Over the last 3-4 years, sentiment analysis has become a more and more common term – understood by marketers and businesspeople alike. The idea is simple: An artificial intelligence system that can detect the emotional “tone” or sentiment of a specific text document (as long as a book, or as short as a tweet).
What many business people don’t know, however, is that there is no single “sentiment analysis” program that works in all circumstances or for all use-cases. Initially, sentiment analysis systems have to be trained on the terms, language, and context in which they’ll be used – and that’s a job for humans.
I spoke with Charly Walther, VP of Product and Growth at Gengo.ai, the crowdsourced AI training arm of Gengo.com – a web-based human translation platform headquartered in Tokyo. I asked Charly about specific circumstances where crowdsourced human perception is necessary to train a sentiment system well.
Charly previously worked at Uber where he worked on self-driving cars. He worked on improving the algorithms for self-driving cars, and then briefly worked in the data labelling efforts at Uber – which involved crowdsourced manual tagging of image data for training machine vision systems.
In the article below, we’ll explore the situations where crowdsourcing is a necessary part of training a sentiment analysis system – and we’ll also examine some representative crowdsourced sentiment labelling use cases from various industries and applications.
When Crowdsourcing is Necessary for Sentiment Analysis
In some domains, the process of sentiment analysis has more-or-less already been solved. If you want to know if a given Twitter message (tweet) is angry or happy, there’s probably a blanket solution to make that determination (so long as the tweets are in English). Many companies have already trained systems on basic social media sentiment detection in English.
Therefore crowdsourcing becomes necessary when:
- A language is being analyzed that hasn’t previously been analyzed at scale (for example, a company aiming to create a social media sentiment analysis solution for Greek or Hebrew)
- A topic being analyzed is unique (maybe a company wants to analyze the sentiment of very complicated and robust articles and essays about politics in the Middle East)
In the cases above, unique terms and entities and words need to be labelled and analyzed en masse in order to get a machine to make sense of them well. This often requires native speakers of the language in question, and it could also involve some degree of contextual knowledge about the domain (in the case of middle eastern politics).
Some data already comes with proxies for sentiment already. For example, Amazon reviews come along with text, as well as a 1-to-5 star review. These stars can be used as a proxy for general sentiment (good or bad), and so it might be less necessary to have humans score sentiment from scratch. In Charly’s words:
“A model needs accurately labelled data in order to detect sentiment well. That data is not necessarily there for most companies. If you’re Amazon, you get a bit of context on your text reviews because you can guess that the words used with a five-star rating is probably positive sentiment, and the text along with a one-star rating is probably negative sentiment.
As a very first step, you need to establish the ground truth data here. The reason you need crowdsourcing is because as soon as you need a certain context or language, then it will be tough to find the right people to label the data.”
Twitter data (tweets) for example – doesn’t come along with any strong cues or proxies for sentiment. Nor do most customer service emails, or call center transcripts. This data must be either scored by an AI system, or understood manually by a human being with an understanding of both the context and the language.
Crowdsourcing for Sentiment Data – When it’s Needed
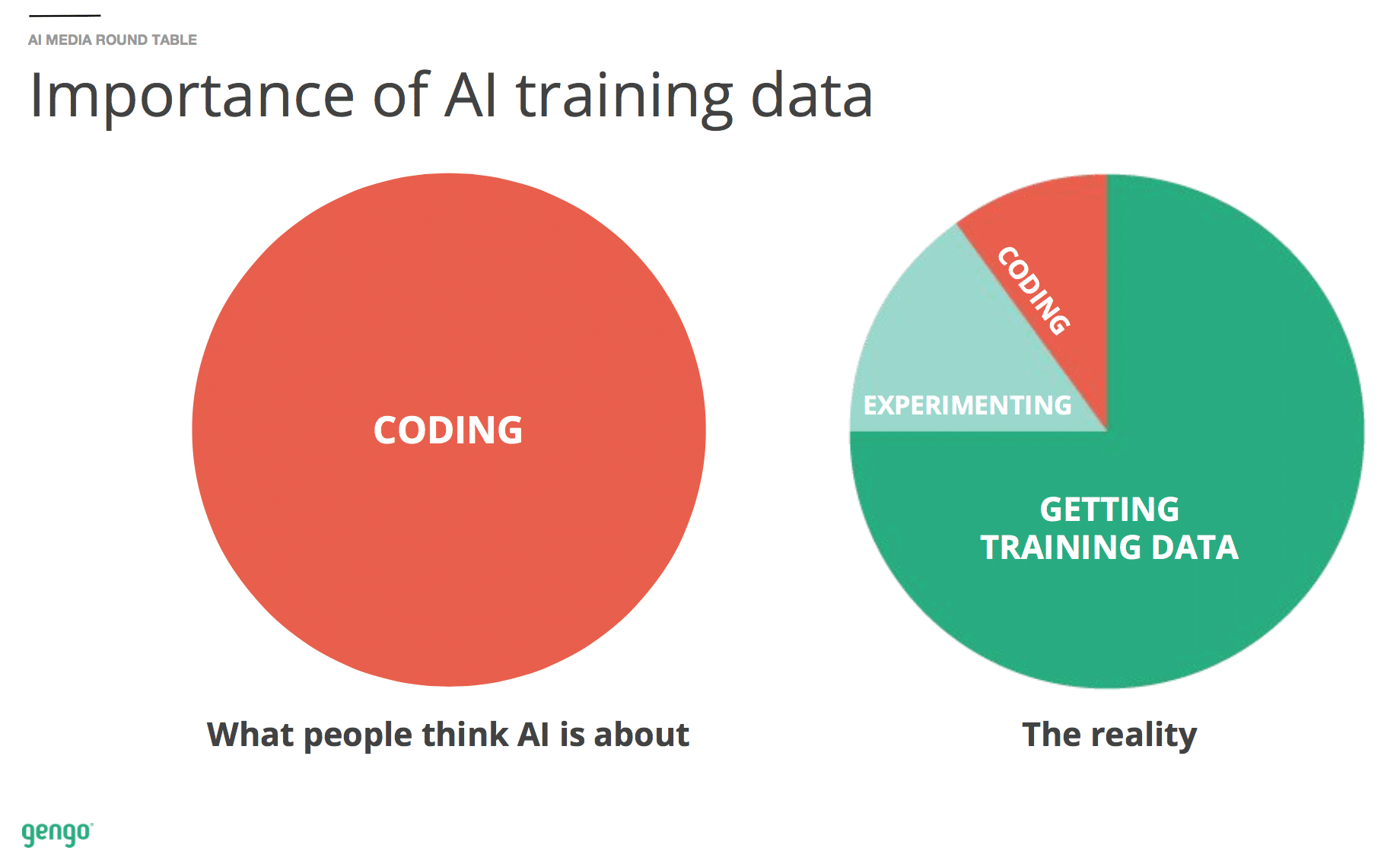
Charly explained the kinds of buyers of crowdsourced sentiment analysis services well:
- First, there are artificial intelligence companies who need to build a large tagged corpus of data in order to train an initial algorithm to master a certain domain. For example, a company striving to become a dominant sentiment analysis vendor for financial statements and public financial records might need to have thousands and thousands of such documents manually scored and labeled by humans – allowing the firm to train their algorithms on these examples.
- Second, there are companies who simply want to understand sentiment for their own business purposes, without repackaging those lessons as a product of some kind. For example, a marketing head for a professional sports team might want to have an ongoing insight into the kind of social media sentiment about his or her team – and cross-reference that data with merchandise sales.
In the first case (AI vendors), the initial volumes of labeled data needed would be rather massive – but once the AI system is trained and performing well – the firm would require a much smaller ongoing stream of data in order to continuously update the system and keep it up-to-date on new terms, trends, or edge-cases.
In the second data, the labelling needs would likely remain more stable because no system is being developed to automate the sentiment analysis process.
Below, we’ll examine some of the use cases when crowdsourcing might be required to get a sentiment analysis up and running:
Sentiment Analysis in Travel and Hospitality
A company handling resort and hotel bookings in southeast Asia may want to understand the sentiment of it’s customer requests – including everything from sales inquiries to customer service requests. The firm may want to analyze not only email messages, but also text messages, social media posts and call center transcriptions.
Developing a specialized system for sentiment analysis would likely require:
- Native speakers of southeast Asian languages, such as Burmese (Myanmar), Indonesian, Khmer (Cambodian) Malay, and Thai
- These people would need to label various customer communications with a variety of emotions, taking into account the regional terms and idioms of the language
The business value of a system of this kind could be:
- To better understand how various sales or customer service requests were being received by customers and website users
- To determine differences in customer perception and customer service levels in different countries and locations
- To detect sentiment trends, understanding which services or issues seem to be getting better or worse in terms of customer perception
- To help an executive team better prioritize website updates or employee training initiatives, focusing on those initiatives most likely to positively affect end users
Dr. Charles Martin has built machine learning applications for a number of global banks, and spent years at Active Equities Group and Black Rock. He provided an anecdote about the importance of training data relevance – a point that any business leader building a sentiment analysis system should bear in mind:
“In the financial markets people might be asked to label data. But if you ask: ‘What would a trader think?’, that’s a different question.
We worked on cases at a large investment management firm and they wanted to understand the sentiment or meaning of SEC filings. The company was looking to have untrained mechanical turks read and interpret the filings. The problem is that these people didn’t understand SEC filings and didn’t understand the nuance and the context.
When a person sees a company being sued, they often assume that the company did something wrong and that’s a negative. When a trader sees a company being sued, they might see it as a good thing because the company is pushing the boundaries of what’s possible.”
The important point here, Charles says, is to make sure that the training data matches the kind of data that will enter a system when it’s in use. If a system needs to be used by experts to make informed decisions, the training needs to reflect some semblance of that same expertise and context. In his words:
“There’s a huge difference between deciding if somebody likes a movie, or if a certain food tastes good, versus some kind of complex technical problem. If your problem (even if it’s determining sentiment) requires domain knowledge, then you can’t expect average people without that experience to be the best way to train a system.”
Sentiment Analysis in Customer Service
A company aims to build a sentiment analysis system for telecommunications vendors in South America. This company might need to train their initial sentiment analysis system on specific telecommunications-related customer service issues – and across multiple dialects of Spanish, Portuguese, and English.
If this task were applied to email communications for telecom companies, it might require:
- Native speakers of Spanish, Portuguese, and English
- People familiar with various complaints and issues in order to identify which issues tied to which specific customer service email messages (refund requests, cancellations due to a move, requests to upgrade a service, etc)
Expressions of frustration or sarcasm or anger might be articulated differently in Peru than in Rio de Janeiro, and the terms and idioms used in those regions might vary wildly, requiring local human discernment to coax out those unique situations.
A system with these more robust distinctions – and a focus on telecom customer service requests – could potentially be much more accurate in sentiment analysis than a general sentiment system for Spanish and Portuguese.
The business value of a system of this kind could be:
- To train a system capable of properly assessing the sentiment of nearly any customer service telecom request in Spanish or Portuguese, and to sell this system to other telecom providers
- To solve the challenges of local nuance and provide better sentiment feedback than other solutions on the market
Sentiment Analysis in Media
An online media company looking to make sense of Twitter sentiment around political issues in the Middle East, they would need to analyze information well beyond simply pumping an existing dataset into an existing sentiment model. A project of this kind would require:
- Native speakers of various Middle Eastern languages (Arabic, Farsi, etc)
- These people would need to be familiar with political issues in order to:
- “Flag” or identify tweets that are about political issues (this can be much more nuanced than simply looking for Arabic keywords that involve politics, it would often involve an “understanding” of the tweet)
- Identify and label the “entities” that are specific to this topic (current political leaders and candidates, politically charged locations, crucial political issues, and more)
Charly says:
“We do a lot of sentiment analysis based off of Twitter… but we often find the tweets in the first place. The customer just gives us the topic – say arabic politics – and we just find the tweets that fit that topic, as well as the sentiment analysis.”
Indeed it’s human judgment that’s needed to determine (a) if a tweet is about Arabic political issues, (b) which entities (people or places or issues) are being discussed, and (c) which emotions – or combination of emotions – are likely being expressed. None of that is evident or pre-labeled in Twitter, it must be parsed out by people – at least for long enough to train a specialized AI system to do the job.
Getting such a task off the ground would be rather challenging without crowdsource labor. Building such a team of data handling specialists within a given country is no easy task, and is in no way worth the effort for a single such project.
The business value of a system of this kind could be:
- Determining which media issues are most emotionally charged and interesting for readers in real time
- Determining which “entities” (political figures, cities) are of the greatest interest to the media company’s readers
- Making more informed predictions about the results of elections or political rulings based on sentiment trends
Crowdsourcing to Solve the Language Problem
The issue of language is particularly challenging for companies that have to expand to many new locations within a relatively short period of time – or for companies operating in unique or niche geographical markets.
In nearly all of the examples highlighted above, language challenges are one of the most important reasons to train a sentiment system, as opposed to using a general pre-built system.
Gengo boasts Amazon and Facebook among their clients, and Charly tells me that this is because – while these tech giants could potentially collect and label this data themselves – they often prefer to partner with a global firm like Gengo to scale their data labelling as they enter a new market with a new language.
Speed to market is important, and even tech giants use crowdsourced labor to move into new markets. Crowdsourced firms with contractors around the globe allows for the training of algorithms for specific geographical, cultural, or language peculiarities.
About Gengo.ai
Gengo.ai offers high-quality, multilingual, crowdsourced data services for training machine learning models. The firm boasts tens of thousands of crowdsourced workers around the globe, servicing the likes of technology giants such as Expedia, Facebook, Amazon, and more.
This article was sponsored by Gengo.ai, and was written, edited and published in alignment with our transparent Emerj sponsored content guidelines. Learn more about reaching our AI-focused executive audience on our Emerj advertising page.
Header image credit: Salesforce