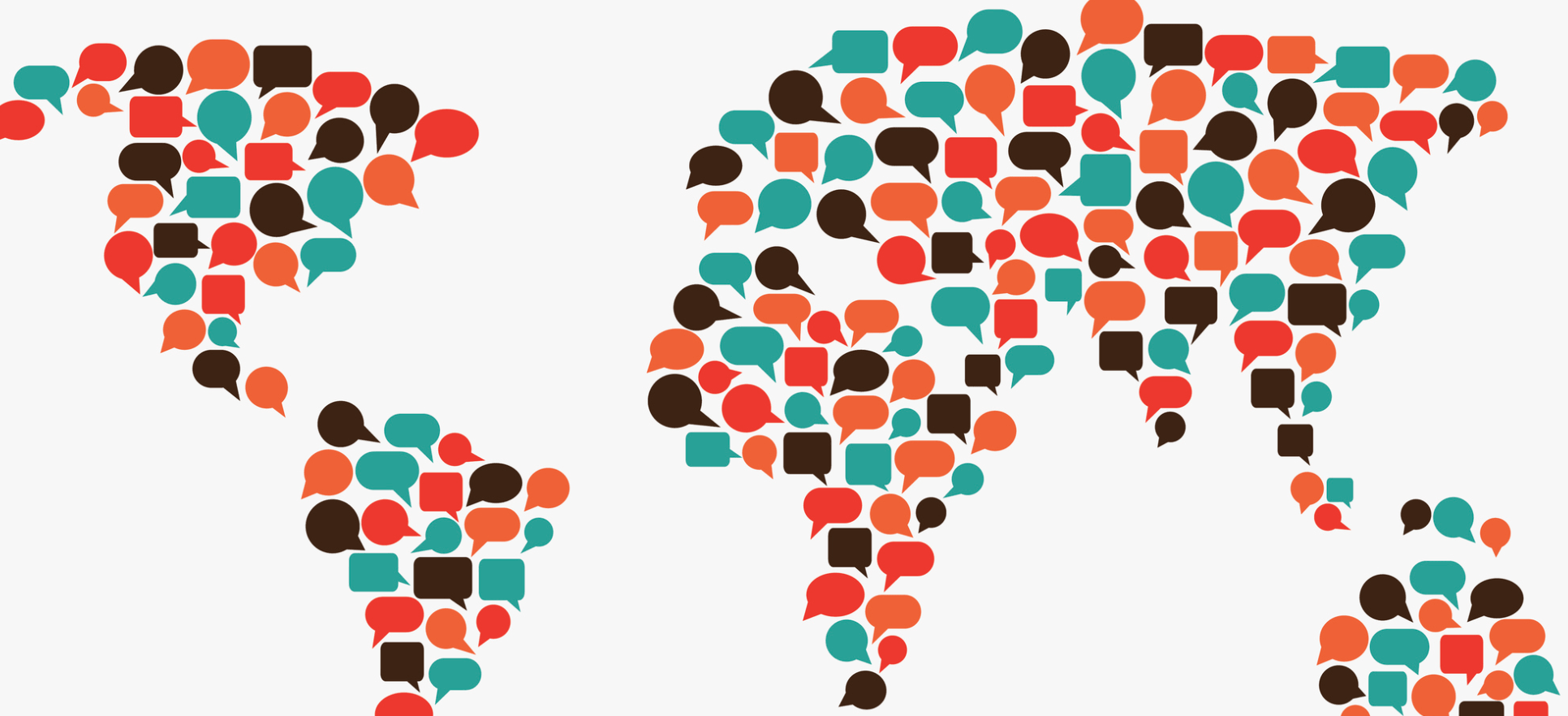
Episode summary: This week on AI in Industry, we speak to Paul Barba (Chief Scientist at Lexalytics) about what how companies are using natural language processing, and what it takes (in terms of expertise, time, and training) to get these systems working. From sentiment analysis to categorization, Paul walks us through interesting and fruitful use-cases and sheds light on the back-end “tweaking” required to keep NLP productive in a changing business environment.
Guest: Paul Barba, Chief Scientist at Lexalytics
Expertise: Natural language processing, sentiment analysis, NLP taxonomies and categorization
Brief recognition: Paul began working with Lexalytics in 2008 and is the company’s Chief Scientist. He earned a degree in computer science and mathematics at the University of Massachusetts, Amherst, where he briefly served as a computer science tutor.
(For readers with a broad interest in NLP use-cases, please refer to our full article on NLP business applications.)
Big Idea:
An NLP system needs to have feedback loops to update and adjust to a changing context or situation, all concrete systems would be too brittle – ongoing iteration is necessary.
Paul explains that businesses need to continuously update natural language processes systems if they want them to continue delivering value. Why? Because the world changes quickly – examples include:
- New abbreviations or symbols are used to communicate on social media every
- New streams of data become accessible (i.e. through a new chat applications, or through a new social media channel)
- Businesses update their products and departments, which may change what an NLP systems needs to look out for
Reviewing and updating systems is part of ensuring their ROI. Paul acknowledges the challenges of using data science talent to solve these problems. Towards the end of this week’s interview, Paul explains what he hopes will be the future of this constant “tweaking” and iteration (hint: It hopefully won’t involve PhDs or AI experts forever).
Interview Highlights on Making NLP Work
Listed below are some of the main questions that were posed to Paul throughout the interview. Listeners can use the embedded podcast player (at the top of this post) to jump ahead to sections that they might be interested in:
- (3:30) What are the most common uses of NLP in business today?
- (5:45) What are some example of the use of sentiment analysis in business?
- (7:30) What is an example of a business use-case of NLP “taxonomies”?
- (12:15) What does it take to implement NLP applications in an existing enterprise? What kinds of teams and expertise needs to be involved to make an application actually work?
- (17:00) What does the ongoing “tweaking” and “adjusting” involve? What needs to be done regularly to continue to update a living NLP system?
Header image credit: Inside Higher Ed