregnancy diagnosis. Bakshandeh emphasizes how dangerous missing that diagnosis is in large part because of the risk of fatality.