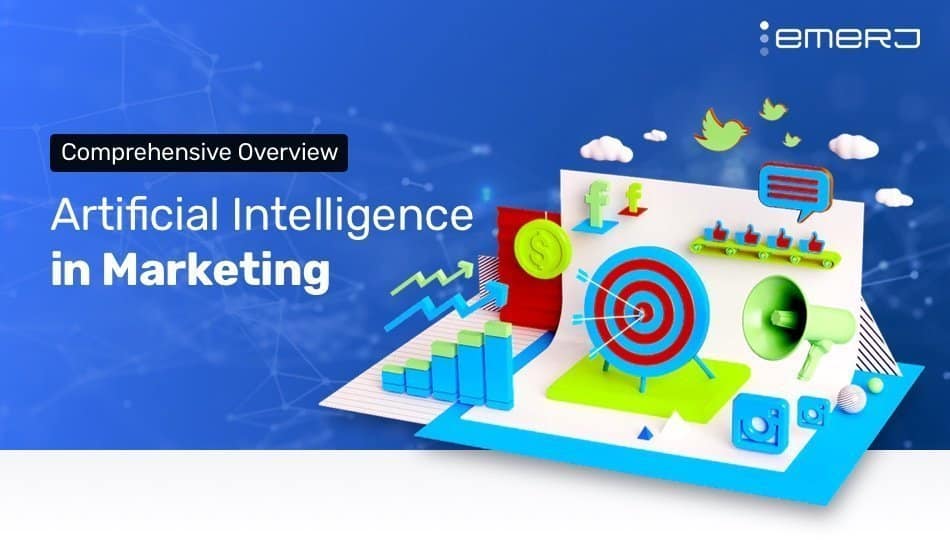
In the hundreds of researcher and executive interviews we’ve been fortunate enough to conduct in the last three years, few artificial intelligence applications are brought up more than marketing and advertising. During talks with execs and researchers from companies ranging from Facebook to Baidu, and IBM to AT&T, marketing has been a perennial theme in conversations of AI’s hottest applications.
This article aims to serve as a kind of executive primer for the intersection of AI and marketing, and the article itself has been broken into three distinct sections for that purpose:
- Real-world examples artificial intelligence marketing / advertising applications with significant traction today
- Burgeoning future applications for AI in marketing / advertising
- A brief glossary of basic marketing-related AI terms, with links to credible references
(Note: Readers of this article might like our “machine learning in marketing” market research, featuring the opinions and predictions of over 50 AI in marketing executives, including estimations of 5-year trends, and industries most likely to be impacted by the development of AI for marketing applications.)
We’ll begin with examples of what’s currently viable in the AI marketing world:
5 Current Artificial Intelligence Applications in Marketing and Advertising
Below are seven extremely prevalent example applications that we’ve decided to highlight for this article, accompanied by a brief description of how the AI approach works, and companies currently leveraging the application.
In this section of the article I’ve aimed to stay away from (a) applications with limited traction (speculative or burgeoning applications are reserved for the next section), and (b) AI applications that have only partial overlap with marketing today (IE: fraud and security could / should be considered to be their own category, and will not be referred to here as a marketing application).
A complete list of currently viable AI marketing applications would be much more broad, but we’ve decided to focus on some of the most popular uses in marketing today:
1 – Search
In 2005, if you “searched” an eCommerce store to find a product, you’d be unlikely to find the result you had in mind unless you knew it’s name or title exactly. Today’s “search” is after much smarter, and it’s improved capacity not only help you find information on Google, but it helps you find the right products on Amazon or Target.com, the right movies on Netflix, and more.
Ten years ago, typing “men’s flip flops” at Nike.com may not have yielded the results I was looking for. Today, it very much does.
Search improvement for eCommerce and marketing has improved due to the same underlying factors that have improved “search” at large, including:
- Technologies like Elasticsearch are now relatively mainstream, allowing any small eCommerce stores to have search that goes beyond simply matching keywords
- Data-as-a-Service companies (like Indix, among others) make it easier than ever to draw from search data from other larger sources, informing your own online product search without having to train your own search models from scratch
- Other misc improvements, such as: Software to detect common misspellings is now more commonplace, and can calibrate for misspellings by context (IE: “Season cikets” can be understood to mean “season tickets”, while “cikets” alone might be more difficult to discern without context)
Google has done good work of simplifying and explaining some of their own search improvements and developments in their “Inside Search” writings.
Our recent interview with Samuir Raman (Data Science at Mattermark) explains some of the dynamics involved in improving search (full episode here).
In the future, consumers can expect more and more eCommerce sites to follow in the footsteps of Google and others in implementing autosuggest, suggested corrections, “advanced” search options, and other such improvements.
[tecarousel]
2 – Recommendation Engines
Recommendation engines are a rarity in the world of digital marketing in that this marketing technology is often appreciated and even loved by customers. Amazon’s book or product recommendations are excellent, Spotify knows your taste in music so well, this kind of “discovery aide” amongst the millions of choices available makes them indispensable for companies with huge inventories (both digital and physical).
Today’s sophisticated online recommendations go vastly beyond a simple human-determined set of guidelines, or even a simple set of historical ratings from other users. A recommendation engine (you’ll also frequently hear the term “recommender system”) can pull from reams of nuanced data in order to draw conclusions from behaviors, actions, etc…
Netflix isn’t merely taking into account what movies a person has watched, or what ratings they give those movies – they’re also analyzing which movies are watched multiple times, rewound, fast-forwarded, etc… These myriad behaviors, when correlated and assessed over millions of other users, help to coax out the best recommendations.
There have been some lay-person-friendly video tutorials on the basics of recommendation systems, including a short recommender video series by Andrew Ng, which are worth the watch. Harvard has a useful 13-minute intro video as well.
3 – Programmatic Advertising
In italics below is a brief explanation of programmatic advertising by Seer Interactive, whose done a great job of explaining the basics:
Simply stated, programmatic advertising is the automated process of buying and selling ad inventory through an exchange, connecting advertisers to publishers. This process uses artificial intelligence technologies… and real-time bidding for inventory across mobile, display, video and social channels – even making its way into television.
Artificial intelligence technologies have algorithms that analyze a visitor’s behavior allowing for real time campaign optimizations towards an audience more likely to convert. Programmatic companies have the ability to gather this audience data to then target more precisely, whether it’s from 1st party (their own) or from a 3rd party data provider.
Programmatic media buying includes the use of DSPs, SSPs and DMPs. DSPs, (demand side platforms) which facilitate the process of buying ad inventory on the open market, provide the ability to reach your target audience due to the integration of DMPs (data management platforms). DMPs collect and analyze a substantial amount of cookie data to then allow the marketer to make more informed decisions of whom their target audience may be.
The classic example of programmatic advertising is SEM advertising on channels like Google (AdWords), Facebook, and Twitter. Companies like PredictiveBid and Israel-based Albert have decided to put a significant amount of their focus on programmatic advertising specifically.
Programmatic ads bring a tremendous amount of efficiency to bear on the “inventory” of website and app viewers. Platforms like Google and Facebook have set the standard for both efficient and effective advertising – and it can be supposed that these systems will become more and more user-friendly in terms of allowing non-technical marketers to start, run, and measure campaigns on line (for all me
4 – Marketing Forecasting
This section might be referred to as “Insight from Marketing Data,” a much broader topic. However, one of the most straight-forward marketing applications of business intelligence data lies in it’s ability to aide in predictions, a capability much enhanced by developments in AI.
We’ll likely compose another entire article (or indeed, an entire market report) on the domain of business intelligence. However, BI and marketing intersect so thoroughly that it would be disingenuous to refer to the rapid-fire advancements in marketing without referencing the underlying technology that helps companies make sense of that flood of marketing data and take action on it.
Companies like Rapidminer, Birst, Sisense, and others are aiming to become industry standards for business intelligence and predictions. Because of the (generally) high volume and quantifiable nature of marketing data (clicks, views, time-on-page, purchases, email responses, etc…), models can often be trained much more quickly on marketing data than on other information such as HR data, inventory data, etc…
Being able to predict the success of an email campaign or marketing initiative can help companies continuously improve marketing efforts (in display, text, video, or even direct mail).
Of the 2000+ marketing technology companies on the market at the time of this writing, a wide swath deal with data management and analysis. We can expect more of these companies in the future, and more refined marketing efforts from companies large and small as these technologies / assessment tools improve and become more mainstream.
5 – Speech / Text Recognition (Conversational Commerce)
In 2014 and even in most of 2015, it might have been safe to say that while chatbots and speech recognition were an interesting use for artificial intelligence, it still hadn’t made a legitimate impact in marketing or advertising.
Beginning in 2016, a wave of legitimately viable speech and chat interfaces have crossed over into the marketing worlds – and some of them showed grand promise. Here are a few of the examples of traction today:
- Amazon Echo – Echo has been a remarkable success in turning the internet of things into a reality… particularly in it’s ability for users to make purchases simply by speaking to the machine. You can order an Uber car or a Domino’s pizza with speech alone.
- Facebook Messenger – Aiming to model the “online to offline” strategy of chat-based purchases, Facebook Messenger allows users order flowers (and soon, much more) via chat alone.
- Baidu’s Duer – Baidu’s new chatbot assistant is capable of ordering products within it’s interface (PC World obtained some of the earliest coverage of the bot here)
- Others – Google and (reportedly) Apple are working on Echo competitors, and the competition over which brand will become the speech “hub” of the smart home should prove to be an exciting one.
While chatbots and natural language processing haven’t made their way into the marketing departments of most of America’s small businesses, the applications from the largest and hottest tech companies are certainly making waves, and making it clear that there’s a bigger trend ahead.
AI in Marketing / Advertising – Burgeoning Opportunities Ahead
While there are hundreds of potential applications of AI in marketing and advertising, the short list below highlights some of the possibilities that we consider to be exciting, and viable in the coming half decade:
Image recognition / machine vision: In the relatively near future, it may be possible for consumers on the web or on mobile to “search” for products (or similar products) to images. This might be as simple as snapping a photo of a pair of shoes you want to buy, or using a web app to select a certain image that you found within a Google search. Companies like CamFind and their competitors and experimenting in this space already.
Our podcast has features some amazing researchers and execs in the domain of machine vision. Our interview with Shutterstock’s Nathan Hurst might be of particular interest to readers with a curiosity for the future of image recognition.
Customer segmentation: Companies such as AgilOne are allowing marketers to optimize email and website communications, continually learning from user behavior (eConsultancy).
Content generation: You might already be aware that a significant portion of sports and finance-related articles are written by machines, not by humans. Yeah – expect more of that.
Companies like Automated Insights and Narrative Science have found ways to turn specific sets of information (domains like sports and finance are laden with time data and numbered data) into human-readable articles, sometimes indistinguishable from those written by human beings. In the future, companies may have product descriptions and entire product line-related articles composed entirely by machines, based on information about the products in question.
Personalization of content will also be an important future trend (Adobe and other companies are already competing on this feature as well).
Glossary of Marketing-Related Artificial Intelligence Terms
In your further exploration of this domain, you’re likely to come in contact with a variety of technical terms and industry jargon. Below are a set of terms that we believe to be important for executives and product managers to grasp:
Programatic advertising: Simply stated, programmatic advertising is the automated process of buying and selling ad inventory through an exchange, connecting advertisers to publishers. This process uses artificial intelligence technologies… and real-time bidding for inventory across mobile, display, video and social channels – even making its way into television. (Seer Interactive)
Supply-side platform (SSP): A piece of software used to sell advertising in an automated fashion. SSPs are most often used by online publishers to help them sell display, video and mobile ads. (DigiDay)
Demand-side platform (DSP): A system that allows buyers of digital advertising inventory to manage multiple ad exchange and data exchange accounts through one interface. (Wikipedia)
Recommendation engine: A recommendation engine is a feature (not a product) that filters items by predicting how a user might rate them. It solves the problem of connecting your existing users with the right items in your massive inventory (i.e. tens of thousands to millions) of products or content. (Data Community DC)
Real-time bidding (RTB): Refers to the buying and selling of online ad impressions through real-time auctions that occur in the time it takes a webpage to load. Those auctions are often facilitated by ad exchanges or supply-side platforms. (DigiDay)
Header image credit: www.cloudpix.co/