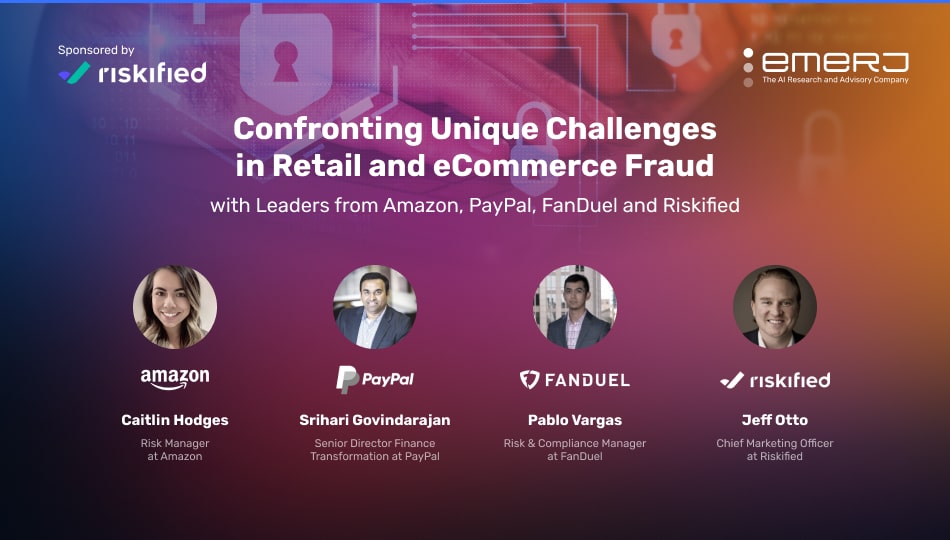
This interview analysis is sponsored by Riskified and was written, edited and published in alignment with our Emerj sponsored content guidelines. Learn more about our thought leadership and content creation services on our Emerj Media Services page.
Policy abuse and fraud are pervasive challenges in retail and eCommerce, significantly impacting businesses’ bottom lines. Policy abuse encompasses a range of behaviors, from outright fraud to exploiting return and refund policies for personal gain. It includes friendly fraud, return fraud, and other forms of abuse.
According to the recent National Retail Federation report “2023 Consumer Returns in the Retail Industry“, policy abuse and fraud impacted 13.7% of overall retail returns last year, totaling USD 743 billion alone.
Activities such as using different emails to take advantage of new member discounts may seem like harmless tactics for maximizing the customer experience to many. However, duplicate accounts and other fraudulent practices tally up to USD 100 Billion in retail losses annually, according to a widely cited new report from the eCommerce fraud solution provider Riskified.
In the following analysis of conversations with leaders on Emerj’s ‘AI in Business’ podcast, we provide a closer look at the challenges their companies face when fighting bad actors and fraud.
The conversations highlighted below are part of a sponsored podcast series focusing on policy abuse fraud in retail and eCommerce sectors. Executives interviewed in the series include Jeff Otto, Chief Marketing Officer of Riskified; Srihari Govindarajan, Senior Director of Finance Transformation at PayPal; Pablo Vargas, Enterprise Risk & Compliance Manager at FanDuel; and Caitlin Hodges, Risk Manager at Amazon.
Each guest brings distinct industry experiences and perspectives to each conversation, particularly on how they see potential data tools and applications as underutilized in the fight against retail fraud in their respective spaces.
This article synthesizes these conversations into four critical insights for retail leaders:
- Leveraging AI to recognize patterns of abuse: Recognizing patterns of abuse in claims and promotions and dynamically adjusting trust levels with the help of machine learning to mitigate financial losses and enhance security against fraud.
- Enhancing financial security for fraud prevention: Integrating advanced language models to preemptively identify and handle potential fraud, securing sensitive customer information through frameworks.
- Strategies for working around challenging regulatory environments: Lessons from the gaming industry in why data quality and responsible data management practices are essential for navigating complex partner relationships, particularly around compliance.
- Streamlining redundant tasks: Leveraging machine learning tools to filter out and cancel fraudulent accounts and activities efficiently.
Looking at Refund and Loyalty Programs in Retail From a Data Perspective – with Jeff Otto of Riskified
Episode 1 – Looking at Refund and Loyalty Programs in Retail From a Data Perspective
Guest: Jeff Otto, Chief Marketing Officer, Riskified
Expertise: Marketing Strategy, Product Marketing, Strategy Development
As Riskified Chief Marketing Officer, Jeff begins his podcast appearance by explaining the specific abuses that merchants face, particularly in claims related to items not received and the exploitation of loyalty programs.
In the case of an ‘item not received’ claim (“INR”), he acknowledges that many such claims are legitimate. However, he highlights a pervasive issue of abuse where a significant number of individuals engage in fraudulent claims, causing financial strain on merchants.
To illustrate the impact, Jeff provides a specific example of promotion and loyalty program abuse ripe for the application of data tools. He recounts a scenario involving a major online retailer in the home goods space:
“After analyzing a couple quarters of their order data, we were able to find that there was this cohort of about 4,000 real people. We could resolve their identities through machine learning. They had created 137,000 accounts, and they were using that to get this first-time customer discount for auto shipping and 50%.”
– Jeff Otto, Chief Marketing Officer, Riskified
Given the retailer’s gross margin of 28%, Jeff emphasizes that this cohort’s actions were causing substantial financial losses for the merchant, as they were essentially losing money on each transaction involving these fraudulent accounts.
Jeff then discusses the challenge of identifying the true identity of individuals engaging in various forms of abuse, ranging from professional fraudsters to serial- and friendly abusers. The goal is to validate the identity of the person behind the keyboard.
He highlights the broad spectrum of abuse, with professional fraudsters actively sharing tactics on the dark web while serial abusers exploit multiple accounts for personal gain. Jeff notes that the largest cohort often consists of friendly abusers or individuals who unknowingly contribute to merchants’ losses by frequently returning items.
Jeff explains the approach to handling individuals with a history of questionable activities, leveraging a comprehensive dataset spanning ten years of fraud data and two years of claims data within Riskified’s merchant network.
Instead of outright denying the return service to the customer, the strategy involves dynamically adjusting the level of trust. For individuals with mixed purchase histories, the system may introduce a moderate level of friction, such as specifying final sales or encouraging in-store returns for verification.
Enhancing Financial Security for Fraud Prevention
Episode 2 – Generative AI Approaches for eCommerce and Retail Fraud
Guest: Srihari Govindarajan, Senior Director of Finance Transformation at PayPal
Expertise: Finance Transformation, Advanced Analytics, AI Strategy & Execution
Sri begins his podcast appearance by discussing ongoing efforts at PayPal to combat eCommerce fraud and highlights preemptive measures implemented through risk toolkits. The PayPal team has employed a version of the popular GPT model called “fraud GPT” as part of their internal engineering initiatives. This toolkit leverages large language models, like Open AI’s GPT-4, to build risk identification and handling layers to address potential fraud preemptively.
Here, Sri emphasizes the importance of securing sensitive information, such as customer VAT and tax details, by applying frameworks to ensure data security. Additionally, the team focuses on know-your-customer (KYC) processes, capturing necessary information and promptly reporting fraud indicators, especially when dealing with cross-border transactions involving multiple currencies.
An illustrative example involves monitoring multi-currency transactions, where potential fraud indicators are checked, including the accurate processing of exchange rates through a service layer to prevent fraudulent activities in international sales.
Sri outlines the risk-centric approach adopted in their budgeting, planning and forecasting processes called risk-based forecasting. It entails incorporating a risk framework into financial data analysis and exploring multiple scenarios during forecasting, including risk factors derived from external global influences.
He explains to listeners PayPal’s process for evaluating how regulatory and reporting risks are managed, specifying his team scrutinizes transaction data for revenue considerations. The overarching goal is loss reduction, achieved through a metric that Sri refers to as ‘risk loss and revenue enablement.’ He explains that measuring these outcomes in such a metric involves leveraging digital identity insights to minimize losses and eventually offering risk-related services.
Specifically, PayPal provides services for chargeback protection and foreign exchange services, providing greater monetization enablement for their cross-border merchants. The emphasis on risk-based forecasting and loss reduction showcases a strategic alignment between risk management and financial forecasting for more informed and secure financial planning.
“If any indicators of fraud are appearing, we report it immediately. To give you an example, we do a lot of what we call ‘trading across the borders.’ So let’s say you have a merchant who sells in the US, and the merchandise is sold in dollars, but then you have a customer who’s an online purchaser or sitting in Brazil. We allow them what we call a ‘multi-currency transaction’ where they can purchase in their local currency, but then there are FX applied to this.
In this transaction, there is also a potential for fraud that we usually elevate. Then, we preemptively check and see that my effects processing is done right. And if it is, the rate that is being applied also goes through the risk layer to ensure that there is no fraud happening.”
– Srihari Govindarajan, Senior Director of Finance Transformation at PayPal
Strategies for Working Around Difficult Regulatory Environments
Episode 3 – Fraud Challenges in the Gaming Industry from a Data Perspective
Guest: Pablo Vargas, Enterprise Risk & Compliance Manager, FanDuel
Expertise: SAS, Data Analysis, Risk Management, Regulatory Compliance
Pablo spends much of his podcast appearance discussing the regulatory complexities of the gaming industry, especially as it grows in the United States. Throughout, listeners understand the importance of data in retail environments with multitudinous rules, stakeholders, and interests.
The sector involves interactions between gaming vendors, venues (i.e., stadiums, casinos, and other brick-and-mortar establishments), and governments, creating a situation where different entities speak different languages, leading to misunderstandings and inefficiencies. Pablo, therefore, highlights the need for a collaborative approach where all stakeholders come together to discuss challenges and create fair regulations.
He further points out that the inefficiencies in the gaming industry are still quite remarkable even in light of these challenges, especially compared to more established sectors like finance and telecommunications.
Pablo also discusses the aspirations of the gaming industry, highlighting the ideal scenario where they have comprehensive information to detect patterns related to responsible gaming. In this “dream state,” the industry aims to identify customers, ensuring sustainability and reliability for years to come.
Gaming publishers are increasingly targeted by criminals, leading to data breaches, identity theft, and other fraudulent activities.
For instance, gamers can be targeted through spyware schemes, and hackers can attack game publishers directly, stealing sensitive information. The lack of fraud prevention measures poses a severe threat to the gaming industry, raising questions about the potential consequences of inadequate safeguards.
“A customer walking into a retail location, placing a $10 bet on anything requires zero information. So we don’t know who the customer is. We don’t know what the source of funds is. We don’t know anything. So what we want is everyone to go through online.
Now, to your point: Do we want all of the information that’s possible? Yes, but we want good, clean data. That is part of the challenge right now. It’s also: How long has the game in this industry been around? Not very long, so you get a lot of smart business people to come together to create this industry. But half of them don’t really come from the background of data analytics and the importance of data quality assurance. So it does get a little muddy, and we’re playing catch up almost when it comes to this data acquisition.”
– Pablo Vargas, Risk Manager & Compliance Manager at FanDuel
He highlights the complexities of using, gathering, and ensuring the quality of data, especially when dealing with customers and partners in a brick-and-mortar space. Pablo feels there are a lot of dynamics that make data gathering a challenging process for any organization. He recommends that other retail leaders in similar situations ask themselves the following questions to understand better how these dynamics are impacting their organizations:
- What data is available and what is not?
- Is it clean or not?
- How can we improve the processes?
- Can we trust the data?
- What data can we get without impeding the customers?
- How do we reward the customers for sharing their data without making it look like we encourage them to play more?
The comparison is drawn to alcohol commercials that portray enjoyable experiences but include a responsible drinking message. Similarly, in gaming, there is a fine line between encouraging engagement and ensuring responsible gaming practices.
Pablo articulates the industry’s desire to strike this balance and create a sustainable model that aligns with regulatory expectations and societal considerations.
In the end, he discusses the adaptability of machine learning, emphasizing the importance of human guidance for practical application. Acknowledging that no model works indefinitely, Pablo suggests that intelligent individuals familiar with the industry and its patterns will be crucial to success in the model development process.
Using the example of post-pandemic fraud detection, Pablo illustrates the need for understanding nuanced behavioral changes, particularly when anticipating increased instances of third-party fraud as governments began distributing stimulus checks.
Streamlining Redundant Tasks
Episode 4 – Evolving Approaches to Competitor and Policy Fraud with AI
Guest: Caitlin Hodges, Risk Manager, Amazon
Expertise: Business analytics, Fraud investigation, Fraud analysis
Caitlin uses her platform on the ‘AI in Business’ podcast to shed light on the challenges sellers face on Amazon, specifically focusing on instances of what Caitlin and her team refer to as competitor fraud. One particularly potent form of competitor fraud is writing damaging reviews or resorting to threatening messages to hinder another seller’s listings.
Another significant concern is an abuse of inventory holds, involving bots and AI to create fake accounts, make purchases, and swiftly cancel them. Such manipulation leads to list holds, making sellers appear unavailable, incurring fees, and affecting their ability to sell items.
Caitlin acknowledges the severity of these challenges, noting that legal involvement sometimes becomes necessary.
To address these issues, Caitlin emphasizes the importance of implementing preventive measures against abusive behaviors from her experience. The more proactive approach not only safeguards sellers from financial losses but also contributes to the overall efficiency of the Amazon platform.
She also describes the prevalence of machine learning exploitation in fraudulent activities on the Amazon platform. Instances include using stolen credit cards and bots to create seemingly legitimate accounts for testing purposes.
The sheer volume of such activities makes it challenging for individuals to address them manually. Consequently, she emphasizes the necessity of leveraging machine learning tools to efficiently filter out and cancel fraudulent accounts, underscoring the crucial role of automated solutions in dealing with the scale of fraudulent activities.
She expresses the current utilization of AI in handling routine tasks or “grunt work” in her field. The ultimate goal is for AI to evolve to a point where it can autonomously manage and enforce actions against fraudulent or abusive behaviors. However, Caitlin points out a critical challenge: the accuracy of AI capabilities, particularly new generative iterations of these technologies at their current stage of development.
While AI systems are functional, their assessments still have a significant margin of error. She emphasizes the ongoing effort to refine and train interlocking AI models and enterprise functions to better differentiate between legitimate and fraudulent activities. Throughout the process, Caitlin acknowledges the ideal goal of achieving 100% accuracy in identifying and addressing such issues:
“So our department’s doing the grunt work. Then, they’re doing SQL query, and they’re saying, ‘Okay, well, we found this one. This one character that has an email address pattern.’ Then, they were able to run a query to pull that information rapidly. Then, we run those sellers through their machine learning to see if they are potentially fraudulent. To have that data grill itself is our future goal.”
– Caitlin Hodges, Risk Manager, Amazon