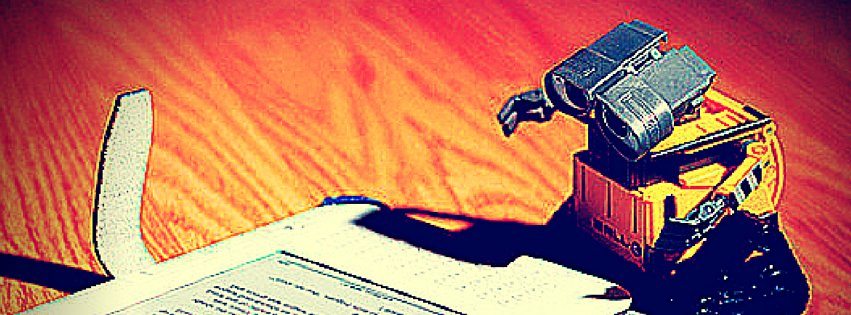
Of all the body’s organs, we understand the brain the least. And yet, it’s this organ whose secrets best hepalp the development of artificial intelligences. One of these secrets includes the labyrinth of biological neural networks – connections within the brain that enable information to be processed, shared, and recorded. In recent years, scientific comprehension of neural networks has enabled engineers to create artificial systems that function in ways similar to (though not exactly like) human brains. In a process called deep learning, artificial neural networks can analyze information in huge stores of data in order to recognize patterns in language and facial features.
And though artificial neural networks are modeled from the ones within our brain, the two are not a lot alike. Where our neural networks have thousands of synapses that branch out from every nerve, artificial neural networks have just a few. Why? Because no one is really sure why our brains need so many connections. Artificial neural networks seem to function just fine without thousands of nodes branching off from each other. Indeed the inclusion of so many nodes may just complicate the processes. Thus, though their essential function may be similar they are not equal – our brain compared to an artificial neural network is like a sports car competing with a bicycle.
This approach is set to change though thanks to two entrepreneurs whose Silicone Valley startup, Numenta, wants to unlock and exploit the vast nuances of biological information processing.
In short, Jeff Hawkins and Subutai Ahmad have developed a new theory (published on arXic) aim will revolutionize our approach to neural networks – a theory that, once applied, could shepherd AI into a new era. But before we get into their theory, let’s take a quick look at neurons.
Our brains’ neurons consist of a cell body (soma), a number of close extensions (proximal dendrites), and a number of distant extensions (distal dendrites) connected by a long cable.
These dendrites serve thousands of connections called synapses, which when combined help translate signals into thoughts. And though proximal synapses are understood to help neurons “learn” certain patterns, the role of distal synapses are less well understood.
Hawkins and Ahmad suggest that – like their proximal neighbors – distal synapses help neurons recognize patterns but, more importantly, help the neuron prepare for and predict upcoming signals. Where proximal dendrites fire when a pattern is recognized, distal dendrites predict what patterns may be next depending on previous experience. Thus, Hawkins and Ahmad suggest, neurons both recognize patterns and predict sequences of patterns with the help of distal dendrites. The great quantity of synapses is required in order for the neuron to perform both functions – pattern recognition and prediction – so quickly and so seamlessly.
In another leap of insight, Hawkins and Ahmad claim that what is most important in predicting patterns is not the total amount of signal and noise received, but the slight differences between signal and noise behind patterns. Using this model, the two entrepreneurs were able to teach an artificial neuron to recognize hundred of patterns.
If their theory is deemed accurate, Hawkins and Ahmad may help roboticists and AI engineers develop a more refined system for recognizing patterns. Where established theories of neural networks prioritized pattern recognition, the key could be to consider pattern prediction in order to help systems act and react more smoothly.
Hawkins invented the Palm Pilot in the 1990s so he’s no newbie to technology. But since then he’s been more focused on neuroscience and entrepreneurship, co-founding Numenta with Ahmad and a mchine to “lead a new era of machine intelligence”. With their new research and apparently enlightened understanding of how neurons function, it may not be long until artificial neural networks are indistinguishable from our own.
Credits: Shutterstock, Numenta