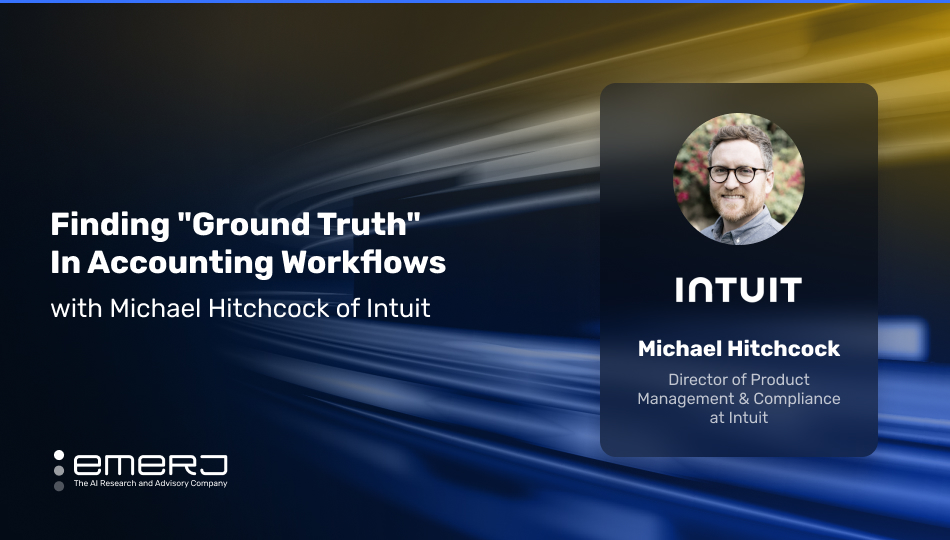
While accounting as a discipline has roots going back to the 13th century, current-day accounting software is still based on manual record-keeping just as it was in the 1990s, only with digital checks in a digital checkbook register, allowing users to keep track of their finances on a computer.
The widespread use of artificial intelligence is having a transformative effect on accounting for both business owners and the accountants they rely on. As Alexander Hagerup, CEO of Vic.AI, explained on a recent episode of Emerj’s ‘AI in Financial Services’ podcast, “The big change that AI has the capacity to do is change things but change things differently.”
Previous developments in accounting focused on changing where the data was registered, but AI changes the dynamic significantly. In Alexander’s words, “With AI, the ‘how’ is changing. It will actually be the AI that is performing the human work of registering accounting transaction data.”
That doesn’t make the challenges of AI adoption in financial services any easier, specifies Boston Consulting Group Managing Director and Senior Partner, Jeanne Bickford.
“You can’t just start using AI – you actually have to be able to do a lot of the work around model validation and model risk management, such as being able to analyze the data lineage, make sure that there isn’t built-in bias,” she tells Emerj. “You have to have some of those controls in place, which pre-AI models you already had to have that in place.”
In today’s business environment, artificial intelligence can automate parts of the accounting workflow. That automation allows business owners to focus on running and growing their businesses due to the time savings. AI also enables more efficient interaction between business owners and accountants, optimizing their interactions and reducing unnecessary ones.
Emerj Senior Editor Matthew DeMello recently sat down to talk with Michael Hitchcock, Director of Product Management, Accounting and Tax at Intuit, about how AI is transforming accounting for both business owners and accountants.
This article will examine three key insights from their conversation:
- Using a knowledge engine to increase accuracy: Using a knowledge engine to power a process they refer to as Guided Q & A to present simple questions to the customer to accurately identify complex transactions.
- Leveraging clustering models to expedite personalization: Expediting creation of personalized machine learning models for every customer through clustering models that identify patterns in the business’s transaction data.
- Building customer confidence into automation: Developing consumer trust in products by training machine learning models to identify the correct sales tax rates based on the company’s products and services.
Listen to the full episode below:
Guest: Michael Hitchcock, Director of Project Management, Intuit
Expertise: Product development, program management, accounting workflows, financial services, lean manufacturing, user experience
Brief Recognition: Michael began as a product manager at Intuit in 2014. Before that experience, he worked as a graduate teaching assistant at the University of Wisconsin-Madison and spent five years at General Electric in electronics design engineering.
Using a Knowledge Engine to Increase Accuracy
Hitchcock provided a thorough overview of how accounting software has changed since the 1990s; current-day accounting software still is based on manual record keeping, just as it was then. He efficiently summarized, “Accounting software has had very consistent workflow and mental models since its inception.”
He also explains how the emergence of accounting software in the ’90s simply recreated the concept of checks in a checkbook register allowing users to keep track of their finances on a computer.
Hitchcock provided insight into how online banking shifted the paradigm substantially by allowing transaction data to flow directly into the accounting product. The development was significant for managing expenses because it specifically automated the portion encompassing all of the record keeping that occurs before a transaction clears the bank.
While explaining how data flows into accounting software from online banking platforms, Hitchcock underscores that automation can make a critical difference in how accountants and other software users are required to sift through transactions individually and decide whether or not to add them to the ledger.
Small businesses and self-employed individuals are eager to automate this work. The main concern Quickbooks has is that they need to earn the trust of their customers in automation on day one. Hitchcock said Quickbooks solves this problem through an emphasis on their accuracy rate.
After all, he notes – their customers are busy, and even if they have a learning mindset, their time is limited, and acceptance of AI and automation doesn’t come easily without expertise. Customers would prefer to work on running and growing their businesses, not to mention that accounting and tax as a space is very high risk.
Quickbooks then introduced a process called guided Q&A to help customers get beyond a stumbling block with more complex transactions.
The feature uses a knowledge engine to surface simple questions for customers, allowing the complexities of double-entry bookkeeping to remain hidden. This feature is essential because complex transactions are problematic for users with limited accounting knowledge access.
Complex transactions also significantly impact the balance sheet and usually involve assets, liabilities, and equities. Michael tells Emerj that Quickbooks trained an AI model to identify complex transactions with high accuracy and built a workflow on top of that.
Hitchcock explains how their guided questions are similar to TurboTax, except they’re at the transaction level rather than the tax form level.
Quickbooks also tweaked the same Q&A process used for business owners to guide the accountants. Quickbooks identifies high-context transactions that might need more input from the business owner.
By queuing those high-context transactions, accountants can dive right in when they’re ready to do their work instead of contacting the small business owner for more information.
One crucial aspect is how Quickbooks measures ground truth for model training. They discovered a fundamental flaw in their system and realized they needed to be labeling data correctly.
Essentially, they were asking a user base who needs help understanding accounting in the first place to serve as ground truth for model training. Also, they failed to provide further weight to the feedback from accounting professionals on their platform more than the small businesses.
Since then, Michael tells Emerj the company is more pragmatic about training models. Subsequently, they’ve seen a massive improvement in the accuracy of their AI engine.
They accomplish this by a specific process to ensure they get the needed rich transaction data. Before a new model is introduced to the product, Quickbook guarantees:
- Offline testing of the model.
- The assistance of accountants to label the data.
Leveraging Clustering Models to Expedite Personalization
Micheal tells Emerj that to deliver accurate accounting from the outset, Quickbooks relies on a reserve of over 2 million personalized machine learning models specifically designed for accounting. They developed an experience called Train Quickbooks to address a problem they encountered when creating customized models for each customer.
Quickbooks initially discovered a problem when they launched the concept of a personalized model for every customer. The personalized model was taking too long to warm up when they attempted to switch from using the populational model that learned from all customers to personalized models that were tailored to each unique business.
The clustering model works by identifying patterns in the business’s transaction data. It does so as a result of the following:
- The customer first connects a bank/app
- Next, the customer answers 5 to 10 questions presented.
- A personalized model is built as a result.
As a result of the above workflow, the automation has enough accuracy to add a substantial number of transactions directly to the books without human intervention.
According to Michael, Quickbooks’ clustering model is partly built based on a customer’s response to the questions. The clustering model creates multiple clusters of transactions and also identifies a head transaction.
Hitchcock further explains how Quickbooks look for data that will be impactful. They start with simply basic firmographics and expand from there. Questions could include:
- What industry are you in?
- What is the benefit you’re looking for?
- Are you more focused on understanding profitability?
- Are you currently getting ready for tax time?
- What is your entity type?
- Are you product-based?
- Are you service-based?
- How do you get paid?
- What is the benefit you’re looking for?
- Are you more focused on understanding profitability?
- Are you currently getting ready for tax time?
Building Customer Confidence into Automation
Michael tells Emerj about how Quickbooks inspires customer confidence by ensuring customers know the product is doing everything it can to help them stay compliant. To that end, they implemented a feature called automated sales tax to inspire customer confidence.
He notes the complexity of the challenge, citing that there are 13,000 U.S. state and local tax jurisdictions, each with unique sales tax rates. To make tracking these rates easier, Michael tells Emerj that Quickbooks trained machine learning models to identify the correct sales tax rates based on the company’s products and services.
Hitchcock caveats that the ability to automate depends on the fidelity of data. Quickbooks wants to offer its customers the ability to connect their data feed and have the accounting done for them so that they can focus on running their businesses. High-quality data feeds are instrumental to being able to achieve that.
Following a question cited on the interviewee’s background in global taxes, Hitchcock recognizes the inherent subjectiveness in accounting. How a client wants to handle certain transactions will depend on what they’re trying to optimize for. In these cases, he tells Emerj that it’s essential to consider what benefits the customer is trying to achieve and what level of accuracy is needed to get a specific level of granularity.
The real benefit of addressing the accounting challenge of subjectivity is it enables businesses to move beyond simply meeting compliance requirements and achieving the ability to reach better business decisions.
Businesses can create accurate balance sheets that meet compliance requirements, but those reports might still lack the fidelity of data needed to make better business decisions.