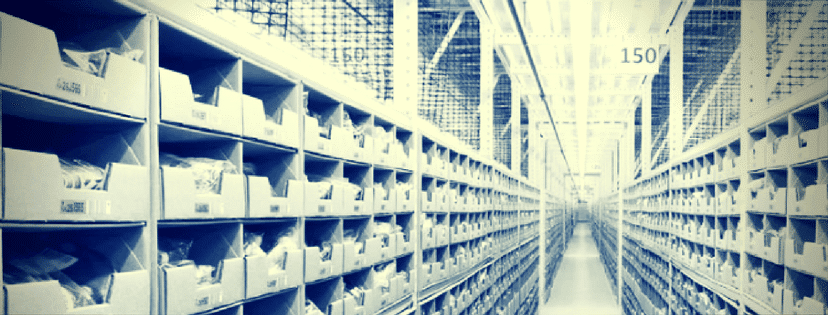
In a global market that makes room for more competitors by the day, some companies are turning to AI and machine learning to try to gain an edge. Supply chain and inventory management is a domain that has missed some of the media limelight, but one where industry leaders have been hard at work developing new AI and machine learning technologies over the past decade.
Many well-known companies are now use machine learning to optimize business processes in ways that might have been deemed science fiction 30 years ago, from customer service inquiries to planning for next month’s shelf supply based on satellite data. Supply chain and inventory management is primed to embody the concept of smart automation over the next five to 10 years.
We’ve highlighted three applications of inventory management with machine learning technology, providing a tip-of-the-iceberg view of what’s possible. The new frontier of supply chain and inventory management allows companies to leverage an abundance of data in new ways—preventing costly facility malfunctions, exceeding customer expectations in product demand and service, and increasing ROI over the long term.
Applications of Inventory Management with Machine Learning
Robots – Seeing to Customer Satisfaction
Two of America’s largest retailers are using robots as part of their inventory management. Over the summer of 2016, Lowe’s introduced its LoweBot in 11 stores throughout the San Francisco Bay Area. These autonomous retail robots not only help customers but create real-time data by using computer vision and machine learning to scan inventory and look for patterns in product or price discrepancies.
The 5” bilingual retail robot features helps customers find the products they’re looking for through a searchable computer display, advanced voice recognition, and laser-based sensors (similar to technology used in autonomous vehicles) that help it navigate around the store. According to initial feedback from Kyle Nel, vice president of disruptive innovation and executive director of Lowe’s Innovation Labs, customers appreciate the convenience and efficiency of the bot, while employees love that LoweBot allows them more time to consult with customers on creative projects.
While Lowe’s is testing robots on the front end, Walmart is testing them behind the scenes. In June 2016, Walmart announced testing of proprietary drones in its massive warehouses to improve inventory management. According to Walmart, manually checking inventory can take about a month for employees, but the same task can be completed in 24 hours using sophisticated drones that fly through the warehouse, scan items, and check for misplaced items. Walmart CEO Doug McMillon stated: “The internet of things, drones, delivery robots, 3D-printing and self-driving cars will allow retailers to further automate and optimize supply chains too.” For more information on how robotics is transforming industry, our recent guide on AI industrial robotics is a worthwhile read.
IoT – Prevention First
If Gartner’s estimates are accurate, by the end of 2017 there should be some 8.4 billion connected things and over 20 billion by 2020. Connectivity goes beyond location of things to their condition. Connected monitors on supply equipment can send alerts about potential problems before they become an issue that would disrupt the movement of merchandise.
An Accenture 2016 report on the industrial IoT concluded predictive assets could save up to 12 percent on scheduled repairs and 30 percent on maintenance, while reducing breakdowns by up to 70 percent. In 2014, Intel’s first pilot into fully integrated IoT manufacturing resulted in $9 million in savings at just one facility.
Predictive Analytics – Weathering Demand
Weather is a major determinant to what consumers buy, as well as where and when they do their shopping. IBM is using Watson to make more accurate predictions about the weather, technology that can be used to help determine supply chain availability and demand. In 2015, IBM acquired the Weather Company to make use of its massive database and collection systems. Watson not only draws weather data from stations but also uses news feeds and social media to create a picture of likely conditions. Predictive weather analytics, which can provide forecasts months in advance, paired with current and planned stock data for individual retail locations, can help retail owners and managers make better strategic decisions on things like supply, pricing, and promotions.
Once again, Walmart is getting in early on ML technology that shows potential to increase profits. Its first IBM Weather-gleaned data sets from 2014 showed some interesting correlations between weather phenomena and consumer purchasing behavior. For example, the company found that people are more likely to buy steaks when it’s warm, windy, and cloudy, while hamburger sales increase in hot and dry weather. The chain used this information to promote hamburger meat, based on weather predictions, and saw an 18% improvement in beef patty sales.
Image credit: LinkedIn