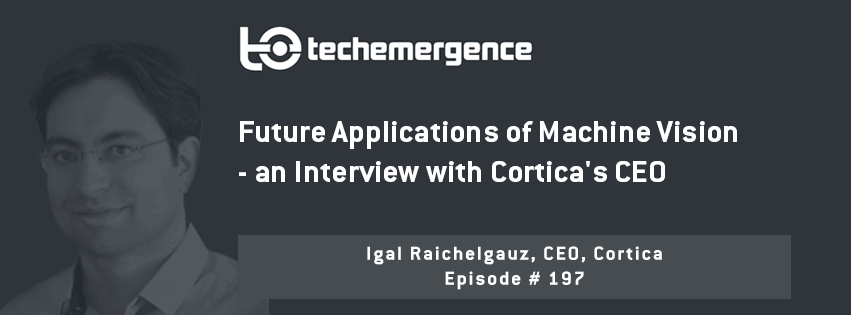
Episode Summary: Right now, you can take a picture of a flower in your garden and post it on social media to see if anyone knows its proper name. Wouldn’t it be nice, though, if a machine could identify the correct name and species in the picture you just took? Solving this problem in applications of machine vision is something that CEO Igal Raichelgauz and his team are working on at Cortica, a machine learning company that is not focused on deep learning, but is instead taking a more “shallow” approach. In this episode, Raichelgauz articulates Cortica’s approach, which is based on neurology and goes against some of the current approaches in getting machines to learn. We discuss some of these primary differences and dive into Cortica’s goals for applying machine vision in consumer products.
Expertise: Computer vision and speech recognition
Brief Recognition: Igal Raichelgauz is CEO and founder of Cortica, and previously served in the role of CTO from 2006 until 2009. Prior to Cortica, Raichelgauz was a project manager at LCB, providing systems and services in the area of speech recognition. He also served as a Technical Lead at Intel Corp. for five years. Raichelgauz attended Technion – Israel Institute of Technology.
Affiliations: CEO of Cortica
Interview Highlights:
(1:44) At Cortica, you’re aiming to differentiate that you’re not going for a deep architecture and you’re also not using supervised learning methods…talk to us what those differentiators are in your approach?
(5:25) To give (an image) its proper name, it would have to be drawing from some sort of source…how does that naming (of the image) after the ‘clumping’ (of several images) happen?
(9:14) When you talk about how our own mind does not leverage networks at the same depth that some folks are leaning into now in order to solve current image problems, talk about how that same kind of recognition and search can be done in architecture that doesn’t have that kind of depth?
(12:31) It sounds like more (deeper) might not be better…is there something about the more minimal result that produces a better result?
(14:19) I know an application that you’re excited about now is in the mobile space, what would better image recognition and better intelligence around visual data allow for folks in the mobile space?
(18:10) What excites you guys about the combination of machine learning and shopping or eCommerce?
Big Ideas:
1 – The brain is not a very deep architecture, but it’s high dimensions and extreme compression constraints (which Cortica is trying to replicate) allow for a different learning algorithm, one that is not supervised but instead rooted in observing information and creating a representation of images. Raichelgauz suggests there may be a number of optimal layers and that more layers can actually lead to degradation of performance.
2 – Applications across industries are ripe for better machine vision, particularly when it comes to evolution of user experience (Shutterstock’s Nathan Hurst discusses different but related applications, particularly in how humans engage with computer vision apps in editing and creating).
A major development will occur when people are motivated to take pictures as a way of engaging with the physical world using mobile technology – this may be the next generation of mobile users, and gaming is a trigger in evolving this type of machine vision technology.