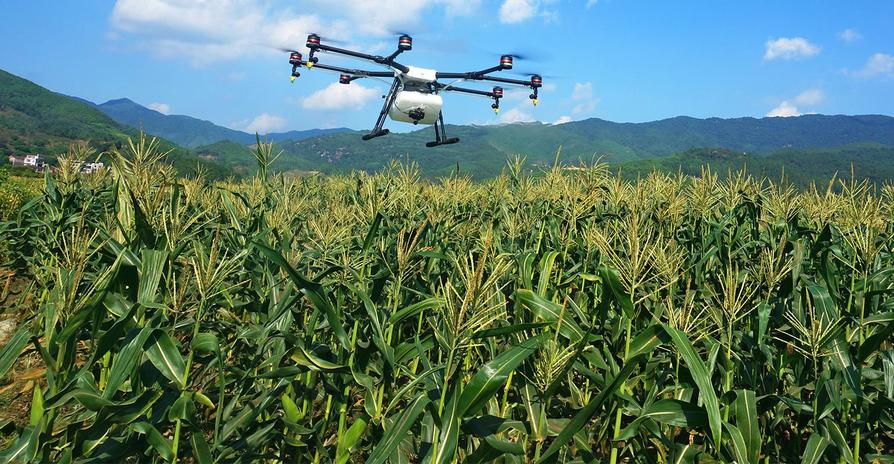
A recent PricewaterhouseCoopers study revealed that the global market for drone-powered business solutions was valued at $127.3 billion in 2016. For agriculture, prospective drone applications in global projects were valued at $32.4 billion.
The same study forecast that agricultural consumption would increase by 69% from 2010 to 2050 due to the predicted increase in population from 7 billion to 9 billion by 2050. To cope with demand, the agriculture industry may need to find ways to improve food production methods and increase harvest yield while staying sustainable and preventing environmental damage, the study suggested.
According to a report by the MIT Technology Review, drones in agriculture are used for soil and field analysis, planting, crop spraying, crop monitoring, irrigation, and health assessment. The report also explained that agriculture drones are more advanced data-gathering tools for serious professionals, compared with consumer-grade counterparts.
This article intends to provide business leaders in agriculture with an idea of what they can currently expect from AI-driven drones. We hope that this report allows business leaders to garner insights they can confidently relay to their executive teams to make informed decisions when thinking about AI adoption.
At the very least, this report intends to act as a method of reducing the time agriculture business leaders spend researching drone companies with whom they may (or may not) be interested in working.
(Readers with a more broad interest in drones might enjoy our full article on industrial drone applications.)
Drone Applications for Agriculture
Gamaya
Switzerland-based Gamaya offers a drone-mounted hyperspectral imaging camera, which the company claims combines remote sensing, machine learning, and crop science technologies. The camera may also be mounted on light aircraft.
The company explains that hyperspectral cameras measure the light reflected by plants. It claims to capture 40 bands of color within the visible and infrared light spectrum, 10 times more than other cameras which only capture four bands or color. The company also explains that plants with different physiologies and characteristics reflect light differently. This pattern changes as the plant grows and is affected by stressors.
The application behind the camera then uses machine learning to process the imaging data into information by comparing the captured images with those in its database and assigning specific conditions with a color. For instance, red shows soil deficiencies, blue is bare soil, white means there are weeds in the soil, green means there are weeds on the crop, black means healthy crops. This color coding system enables the system to create a map of the conditions of crops and the soil.
As explained in the video below, Gamaya’s technology is capable of mapping and distinguishing the weeds from plants. It is also able to identify other plant stresses such as disease and malnutrition, as well as chemical inputs in the soil.
The K Farm in Brazil turned to the Gamaya team in 2015 to fly over their fields over a 5-day period to give insights on its corn crops.
The farm was facing the following challenges:
- Strong difference in the performance of different corn varieties in one field. For instance, in the specified field Pioneer seeds work much better than Limagrain.
- Low-yield efficiency expected in several fields (in some more than 40% losses) due to diseases, weeds, and other crop issues.
- Nutrient deficiencies across the farm soil, potentially affecting production yield.
Based on the data captured by the camera, the algorithms found matches to the corn varieties in its database and helped the Gamaya team determine the different varieties and where they were planted in the field. This enabled them to correlate the good performance of each corn variety with its location in the field.
The algorithms displayed the crop performance by matching the level of crop efficiency with a particular color: green signifies no stress, yellow shows medium stress, orange is high stress, while red has no vegetation, as shown in the image below:
This enabled the team to understand which corn patches were growing healthily, abnormally, or were dying.
The Gamaya team was also able to produce chemical maps of the entire farm by comparing chemical data between the corn leaves they gathered and the spectral images.
The chemical maps gave the team and farm owners insight into the nutrient distribution across the farm, and helped the Gamaya make recommendations to match crop needs.
The maps enabled the Gamaya team to make the following recommendations to the farm owner:
- Distribute the corn seeds based on soil type and composition of nutrients in the soil.
- Regularly monitor crop growth and the presence of weeds and diseases to increase crop yield.
- Apply precise amounts of fertilizer to soil based on the crop needs and soil conditions.
The team forecast a 5% to 30% increase in the harvest of each plot at that current farm cycle if the recommendations were followed. Meanwhile, a total 10% yield increase for the entire farm was expected. No follow-up figures were provided after this project.
Gamaya also lists the Kayapo soybean farms in Brazil as among its clients. The company has raised $7.6 million in funding from investors ICOS Capital Management, VI Partners AG, Sandoz Foundation, Peter Letmathe, and Seed4Equity SA.
Dragos Constantin is the CTO at Gamaya. Prior to Gamaya, he served at Philips and Schlumberger-Doll Research. He holds a PhD in Computer Science and Remote Sensing from the Ecole Polytechnique Federale de Lausanne and a Master’s degree in Computer Vision from Karlsruhe Institute of Technology.
Neurala
Neutrala has developed the Neurala Brain, a deep learning application which the company claims requires less training, less data storage, and less computing resources.
This industry-agnostic application may be implemented in drones, cameras, smartphones, according to the company website. The company claims that its technology works with the NVidia TX1 GPU drone and captures five to eight frames per second.
Training the algorithms uses the company’s Brain Builder data processing tool which enables users to upload and label their own image sets. To start the training process, the user must upload to the device about 8 images per object. The company claims that Neurala’s algorithms will learn the in 25 seconds.
This is much faster than traditional deep neural networks (DNN) which take more than 15 hours to be trained on a server, and which require 36 images per object.
The company has no agriculture-related case study but explains how its AI-powered drone can be used to inspect cell towers, wind turbines, pipeline, and electrical infrastructure.
The company cites an interview with Zack Clark, Integration Specialist at Drone America, who says, “We inspect 300 miles of pipeline or 500 miles of utility line. A technician isn’t out there to instruct the drone operator on anything. The drones are out of range to feed real-time video back, and they are out of the line of sight for operators. Drones collect vast amounts of data, and this data needs to be reviewed and processed later to detect defects in the pipelines.”
The paper adds that the AI enables a drone to recognize the parts of a tower or line identify defects such as corroding or broken parts that need to be replaced or fixed. A human will still need to need to review parts of the videos or images captured by the AI, as seen in the video below:
The company claims Motorola as one of its clients, as well as one of its investors. $15.2 million in funding from investors that include Pelion Venture Partners, Sherpa Capital, Motorola Ventures, 360 Capital Partners, Draper Associates, SK Ventures, Idinvest Partners, and Ecomobility Ventures.
Anatoli Gorchetchnikov is the Co-Founder and CTO at Neurala. Prior to this, he was a research assistant professor at the Boston University, and Lead AI developer at Surfari. He holds several patents and has authored over 30 publications on neural networks. He has a PhD in Cognitive and Neural Systems from Boston University; and a Master’s degree in Computer Science.
Iris Automation
Iris Automation developed the Iris Collision Avoidance Technology for Commercial Drones, an application that allows drones to observe and interpret its surroundings and moving aircraft to avoid collision.
The company states that this application is suitable for use in agriculture, mining, oil and gas, and package delivery. Specific to agriculture, the company claims that the drone application is capable of assisting farmers in surveying crops, planting seeds, and controlling pests while interacting with other drones safely.
As seen in the video below, Iris Automation’s Collision Avoidance technology enables drones to identify, track, and avoid other aircraft when operating beyond visual line of sight:
The system’s computer vision gives it the ability to see obstacles, aircraft, and other potential dangers for a safe and reliable flight as it captures images of its surroundings during flight. Once the images are captured, the camera’s deep learning algorithms process the data by finding similar images in its database to recognize the object. Recognizing the objects allows the drone to then know where to fly.
The company does not list clients or case studies but announced that it partnered with Stratus Aeronautics Inc. and InDro Robotics Inc. to integrate its application into their drones.
Established in 2015, the company has raised $10 million in funding from 17 investors including, Bee Partners and Bessemer Venture Partners.
Alexander Harmsen is the Co-founder and CEO of Iris automation. He previously worked as an aircraft computer vision programmer at the NASA’s Jet Propulsion Laboratory. He was also Co-captain of the Unmanned Aircraft Systems, and flight software engineer at Matternet. He earned his Bachelor’s degree in Applied Science, Engineering Physics from the University of British Columbia.
Sensefly
SenseFly offers the Ag 360 computer vision drone, which captures infrared images of fields to help farm owners monitor crops at different stages of growth and assess the condition of the soil. This could enable farmers to keep track of plant health and determine the amount of fertilizer needed to be applied to avoid wastage.
As explained in the video below, the drone works in collaboration with the eMotion Ag software. Users first use this software to plan the drone’s flight path and monitor the drone in flight. The company claims that the can access airspace data and live weather updates to help plan and monitor the drone.
During the flight, the drone captures the imaging data of the fields while eMotion then directly uploads these images to cloud services. The Pix4Dfields image processing application generates aerial maps of fields for crop analysis. Its algorithms translate the imaging data to create maps of the field by finding matching images within its database to recognize the condition of the plants and soil. The company claims that this application was trained using input from farmers, agronomists, and breeders.
The maps enable farm owners to determine soil characteristics, such as temperature and moisture, and to determine what to put in the soil to improve crop growth. This information could then influence decisions in the next farm cycle to improve production.
The company claims the drone is capable of covering 200 hectares (500 acres) per flight at 120 meters (400 feet) above ground level.
The company also offers other agricultural drones with an optional Pix4Dfields software.
The company did not have a case study specific to the Ag 360 drone but features one highlighting the eBee Ag drone. Ocealia Group flew two SenseFly AI-equipped eBee Ag drones, which resulted in a 10% increased crop yield for farms in its group, according to the case study.
SenseFly’s other clients include the Golly Farms, French farmer Baptiste Bruggeman, farmer Joshua Ayinbora in Ghania, Château de Châtagneréaz wine producer in Switzerland, Drone by Drone, and AgSky Technologies.
SenseFly was founded in 2009 and is the commercial drone subsidiary of Parrot Group, acquired in 2012 for $5.16 million.
Raphael Zaugg is the Head of Research and Development at SenseFly. He obtained his Master’s degree in Mechatronics, Robotics and Automation Engineering from the Ecole Polytechnique Federale de Lausanne.
Takeaways for Business Leaders in Agriculture
Among the companies covered in this report, SenseFly seems to be the most viable due to its recent acquisition by Parrot Group. The company also seems to offer the most diverse product line, covering the most industries.
Using the data captured by AI-equipped drones and analyzed by collaborative software, farm owners are able to monitor crop growth and crop health and evaluate the condition of the soil. In turn, this information enables them to make decisions about managing weeds, diseases, and pests, as well as the amount of fertilizer and pesticides to apply to crops.
However, the information is only as good as the people who use it. The AI itself does not improve the yield. The agronomists who interpret the maps generated by the data can only provide recommendations to improve crop harvest. The farm owners have to apply to recommendations themselves.
From our research, it seems that operating the drones may be manageable by farm employees, but an agronomist may be necessary to interpret the data acquired by the drone.
Header Image Credit: Dakota Farmer