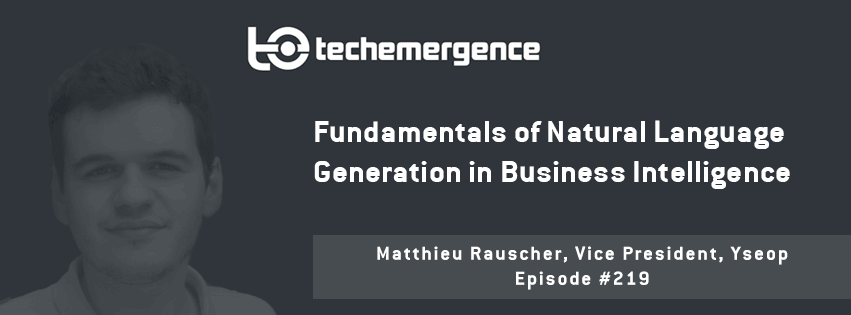
Episode Summary: You might be aware that some of the articles online about sports or financial performance of companies are article written by machines; this machine learning-based technology is the burgeoning field of natural language generation (NLG), which aims to create written content as humans would—in context— but at greater speed and scale. Yseop is one such enterprise software company, whose product suite turns data into written insight, explanations, and narrative. In this episode we interview Yseop’s Vice President Matthieu Rauscher, who talks about the fundamentals of NLG in business, and what conditions need to be in place in order to drive business objectives. Rauscher also addresses the difference between discover-oriented machine learning (ML) and production-level ML, and why different industries might be drawn to one over the other.
Expertise: Applying emerging technologies in marketing and business strategy
Brief Recognition: Yseop currently works with leading companies in the Fortune 1000 globally. As Vice President, Rauscher works with senior professionals in financial services, banking, healthcare and other industries, to help apply machine learning technologies to address existing business problems. Rauscher has held multiple positions since joining Yseop in 2012 as a consultant. He earned his MS in business administration and management from the IESEG School of Management.
Current Affiliations: Vice President of Yseop
Big Ideas:
1 – There’s no good or bad AI; it’s only a question of the tool.
The fundamental nature of ML is trial and error; if you look at businesses that invest in ML, it’s where success has value and error doesn’t have any incidence, says Rauscher. For example, if Google uses ML to improve its advertising, and once in a while a wrong ads show up, there’s no real problem; but if a company is selling financial products and has a 5 percent error rate, it’s not worth the risk. NLG is just one form of AI tool, and it’s particularly suited (at present) to automating the process of gathering lots of data and disseminating these points into one actionable deliverable.
2 – NLG allows service industries to scale “the pilot.”
This is a literal analogy for NLG’s ability to allow (with limited inputs) a system that flies automatically 95 percent of time and the pilot to fly 5 percent of time, or whenever the situation becomes complicated and requires increased judgment. For example, when using structured data, NLG can scale 95 percent of initial financial analysis reporting cases—allowing financial analysts to work on figuring out more complex problems. As we’ve heard many times before regarding machine learning business best practices, Rauscher notes that the more clean data that you have to feed the system, the better your results.
Interview Highlights:
The following is a condensed version of the full audio interview, which is available in the above links on Emerj’s SoundCloud and iTunes stations.
(2:14) How do you explain NLG to people who aren’t from this field?
Matthieu Rauscher: Simply put, NLG means helping you compute and generate text. So in the past we’ve always used templates…the real innovation today is we have software that is coming out that allows you to analyze structured data and use it to generate text on the fly.
(3:25) Sounds like we’re talking about a lot of context…we were talking off mic about structured input…how do context points get pulled into a report, because I can see it being easier to garner what’s (already) on paper, but having to garner insights form other market forecasts that came up the month before, for example—I can see that being a challenge.
MR: The fundamental question you’re asking is where does NLG fit and what are the correct use cases…AI has great potential, but everyone’s selling this as the silver bullet…I don’t believe in that; I believe that every tool is only as great as the problem it solves. When I look at AI, I believe the word is too broad; very simply, the definition of AI is to create intelligent machines…
…there are actually two (AI) ideologies running the world today and they’re competing against one another. The first one is what I call discovery artificial intelligence…the technologies that take massive amount of inputs, take any input and what they sell you is that regardless of the question you ask, they’re going to answer—the ideology behind people running this is they want to create AI that’s as great as human beings…the problem with that is the error rate; when you do ML, it’s based on the fact that correlations and causality are the same…when you are the expert, and you want to be smarter, discovery is fantastic…the second family in AI is what I would call production AI…very simply, I have a controlled set of inputs and I want to scale my output…
(15:54) Maybe you can walk us through what an example looks like…what kind of data is the machine taking in the front end, and how is it structuring things…just so people can get a sense of what NLG in business looks like.
MR: I think that when you’re in production, you have to program everything. The “war”, i.e. which vendor wins, depends on the level of effort; if in 10 days you can build an application to put in production and generate a million pages of financial reports per day, it’s very easy to do and it’s very simple; if it takes you a thousand days to generate, at the end, a hundred pages, it’s not worth it, so that’s really what we focus on…
(18:25) (Initial context) is something that a computer itself doesn’t know…is this kind of contextual stuff part of the initial input that gets put in the front end?
MR: That’s exactly what the analyst does when he builds this application, he’s going to say “I’m the expert, I’m going to teach the machine everything I know so it’s going to scale it and I can focus on something else”…my qualitative information is what allows me to know, what do I need to talk about and how do I need to talk about it, and once I have these results I’m going to start generating the text; and the text..that layer, the user interface, that is the pure NLG…fundamentally it’s a template that conjugates itself…allows you to write 10 or 15 time more text with only once centralized template…
…and then if you want to take it to the next level, which is where you have an additional layer of intelligence, which is particularly important for people who do reporting on data…when you have comparisons and things like that…this is the ability of not just expanding what just happened, but explaining the underlying data and explaining why it happened.
(24:56) We just talked about reporting, there’s one other major use case that we were talking about off mic..to add some color to what NLG means and does in a real business environment, what is that other use case and how does it work in basic terms?
MR: When we look at data in general, there’s only two reasons why we do (business intelligence) BI. The first reason is to figure out what the data means for me—that’s the reporting or insights— and the second one is what should I do about it, which is action. Together it’s actionable insight…and we have one specific use case…a CRM (today) is one hundred thousand data points, which we send out to sales people expecting them to use this data in order to sell to 50 customers that they’re going to call today, but there’s too much data, it’s impossible…
…in a lot of industries, you have so much regulation that when you look at the data and you find a product that is good for the customer, it’s difficult to figure out what the procedure is to follow in order to sell the products; so what we do is we have a linguistic user face on top of the CRM…you just have one page that says “here’s where the customer is, here’s what you need to know about him, here’s what you need to sell, and here ‘s how you need to sell it”…
(27:49) What’s the front-end programming that would happen in a CRM insight use case, where we’re trying to figure out what’s the best way to allocate sales person time?
MR: This is…the perfect example of where you have the value of language. Data is useless if you don’t use it…a sale is (the same thing as) saying, you need to sell this car because _____.
…if you’re a young mother, I’m (the system) going to say the Toyota Corolla (for example) is great for your children because there’s lots of room for a car seat, we have the safest car in the market, and it doesn’t consume a lot of oil, which is great for your children’s future…it’s the argumentation that makes the sale…