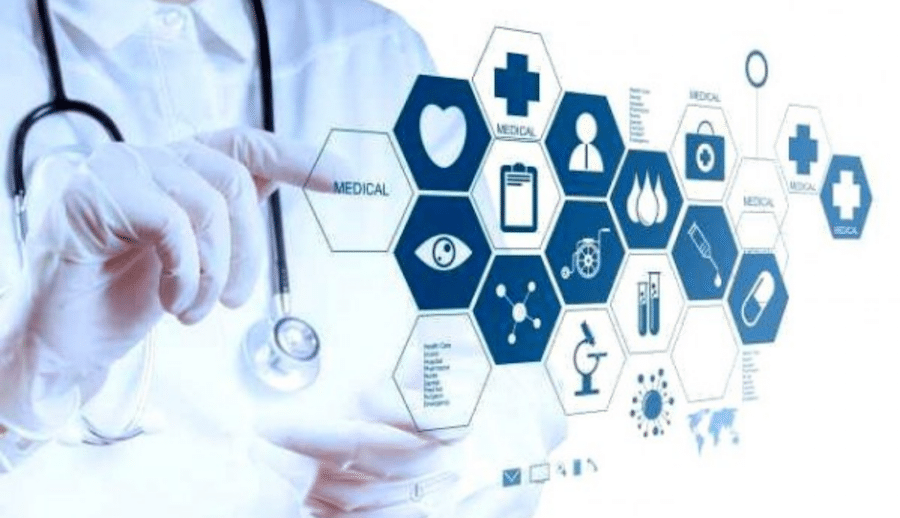
According to data from the U.S. Department of Health and Human Services, the progress of the value-based healthcare delivery system in the U.S. — a provider payment model based on patient outcomes — has run almost parallel to the significant implementation rate of electronic health records/electronic medical records (EHR/EMR).
Market research firm Research and Markets estimates that the EHR market will reach $33 billion by 2025. The firm anticipates that government initiatives to support EHR adoption will greatly influence this trend.
As the volume of patient data increases, some healthcare professionals believe the complexity of finding tools to extract insights could grow more challenging. Researchers are exploring how artificial intelligence can help draw useful information form massive and complex medical data sets.
This article will set out to determine the answers to the following questions:
- What types of AI applications are emerging to improve analyses of medical records?
- How is the healthcare market implementing these AI applications?
Medical Data Mining AI Applications Overview
Our research suggests that the majority of AI use cases and emerging applications for medical data mining appear to fall into three main categories:
- Predictive Analytics: When companies and healthcare professionals use machine learning to analyze patient data in order to determine possible patient outcomes, such as the likelihood of a worsening or improving health condition, or chances of inheriting an illness in an individual’s family.
- Diagnostic Analytics: Is defined by Gartner as “a form of advanced analytics which examines data or content” to determine why a health outcome happened.
- Prescriptive Analytics: When research firms develop machine learning algorithms to perform comprehensive analyses of patient data to improve the quality of patient management such as handling patient cases or coordinating the flow of tasks, such as ordering tests, among of medical personnel.
Below, we present representative examples from each category, as well as the current progress (funds raised, pilot applications, etc) of each example.
Predictive Analytics
Pieces Tech
Founded in 2015, Texas-based Pieces Tech claims that it leverages AI, machine learning and natural language processing to build its software platform to interpret patient data and recommend personalized treatment approaches.
Specifically, the cloud-based software platform, Pieces™ Decision Sciences (DS), uses algorithms trained on billions of data points to draw insights from patient data, improve quality of care and to lower costs by performing analyses throughout a patient’s journey, according to Pieces Tech.
When a user logs into the online platform, they can see a sidebar with a variety of different categories, including case management, patient enrollment and last encounter. After a health provider or health professional, such as a doctor, social worker, or transporter sees a patient, they can log into the platform, click into the case management category and search for a client. They can then see a client profile where they may view information on old and recent encounters, or notes or data from one specific doctor’s appointment or interaction with the health care professional.
In the 2-minute below, the company provides a demo of how a patient encounter would be entered into the company’s Iris platform.
According to the video, the data entered on the Iris platform can then work in collaboration with the AI-powered DS platform to evaluate clinical and social factors for social service providers, hospitals and health plans. The company claims it can also prevent new providers from adding the same data twice, in cases like ER visits.
The 2-minute video below further demonstrates how artificial intelligence is used with this platform:
Case studies are not currently available on the company’s website. Examples of clients include Children’s Health in Dallas, Texas and Parkview Medical Center based in Pueblo, Colorado.
To date, the company has raised $21.6 million in total funding. Chief Technology Officer, Rahav Dor, is a PhD in Computer Science who received his training at Washington University in St. Louis.
KenSci
Founded in 2015, Seattle-based startup KenSci reportedly uses machine learning to predict patient risks by analyzing clinical data. The company claims that its cloud-based software platform draws from a database of over 150 machine learning models using algorithms trained on over 10 million data sets which include clinical data from patient health records.
KenSci’s platform integrates with clinical systems such as electronic medical records (EMRs) so recommendations can be readily implemented by healthcare teams, according to the company. The company claims the software identifies patterns which may indicate potential risks by aggregating, cleaning and analyzing client data and provides predictive insights on healthcare outcomes.
Similarly to other simple analytics dashboards, healthcare providers or professionals can log into the online platform in search of various group statistics such as cost of procedures based on certain populations or geographies; or average number of readmissions for various health conditions. After entering data such as location or a health condition, the site will show the healthcare professional a chart and figures based on their search parameters. The user can than print or share these results with colleagues.
The 4-minute demo below provides examples of how KenSci’s Clinical Analytics solution may help reduce the total cost impact of sepsis and Hospital-Acquired Conditions (HAC) by helping to identify patterns and predict patients who are at high risk:
According to its website, the platform can deliver ROI to clients in 12 weeks and is providing predictive analytics on 17 million patients.
In a case study the company claims that it helped the US Army Medical Corps avoid 1,666 patient readmissions over four years and to save $33 million per facility within the same time period. Previously, nurses used a 23-year old program just to log data. The case study states that the useage of KenSci’s software saved the user on average 11 clicks when logging data.
KenSci’s investors include Microsoft through its Microsoft Ventures Accelerator in Seattle.
Diagnostic Analytics
Prognos
Founded in 2010, New York-based Prognos claims that it uses machine learning to run its software which claims to analyze electronic medical records from various hospitals and healthcare systems.
The company says that its database of clinical diagnostics information includes data for 50 diseases and its 1,000 algorithms are trained to analyze over 14 billion medical records for 180 million patients.
The company claims its algorithms enable the platform to identify patients earlier who may require enhanced treatment options, in addition to improving overall risk management and quality of operations.
The company states that the platform can also provide pharmaceutical clients with weekly alerts when newly diagnosed patients have been identified to help optimize the marketing and sales strategies. Examples of clients and collaborators include Biogen, QuestDiagnostics and LabCorp.
To date, the company has raised $42.3 million in total funding and lead investors include the Merck Global Health Innovation Fund and Cigna.
“We have seen Prognos’ capabilities first-hand and believe health plans will greatly benefit from integrating real-time lab and diagnostics data intelligence to refine their approaches to risk adjustment, clinical quality, and care management.” – Craig Cimini, VP Strategy and Business Development at Cigna (November 2017).
According the company’s LinkedIn page, Fernando Schwartz, a PhD in mathematics who trained at Cornell University and Stanford University, is listed as Prognos’ Chief Data Scientist and Head of AI.
Case studies and a demonstration video for this software could not be found.
Prescriptive Analytics
CareSkore
Founded in 2014, California-based CareSkore claims that it leverages machine learning to generate predictive and prescriptive analytics to help healthcare providers implement personalized patient care management.
The platform, which the company claims can integrate with various existing patient management platforms, gathers a combination of data including clinical information, insurance claims and demographics for each patient from electronic medical records. Once a profile is generated for the patient, and a healthcare provider logs into their existing patient management platform, the CareSkore’s integration can alert the professionals about patients who appear to be a higher risk for factors that may disaffect their care. Examples of factors include infections and medication adherence issues. This information can be used by the clinician to attempt to prevent these risks before they occur.
The company’s machine learning analytics engine is called ZEUS, and its algorithms are reportedly trained on 4.3 billion data points from more than 42 million patients. These data sets represent the framework from which personalized patient care plans are developed.
The video below depicts how CareSkore integrates multiple data sources into its machine learning and AI-driven patient engagement platforms.
The company’s website claims that its platform achieves 90-percent accuracy compared to an average range from 60 to 70 percent for traditional analytics solutions.
No use cases are available on CareSkore’s website, however, Chicago Medical School has noted that it is a client:
“CareSkore has dramatically simplified the implementation of advanced patient-specific analytics…access through and integration with existing EHR and other apps significantly lowers the barriers to adoption and deployment.” -Rohit Arora, MD, Professor and Chairman of Cardiovascular Medicine, Chicago Medical School (February 2017)
The company has raised $4.4 million to date and its Senior Data Scientist, Mehrdad Nouralishahi, is a PhD who received his training from UCLA in Electrical Engineering/Optimization.
Roam Analytics
Founded in 2013, with headquarters in California, Roam Analytics claims that it leverages machine learning to deliver its web-based healthcare data analytics platform.
Roam says the algorithms driving the platform draw on thousands of patient data points, such as electronic medical record data from various healthcare organizations. The company also claims to collect unstructured data, defined as “information that is documented without standard content specifications, often recorded as free text.”
While instructions on how to use the platform were not available through a demo video or on its site, we have found from our research that a healthprovider would log into the platform and link data from existing EMRs. After EMR information is integrated from a health organization, the platform can present “actionable insights” about each patient. According to its site, this could include treatment suggestions or tests that a doctor may want to run based on comments made by a patient in an appointment.
The Agency for Healthcare Research and Quality has identified unstructured data as a major barrier to quality measurement efforts. Use cases, a video demonstration and client examples could not be found in our research.
In the 6-minute below, Alex Turkeltaub, Roam CEO and co-founder, provides an overview of his company, addresses the barriers in synthesizing actionable insights from unstructured health data and discusses challenges around consumer data.
To date, Roam Analytics has reportedly raised $21.9 million in total funding. Co-Founder and Chief Scientist, Andrew Maas, earned his PhD in Computer Science from Stanford University. He received his B.S. from Carnegie Mellon University in Computer Science and Cognitive Science. No demonstration video or instructions for use could be found on Roam’s Youtube account or online.
Concluding Thoughts
Among current and emerging applications in the medical record data mining industry, our research finds that machine learning applications show a trend. While the general objectives of these platforms are mostly similar, to gain useful insights from medical data to improve patient outcomes, there are slight differences worth highlighting.
Predictive analytics solutions appear to be the largest category for use-cases. This may be ideal territory for machine learning applications due to the large amount of medical data that leading companies are able to access through partnerships with healthcare institutions as in the example of KenSci.
Similar to recommendation engines, predictive analytics platforms appear to deliver trends that are proving meaningful to healthcare systems and investors in the healthcare space. With the oldest company profiled in this article, Prognos, founded in 2010, the applications noted seem to be in the early stages.
With Pieces Tech, we could see an increased trend in the integration of both clinical and non-clinical data. The effort to demonstrate value-based care could introduce more innovative, technology-based approaches in the coming year. As a result, automated software solutions capable of interpreting patient data and the multiple other data types that influence patient outcomes will be very useful.
In our research of diagnostic analytics platform we found fewer examples, which is in strong contrast to the high volume of chatbot applications that focus on diagnostics solutions. It could be that the idea of an analytics platform providing diagnostic recommendations may feel less intuitive compared to a chatbot designed to provide diagnostic support at the point-of-care.
More time and research will be needed to determine if diagnostic analytics AI applications gain greater traction in the healthcare industry. In comparison, prescriptive analytics applications which are very similar to predictive analytics with slight variation, appear to be tackling some important challenges faced by providers and healthcare systems.
For example, Roam Analytics is tackling unstructured data which has traditionally been a harder category of data to analyze and interpret. However, AI approaches appear to be offering new possibilities in gathering valuable information from these data sources.
While healthcare delivery in the US may continue to explore and implement technology-based solutions, value-based healthcare will also necessitate efficient and well-organized uses of these technology. Research shows that an estimated $17.2 billion in incentives has been allocated for the “adoption and meaningful use of health information technology, part of which involves the participation in the electronic exchange of clinical information.”
Header Image Credit: BetaNews