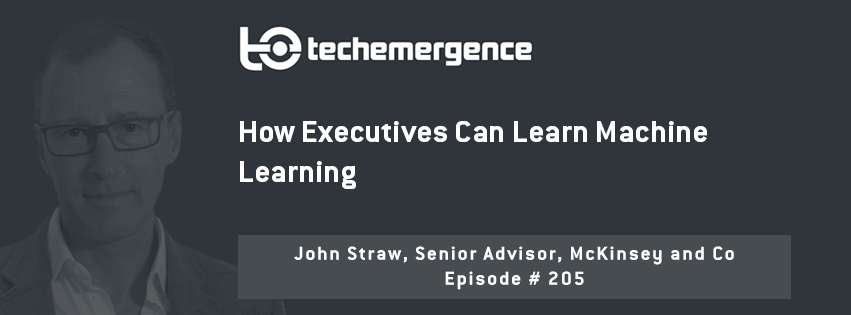
Episode Summary: What are executives missing the boat on and what do they need to think about when it comes to AI and machine learning? This week, we speak with John Straw, who has had a number of businesses in the UK and US and is currently a senior advisor to McKinsey & Co.
John works with a lot of executive teams in finding new applications for AI and machine learning and pinpointing ROI for those technologies in industry. This week, Straw shares his insights on how to solve business problems with machine learning. Straw also touches on aspects he believes are most commonly overlooked when executives learn machine learning, specifically in finding applications that can keep them up to speed with their competitors in the field.
Expertise: Technology/economics entrepreneurship, venture investing, and digital disruption
Brief Recognition: During his 33 years in IT, John Straw has been involved in 4 Internet startups as cofounder or executive with 3 exits. Most recently, he was Chairman of Thomas Cook Digital and author of iDisrupted. He is also an active early stage venture capitalist specializing in marketing and cloud technology investments. In 2015, Straw was appointed Senior Advisor to McKinsey and Co., and in 2016 he was appointed as an advisor to IBM on the subject of Internet of Things with Watson artificial intelligence.
Current Affiliations: Senior Advisor to McKinsey and Co.; Senior Advisor to IBM; Venture Investor with Thorium Technology Investors; Investor and Advisor with BoughtbyMany.com; Investor with CTRLio
Interview Highlights:
The following is a condensed version of the full audio interview, which is available in the above links on Emerj’s SoundCloud and iTunes stations.
1:23 – When you are in the C suite – obviously ML is something that’s relevant for larger organizations and something they should be considering – what do you see as maybe going over the heads of C-level folks that maybe shouldn’t in terms of where AI and ML is headed?
John Straw: “I do a significant amount of speaking to C-levels, and I always start off the conversion with, ‘Who knows what big data is?’, and universally their hand will go up in the air…and the immediate subsequent question is, ‘Who knows what to do with it?’, and there’s this series of slightly embarrassed blank faces, and this is pretty much universal, and the way I would probably typify that is, most organizations – most C-level people – understand what this stuff does, but they don’t know what to use if for; so it’s the use cases, it’s the apps, etc. that really we as a technology community need to be able to think creatively around those things with them…
…there are two ways of looking at this; with the IoT, for example, with advanced analytics, cognitive analytics with IOT, a lot of the focus in that is actually the CFO’s office, because it’s cost-saving; but the flip side of the coin is always the question, ‘How does the client actually make money out of this technology?’, so I think there’s two interesting opportunities here. The one side of that is the consulting side, which will actually help you save money, but then there’s the entrepreneurial side, which actually helps you make money out of it…so in those cases there’ s a lot of brainstorming that goes on on about how we use (machine learning technology)…”
4:10 – What are the applications that maybe are common enough that people should understand them, they should know that this is possible in a business, they should know that these applications can make a difference?
JS: “I would say that most organizations don’t know what data they’ve got, and they don’t know what data they haven’t got…so I think the first thing is, data audits are a very big part of it, that’s both internal data audits and then having the imagination to be able to actually understand what external data…they could use to enrich the data set, and the way that I explain identifying opportunities is simply, think about this as the use of a Venn diagram, to go look at those big data sets and then consequently machine learning, that’s one part…”
12:12 – What are the kinds of features and function in regards to machine learning that maybe under situation xyz you want to let Facebook or Google run that show, and at what point does it make sense, what threshold do we start contemplating building things in-house?
JS: “…The value is not in software, the value is in data, and this is really important for every single company, that they understand what data they’ve got and what format it is, and then they use third-party developers to actually make sure the data is a a level playing field by using parsing functionality…the value is no longer in code, the value is in the data that sits behind it, and a lot of organizations don’t have that data, at least it’s not surfaceable and it’ s not readable data, but again I come back to what I said earlier; you’ve got to do an audit, you’ve got to understand what data you’ve got, secondarily what data that you don’t know that you’ve got, and then thirdly what external data sets that you could actually merge into all that to make something really useful and really interesting…”
15:19 – What are some other maybe tangible examples…of what a unique data set with company X could look like if we combined it and really made sense of it?
JS: “These are all very nascent yet; IBM’s got some amazing technology, image recognition in the radiology space; I just had a conversation with a surgeon recently and he said that actually, radiology is about to get severely disrupted by machine learning capability to identify issues at the point of the scan, rather than waiting for a subjective human being without perhaps enough experience to do exactly the same thing…
…it’s down to the imagination of the organization with the data set to be able to use it…I recently saw a YouTube video where Google was doing apparently image recognition inside of the video and it was phenomenal…and that transforms the advertising business straight away…because all of a sudden it becomes absolute real-time, contextual advertising…”
20:44 – How are executives getting up to speed on these things John, without going back to get a PhD in machine learning, how do you see people successfully grasping this as an executive who already has a lot to do?
JS: “…when I talk to CEOs, I use “Where’s Waldo” to show that this is how pattern recognition works, this is how machine learning works, and they pretty much get it…they don’t need to go back to school, they just need to be focused on the outcomes, but to be focused on the outcomes, they need to be given visibility to lots of use cases, applications, case studies, etc., so that they can get their heads around where the opportunities lie…”