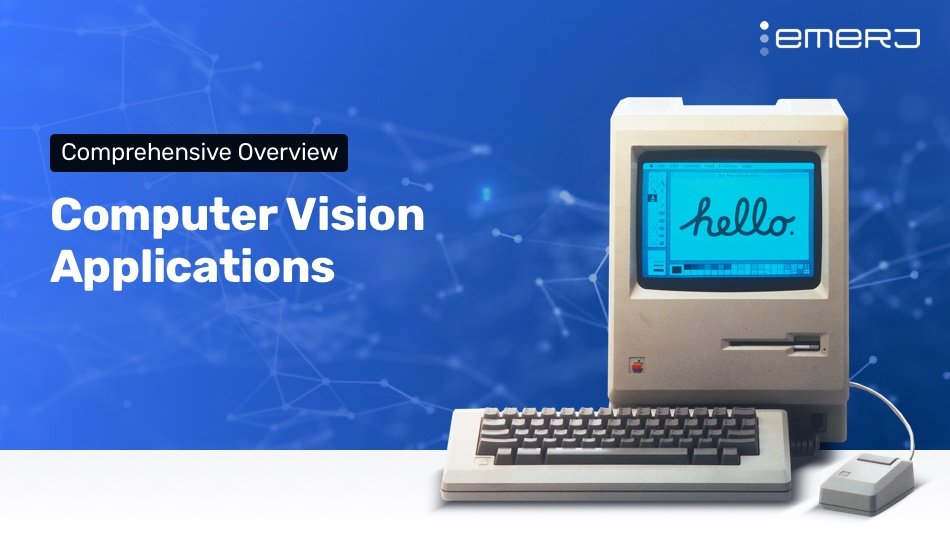
Computer vision, an AI technology that allows computers to understand and label images, is now used in convenience stores, driverless car testing, daily medical diagnostics, and in monitoring the health of crops and livestock.
From our research, we have seen that computers are proficient at recognizing images. Today, top technology companies such as Amazon, Google, Microsoft, and Facebook are investing billions of dollars in computer vision research and product development.
With this in mind, we decided to find out how the top global tech firms are making use of computer vision and explore what kind of new technology and media could appear over the next few years. To learn more about how Emerj maps the capability-space of AI (including computer vision) across sectors, visit our AI Opportunity Landscape page.
From our research, we’ve found that many of the use cases of computer vision fall into the following clusters:
- Retail and Retail Security
- Automotive
- Healthcare
- Agriculture
- Banking
- Industrial
In providing you with this industry application report, we aim to give business leaders a bird’s eye view of the available applications in the market, and help them determine if AI is the right solution for their business.
Retail and Retail Security
Amazon
Amazon recently opened to the public the Amazon Go store where shoppers need not wait in line at the checkout counter to pay for their purchases. Located in Seattle, Washington, the Go store is fitted with cameras specialized in computer vision. It initially only allowed Amazon employee shoppers, but welcomed the public beginning in early 2018.
The technology that runs behind the Go store is called Just Walk Out. As shown in this one-minute video, shoppers activate the IOS or Android mobile phone app before entering the gates of the store.
As also seen in the video, cameras are placed in the ceiling above the aisles and on shelves, Using computer vision technology, the company website claims that these cameras have the capability to determine when an object is taken from a shelf and who has taken it. If an item is returned to the shelf, the system is also able to remove that item from a customer’s virtual basket. The network of cameras allows the app to track people in the store at all times, ensuring it bills the right items to the right shopper when they walk out, without having to use facial recognition.
As the name suggests, shoppers are free to walk out of the store once they have their products. The app will then send them an online receipt and charge the cost of the products to their Amazon account.
While the store has eliminated cashiers, The New York Times reports that store employees are still available to check IDs in the store’s alcohol section, restock shelves and assist customers with finding products or aisles. An Amazon rep also confirmed to Recode that human employees work behind screens at the Go store to help train the algorithms and correct them if they wrongly detect that items are pulled off the shelf.
Amazon has not revealed plans for the Go store in the longer term, but the company has registered Go trademarks in the UK.
While Amazon in 2017 purchased Whole Foods, Gianna Puerini, Vice President at Amazon Go, said the company has no plans to implement the Just Walk Out Technology in the supermarket chain.
In retail fashion, Amazon has applied for a patent for a virtual mirror. In the patent, the company said,“For entertainment purposes, unique visual displays can enhance the experiences of users.”
The virtual mirror technology, sketched in the patent image below, is described as a blended-reality display that puts a shopper’s images into an augmented scene, and puts the individual in a virtual dress.
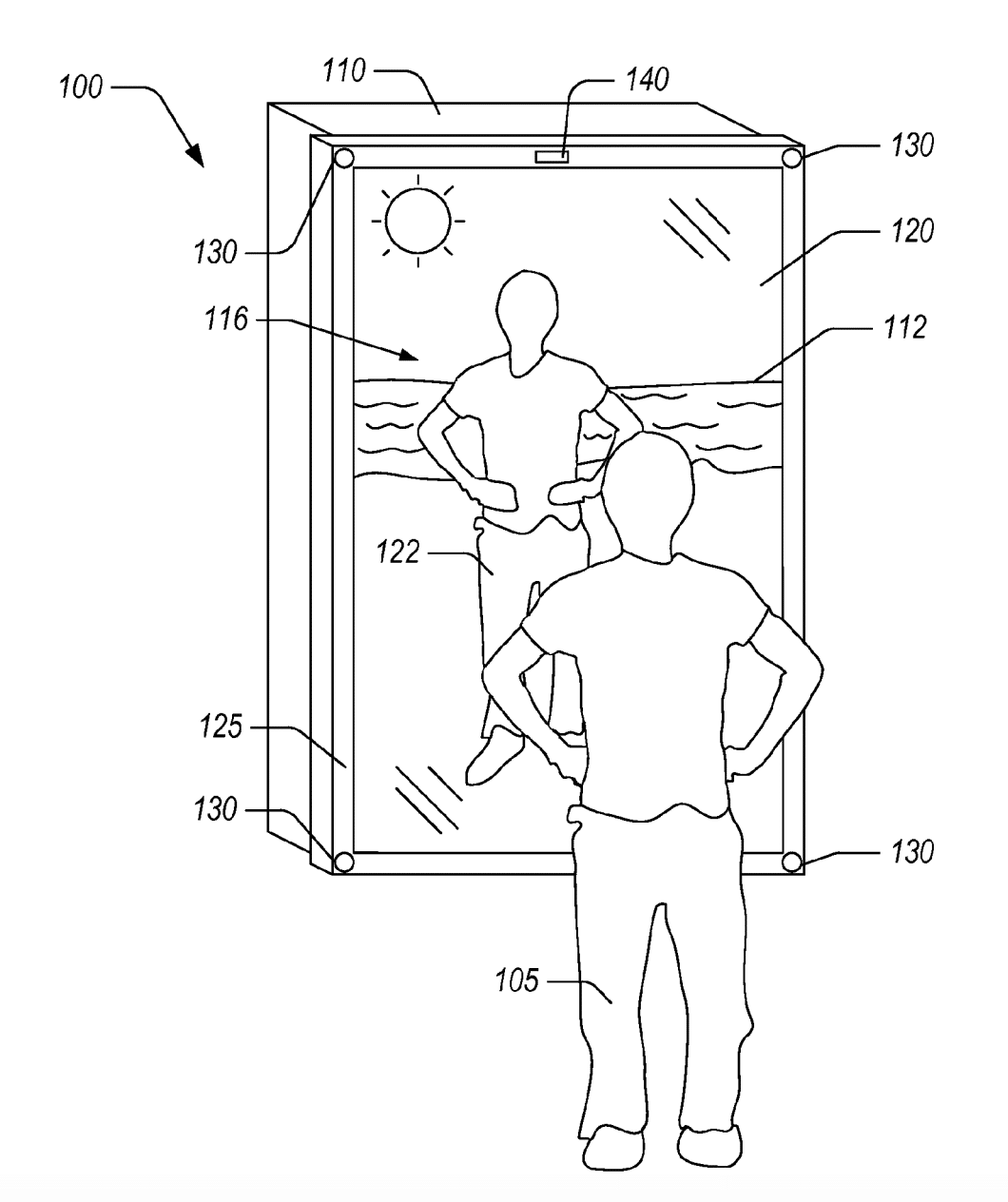
According to the patent, the virtual mirror will use enhanced facial detection, a subset of computer vision, whose algorithms will locate the eyes. Catching the user’s eye position will let the system know what objects the user is seeing in the mirror. The algorithms will then use this data to control the projectors.
Amazon has not made any announcement on this development and the virtual mirror has not been deployed, but the sketches released by the patent office show how a user could see illuminated objects reflected on the mirror combined with images transmitted from the display device to create a scene.
For example, based on the patent descriptions, the transmitted image could show a scene, say a mountain trail, as software would put the shopper into the scene and potentially superimpose virtual clothes onto the reflection of his body. Face-tracking sensors and software would show a realistic image from all angles. The shopper would be able to try on several outfits without needing to put them on.
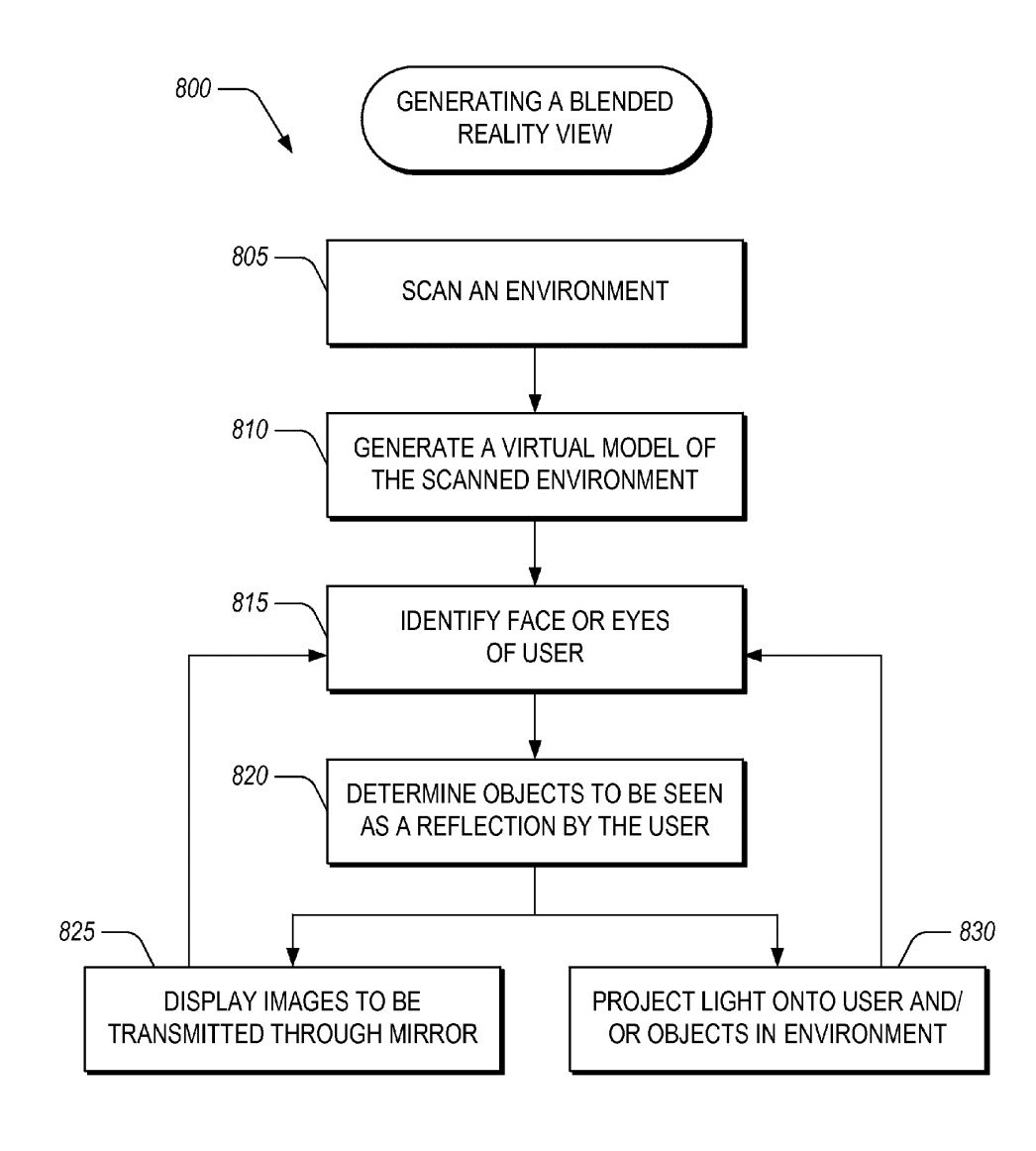
There is no available demo of Amazon’s virtual mirror but here’s a 2-minute video sample of how Kinect’s on-the-market Windows virtual mirror works, showing a shopper “trying on” outfits, superimposing it on the image of her body in the mirror, following her movements, and even changing the color of items at her voice command:
Amazon has previously released Echo Look, a voice-activated camera that takes pictures and six-second videos of an individual’s wardrobe and recommends combinations of outfits.
This 2-minute review of the app shows how it claims to use Amazon’s virtual assistant Alexa to help users compile images of clothes and can even recommend which outfit looks better on the individual.
As the video shows, a user can speak to the gadget and instruct it to take full-body photos or a six-second video. The content is collated to create an inventory of the user’s wardrobe, according to Amazon. Alexa compares two photos of the user in different outfits and recommends which looks better.
According to Amazon, Echo Look is equipped with a depth-sensing camera and computer vision-based background blur that focuses on the image of the user. Part of the company’s home automation product line, it is intended for consumers and priced at $200. It is not clear if any retail businesses have used it.
StopLift
In retail security specific to groceries, Massachusetts-based StopLift claims to have developed a computer-vision system that could reduce theft and other losses at store chains. The company’s product, called ScanItAll, is a system that detects checkout errors or cashiers who avoid scanning, also called “sweethearting.” Sweethearting is the cashier’s act of fake scanning a product at the checkout in collusion with a customer who could be a friend, family or fellow employee.
ScanItAll’s computer vision technology works with the grocery store’s existing ceiling-installed video cameras and point-of-sale (POS) systems. Through the camera, the software “watches” the cashier scan all products at the checkout counter. Any product that is not scanned at the POS is labeled as a “loss” by the software. After being notified of the loss, the company says it is up to management to take the next step to accost the staff and take measures to prevent similar incidents from happening in the future.
Using algorithms, Stoplift claims that ScanItAll can identify sweethearting behaviors such as covering the barcode, stacking items on top of one another, skipping the scanner and directly bagging the merchandise.
The three-minute video below shows how the ScanItAll detects the many ways items are skipped at checkout, such as pass around, random weight abuse, cover-up, among others, and how grocery owners can potentially stop the behavior.
In a StopLift case study of Piggly Wiggly, StopLift claims that incidences of sweethearting at two of its grocery outlets have diminished since the computer vision system was deployed. From losses amounting to a total of $10,000 monthly due to suspected failure to scan items at checkout, losses have dropped to $1,000 monthly. These losses are mostly identified as errors, rather than suspicious behavior, according to StopLift. Piggly Wiggly representatives said that cashiers and staff were notified that the system had been implemented, but it is unclear of whether that impacted the end result.
Based on news stories, the company claims to have the technology installed in some supermarkets in Rhode Island, Massachusetts, and Australia, although no case studies have been formally released.
CEO Malay Kundu holds a Master of Science in Electrical Engineering and Computer Science from the Massachusetts Institute of Technology. Prior to this, he led the development of real-time facial recognition systems that identify terrorists in airports for Facia Reco Associates (licensor to facial recognition company Viisage), and delivered the first such system ever to the Army Research Laboratory.
Automotive
According to the World Health Organization, more than 1.25 million people die each year as a result of traffic incidents. The WHO adds that this trend is predicted to become the seventh leading cause of death by 2030 if no sustained action is taken. Nearly half of road casualties are “vulnerable road users:” pedestrians, cyclists and motorcyclists. According to this research, there’s a clear theme to the vast majority of these incidents: human error and inattention.
Waymo
One company that claims to make driving safer is Waymo. Formerly known as the Google self-driving car project, Waymo is working to improve transportation for people, building on self-driving car and sensor technology developed in Google Labs.
The company website reports that Waymo cars are equipped with sensors and software that can detect 360 degrees of movements of pedestrians, cyclists, vehicles, road work and other objects from up to three football fields away. The company also reports that the software has been tested on 7 million miles of public roads to train its vehicles to navigate safely through daily traffic.
The 3-minute video below shows how the Waymo car navigates through the streets autonomously.
According to the video, it is able to follow traffic flow and regulations, and detects obstacles in its way. For example, when a cyclist extends his left hand, the software will detect the hand signal and predict if that cyclist will move to another lane. The software can also instruct the vehicle to slow down to let the cyclist to pass safely.
The company claims to use deep networks for prediction, planning, mapping and simulation to train the vehicles to maneuver through different situations such as construction sites, give way to emergency vehicles, make room to cars that are parking, and stop for crossing pedestrians.
“Raindrops and snowflakes can create a lot of noise in sensor data for a self-driving car. Machine learning helps us filter out that noise and correctly identify pedestrians, vehicles and more,” wrote Dmitri Dolgov, Waymo’s Chief Technology Officer and VP of Engineering in a blog. He holds a Master of Science degree in Physics and Math from the Moscow Institute of Physics and Technology and a Doctorate in Computer Science from the University of Michigan.
There were reports that a Waymo van was involved in an accident in Arizona while on autopilot with the driver behind the wheel, but police said the software was not believed to be at fault.
On its website, the company said it intends to apply the technology to a variety of transportation types from ride-hailing and logistics, to public transport and personal vehicles. According to Waymo press releases, the company has partnered with Chrysler and Jaguar on self-driving car technology.
Tesla
Another company that claims it has developed self-driving cars is Tesla, which claims that all its three Autopilot car models are equipped for full self-driving capability.
Each vehicle, the website reports, is fitted with eight cameras for 360-degree visibility around the car with a viewing distance of 250 meters around. Twelve ultrasonic sensors enable the car to detect both hard and soft objects. The company claims that a forward-facing radar enables the car to see through heavy rain, fog, dust and even the car ahead.
Its camera system, called Tesla Vision, works with vision processing tools which the company claims are built on a deep neural network and able to deconstruct the environment to enable the car to navigate complex roads.
This 3-minute video shows shows a driver with his hands off the wheel and feet off the pedals as they move through rush hour traffic in a Tesla Autopilot car.
Last March 2018, a Tesla self-driving car was involved in a fatal accident while autopilot was engaged. The report said that the driver did not have his hands on the wheel despite the platforms warnings, telling him to do so. In the six seconds that hands were not on the wheel, his SUV hit a concrete divider, killing the driver. It was later discovered that the driver nor the car activated the brakes before the crash.
Recent software improvements introduced to Tesla cars include more warnings to drivers to keep their hands on the steering wheel. After three warnings, the software prevents the car from running until the driver restarts, according to the company.
Healthcare
In healthcare, computer vision technology is helping healthcare professionals to accurately classify conditions or illnesses that may potentially save patients’ lives by reducing or eliminating inaccurate diagnoses and incorrect treatment.
Gauss Surgical
Gauss Surgical has developed blood monitoring solutions that are described to estimate in real-time blood loss during medical situations. This solution, the website reports, maximizes transfusions and recognizes hemorrhage better than the human eye.
Gauss Surgical’s Triton line of blood monitoring solutions includes Triton OR which uses an iPad-based app to capture images of blood on surgical sponges and suction canisters. These images are processed by cloud-based computer vision and machine learning algorithms to estimate blood loss. The company says the application is currently used by medical professionals in hospital operating rooms during surgical operations or Caesarian deliveries.
This 6-minute video shows Triton how captures images of sponges or cloths that absorb blood during a medical procedure, works as a real-time scanner and estimates the potential of blood loss in the patient. CEO of Gauss Surgical Siddharth Satish explains how the solution uses computer vision technology to predict the onset of hemorrhage.
The Triton OR app underwent clinical studies in childbirth settings to validate accuracy and precision, and was cleared by the US Food and Drug Administration in 2017.
Gauss Surgical in 2017 conducted an Accuracy of Blood Loss Measurement during Cesarean Delivery study at the Santa Clara Valley Medical Center. By the computer vision (colorimetric image analysis), the application determined amounts of blood on surgical sponges and in suction canisters collected from 50 patients during cesarean deliveries.
When the application’s estimates were compared with obstetricians’ visual estimates, it was discovered that the application’s estimates were more accurate, presenting the hospital with an opportunity to potentially improve clinical outcomes.
Kevin Miller is the Senior Algorithm Scientist. He holds an M.S. in Computer Science from Stanford University.
DeepLens and DermLens
Amazon Web Services (AWS) also developed DeepLens, a programmable deep learning-enabled camera that can be integrated with open source software in any industry. In this video, DeepLens is described as a kit that programmers from various industries can use to develop their own computer-vision application.
One healthcare application that uses the DeepLens camera is DermLens, which was developed by an independent startup. DermLens aims to assist patients to monitor and manage a skin condition called psoriasis. Created by digital health startup Predictably Well’s Terje Norderhaug, who holds a Master’s degree in Systems Design from the University of Oslo, the DermLens app is intended as a continuous care service where the reported data is available for the physician and care team.
The 4-minute video instructs developers on how to create and deploy an object-detection project using the DeepLens kit.
According to the video, developers will need to enter the Amazon Web Services (AWS) management console with their own username and password.
From within the console, developers must choose a pre-populated object-detection project template, then enter the project description and other values in appropriate fields. The console has a method in which the project will deploy to the developer’s target device, and enable developers to view the output from their own screen.
For DermLens, this short video explains how the application’s algorithms were trained to recognize psoriasis, by feeding it with 45 images of skin that showed typically red and scaly segments. Each image in the set comes with a mask indicating the abnormal skin. The computer vision device then sends data to the app, which in turn presents the user with an estimate of the severity of the psoriasis.
The DermLens team also created a mobile app for self-reporting of additional symptoms such as itching and fatigue..
In a case study published in the Journal of American Dermatology, DermLens claims to have been tested on 92 patients, 72 percent of whom said they preferred using the DermLens camera compared with using a smartphone. The study also revealed that 98 percent of patients surveyed said they would use the device to send images to the health care provider if the device was available.
At the moment, a search of the company website and the web does not reveal any clients.
Agriculture
Some farms are beginning to adopt computer vision technology to improve their operations. Our research suggests that these technologies aim to help farmers adopt more efficient growth methods, increase yields, and eventually increase profit. We’ve covered agricultural AI applications in great depth for readers with a more general interest in that area.
Slantrange
Slantrange claims to offer computer vision-equipped drones that are connected to what the company calls an “intelligence system” consisting of sensors, processors, storage devices, networks, an artificial intelligence analytics software and other user interfaces to measure and monitor the condition of crops. At 120 meters above ground level, the camera has a resolution of 4.8 cm/pixel. However, its website notes that flying lower provides better resolution.
The company claims that the drone captures images of the fields to show the different signatures of healthy crops compared with “stressed” crops. These stressors include pest infestations, nutrient deficiencies and dehydration; and metrics to estimate potential yield at harvest, and others. These signatures are passed on to the SlantView analytics system which interprets the data and ultimately helps farmers make decisions related to treatment for stress conditions.
This 5-minute video provides a tutorial on how to use the basic functions of the SlantView app, starting with how a user can use the solution to identify stressed areas through drone-captured images.
According to case study, Alex Petersen, owner of On Target Imaging, believed that changing their farming approach from analog to digital agriculture could bring more crop with less inputs and translating to more efficient farming.
Using the Slantrange 3p sensor drone, the team flew and mapped the first 250 acres of corn fields to determine areas of crop stress from above. This enabled them to collect data about areas with high nitrate levels in the soil, which would negatively affect the sugar beet crops.
The team then processed the data in SlantView Analytics, whose algorithms have been trained to determine if a plant is a crop or weed for accurate counts. Slantange claims that its drones only requires 17 minutes of flight time to cover 40 acres of field, equivalent to about 8 minutes of data processing. Alex reports that it took about 3 hours of combined flying and data processing to produce stress maps of the area covered.
The resulting data enabled the team to decide using 15% to 20% less nitrogen fertilizer on 500 acres, which translated to savings of about 30-40 pounds per acre or $9 to $13 per acre. They also anticipated healthier corn and estimate an increase in crop yield of about 10 bushels per acre. The combined savings, higher yields and increased profits have allowed the team to recoup the investment of implementing precision farming, according to the blog.
Michael Ritter, CEO at Slantrange, has a BS in Mechanical Engineering from University of California, San Diego and and MBA from Georgetown University. Since 1996, Michael has been developing airborne intelligence systems for applications in the earth sciences, defense, and agronomy fields. Prior to Slantrange, he was the Director of Electro-Optic Systems and Manager of Mission Systems at General Atomics Aeronautical Systems, where he oversaw the development of intelligence payloads for a number of unmanned military aircraft.
Cainthus
Animal facial recognition is one feature that Dublin-based Cainthus claims to offer. Cainthus uses predictive imaging analysis to monitor the health and well-being of crops and livestock. Cainthus uses predictive imaging analysis to monitor the health and well-being of crops and livestock.
The two-minute video below shows how the software could use imaging technology to identify individual cows in seconds, based on hide patterns and facial recognition, and tracks key data such as food and water intake, heat detection and behaviour patterns. These pieces of information are taken by the AI-powered algorithms and sends health alerts to farmers who make decisions about milk production, reproduction management and overall animal health.
Cainthus also claims to provide features like all-weather crop analysis in rates of growth, general plant health, stressor identification, fruit ripeness and crop maturity, among others.
Cargill, a producer and distributor of agricultural products such as sugar, refined oil, cotton, chocolate and salt, recently partnered with Cainthus to bring facial recognition technology to dairy farms worldwide. The deal includes a minority equity investment from Cargill although terms were not disclosed.
According to news reports in February 2018, Cargill and Cainthus are working on trials using pigs, and aim to release the application commercially by the end of the year. There are also plans to expand the application to poultry and aquaculture.
Victor Gehman, Director of Science at Cainthus Corporation and a Doctor of Physics from the University of Washington, is responsible for image processing, data analysis, computer modeling, machine learning/feature detection, computer vision, optics, mechanical prototyping, and phenomenological analysis. His experience at Insight Data Science as a fellow for more than four years allowed him to build skills and a professional network to transition from academe to the data science industry.
Banking
While most of our previous coverage of AI in banking has involved fraud detection and natural language processing, some computer vision technology has also found its way into the banking industry as well.
Mitek Systems
Mitek Systems offers image recognition applications that use machine learning to classify, extract data, and authenticate documents such as passports, ID cards, driver’s licenses, and checks.
The applications work by having customers take a photo of an ID or a paper check using their mobile device and send to the user’s bank where computer vision software on the bank’s side verifies authenticity. Once verified and accepted by the user’s bank, the application or check is processed. For deposits, funds typically become available to the customer within a business day, according to the Mitek company website.
The two-minute demo below shows how the Mitek software works on mobile phones to capture the image of a check to be deposited to an account:
To start the process, the user enters his mobile phone number into the bank’s application form. A text message will be sent to his phone with a link the user can click to open an image-capture experience. The customer can choose from a driver license, ID card or passport. Mitek’s technology recognizes thousands of ID documents from around the world. Front and back images of the ID or document are required.
Once the user has submitted the images, the application will real-time feedback to ensure that high-quality images are captured. The company claims that its algorithms correct images; dewarp, deskew, distortion, and poor lighting conditions.
Mobile Verify detected that this user started his session at his desktop, so once it’s done processing, it will automatically deliver the data extracted to the user’s channel of choice, desktop in this case.
A client, Mercantile Bank of Michigan, wanted to expand its retail bank portfolio and associated core deposits by providing retail customers with digital services. The bank chose Mitek’s application called Mobile Deposit that allows customers to deposit checks using their camera smartphones.
Mitek claims that it took just over 30 days to implement the application. Within four months, 20 percent of the bank’s online banking users started using mobile banking, according to the study.
In the same period, the monthly enrollment into the bank’s Consumer Deposit Capture program increased by 400 percent, due to the shift in mobile banking usage from the flat-bed scanner solution.
Stephen Ritter, Chief Technology Officer, is responsible for Mitek Labs and Engineering. Prior to Mitek, Ritter lead technology for Emotient, a facial analytics startup acquired by Apple. He also served in technology leadership roles in Websense (now Forcepoint) and McAfee. He holds a B.S. in Cognitive Science, Computer Science, Economics from the University of California, San Diego.
Industrial
Osprey Informatics
In the industrial sector, computer vision applications such as Osprey Informatics are being used to monitor the status of critical infrastructure, such as remote wells, industrial facilities, work activity and site security. In its website, the company lists Shell and Chevron as among its clients.
In a case study, a client claims that Osprey’s online visual monitoring system for remote oil wells helped it reduce site visits and the equivalent cost. The client was seeking ways to make oil production more efficient in the face of depressed commodity prices. The study notes that it turned to Osprey to deploy virtual monitoring systems at several facilities for operations monitoring and security, and to identify new applications to improve productivity.
The Osprey Reach computer vision system was deployed to the client’s high-priority well sites to provide 15-minute time-lapse images of specific areas of the well, with an option for on-demand images and a live video. Osprey also deployed at a remote tank battery, enabling operators to read tank levels and view the containment area.
According to the case study, the client was able to reduce routine site visits by 50 percent since deploying the Osprey solution. The average cost for an in-person well site inspection was also reduced from $20 to $1.
The 3-minute video below shows how the Osprey Reach solution claims to allow operators to remotely monitor oil wells, zooming in and out of images to ensure there are no leaks in the surrounding area. The on-demand video also shows the pump jack operating at a normal cadence.
According to the company website, the list of industrial facilities that could potentially use computer vision include oil and gas platforms, chemical factories, petroleum refineries, and even nuclear power plants. Information gathered by sensors and cameras are passed on to AI software which alerts the maintenance department to take safety measures at even the slightest stress detected by the application.
Concluding Thoughts
Computer vision applications have emerged in more industries, although some have adopted the technology faster than others. Whatever computer vision technology exists continues to rely on the human element, to monitor, analyze, interpret, control, decide and take action.
In the automotive industry, global companies such as Google and Tesla are moving forward in improving self-driving cars equipped with computer vision cameras. However, with reported fatal accidents, it is clear that these cars are not completely ready to be commercially available and cannot be entirely autonomous.
In retail stores such as the Amazon Go store, human employees continue to work in customer service, and behind the screens to train the algorithms and confirm that the machine learning capability is on track. In terms of retail security, the technology assists to capture videos of the theft incidents, but human resources must step in to correct erring employees.
The application of computer vision in agriculture has been slow to take off. But companies such as Cainthus have entered this market aiming to take the technology from other industries and apply it to agriculture.
These applications claim to offer farmers the opportunity to conduct precision farming, to raise production at lower cost. The partnership of Cainthus and Cargill could potentially open other forms of artificial intelligence to the rest of the industry.