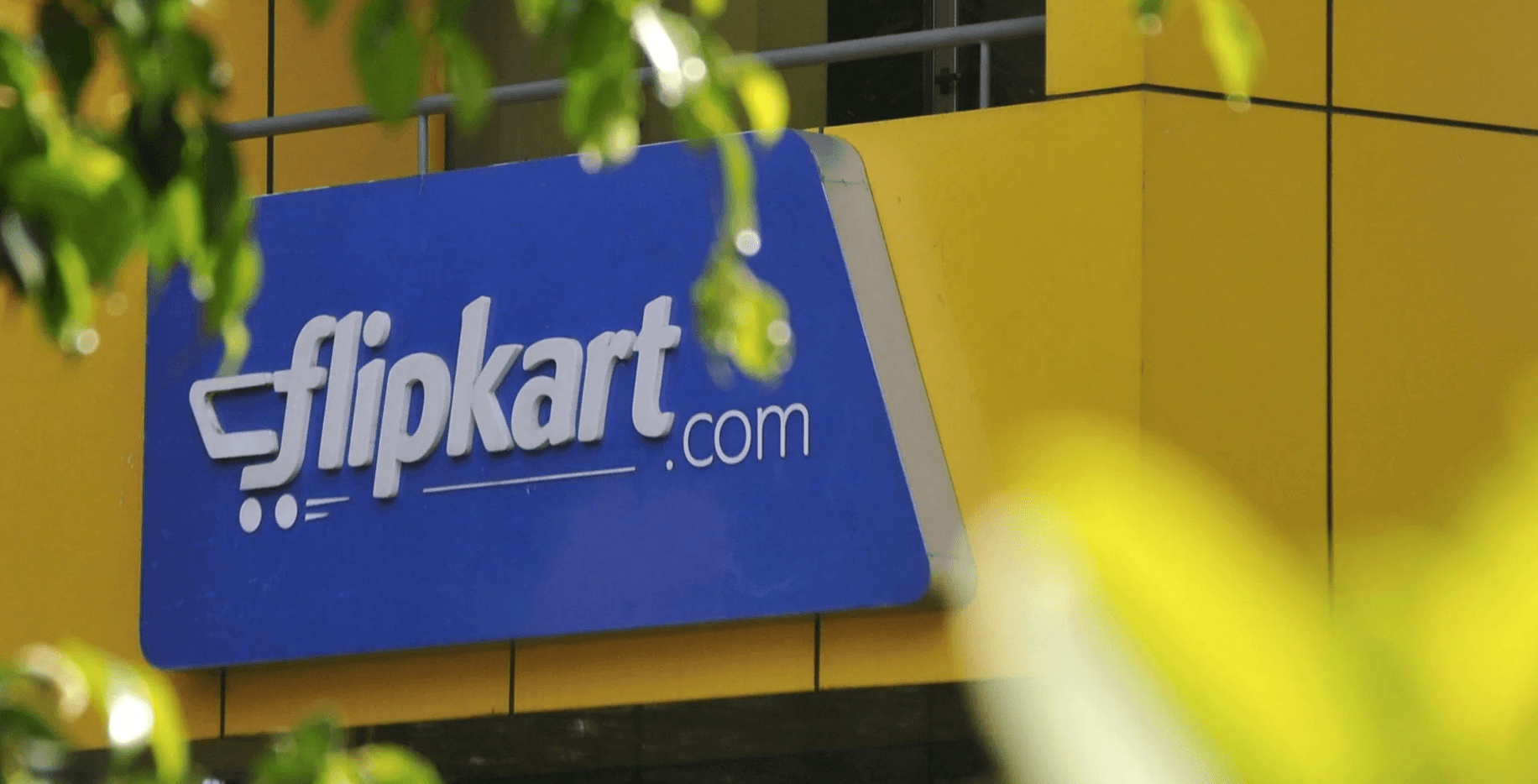
With internet penetration on the rise, the e-commerce sector is booming in India. According to research by Boston Consulting Group, the number of internet users in India will jump upwards of 550 million in 2018 from 190 million as of June 2014. India has over 300 million smartphone users which has surpassed the US to become the second largest smartphone market in the world. As per estimates, the Gross Merchandise Value (GMV) sold by eCommerce companies in India is expected to grow to around $80 billion by 2020 up from around $4 billion in 2009.
eCommerce companies in India are in a cut-throat competition, each trying hard to outdo the other. Many of the top eCommerce companies are deploying artificial intelligence to create better products, user experience, smarter logistics, target the right demographics, and be the preferred choice for their customers. They realise that differentiation is key to their survival in a tough market.
In this article, we will examine how the top 3 eCommerce companies in India by market share– Flipkart, Amazon India, and Myntra, are using AI. As per the estimates of India’s Economic Times, Flipkart and its independently-run subsidiary Myntra, together own a market share of 38.5% while Flipkart alone has a share of about 34%. Amazon India constitutes 29% market share. It may be noted that these are privately-held companies that do not share their revenue or market share numbers and often dispute the numbers the media and analyst firms generate.
Flipkart – $2.4 billion in 2016 revenues (source)
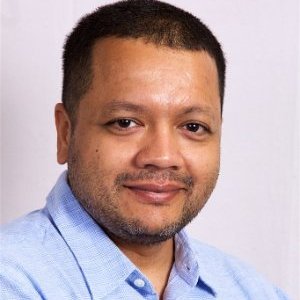
Partnership with Microsoft
Earlier this year, Flipkart partnered with global tech giant Microsoft to provide consumers in India a better online shopping service. As a first step in the broad collaboration between the two companies, Flipkart has adopted Microsoft Azure as its exclusive public cloud platform. The announcement was made when Microsoft CEO Satya Nadella visited Bengaluru in February, his first visit to India after being appointed the CEO of Microsoft.
During an event organised during Nadella’s visit, Flipkart said in a statement that it plans to leverage AI, machine learning and analytics capabilities in Azure, such as Cortana Intelligence Suite and Power BI, to optimize its data for innovative merchandising, advertising, marketing and customer service (this statement is a claim from the author, who states that he was at said press conference).
Project Mira
Flipkart’s AI project known as ‘Mira’ aims to deliver an offline experience to online shopping. The project appears to be a response to Flipkart’s reported 10-11% return rate. Flipkart’s president of product Ram Papatla claims that the company studied its customers and came to the conclusion that a large percentage of their returns could have been prevented with one or two simple questions asked to the customer before their purchase.
This goal of Mira is to scale the in-store experience of having a sales associate – but via artificial intelligence and through digital channels. “…Now, our users with broad intent (searching for, say, shoes or bedsheets) are guided with relevant questions, conversational filters, shopping ideas, offers and trending collections”, said Papatla.
The company claims that this technology is already in use at Flipkart (though we were unable to determine what percentage of users have been exposed to Mira). The Economic Times article (cited two paragraphs above) uses the following example of a real-world application of this conversational technology:
“Say, someone comes to Flipkart searching for an air-conditioner. Because of Project Mira, Flipkart now asks buyers about what kind of AC they want, the tonnage, room size, brand, and such. It is a beginning in helping customers find the exact product they need in online settings that aren’t exactly easy to navigate.” (source: Economic Times)
Like in offline shopping where the sales executive asks a customer about her size and other requirements before making a suggestion, Flipkart is trying to get AI to do something similar.
Silicon Valley Ambitions
Flipkart is also expanding its presence in the Silicon Valley in the US and focusing on AI-based products by making use of world-class research facilities in the Valley. This is being done through F7 Labs, Flipkart’s US-based research arm in Palo Alto.
Mihir Naware, Director Product at F7 Labs, has stated that Flipkart has a number of artificial intelligence problems made more interesting by indian context. “Take color recognition, you can have the standard colors but we also have Gulaabi in India. That is something that has to be taught. The difference between a kurta and a kurti is length but you need to compensate based on whether the picture is shot on a mannequin or a person”, says Naware (in the F7 Labs article cited above). Naware also states that F7 Labs has made progress using natural language processing to understand the specific kind of colloquial English which is commonly used in India.
Flipkart’s Principal Data Scientist Mayur Datar mentions (among other points) that image analysis and deep learning are areas of high interest for Flipkart right now. The video below is the full Tech2 interview with Mayur:
Deep learning skills are indeed scarce, so having a presence in Silicon Valley certainly makes sense from a talent acquisition perspective.
Amazon India
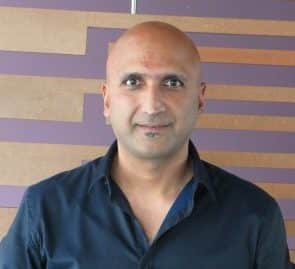
With $2 billion of investments already pumped into its India business operations, Amazon is committed to long-term investment in technology and infrastructure in India, of which AI is a critical technology it has invested in. “AI will enable smart eCommerce, improve operational efficiency and enable new customer experiences (e.g. voice interfaces, conversational systems) that will transform people’s lives,” Rajeev Rastogi, Director, Machine Learning, Amazon India told Emerj.
Amazon India has used machine learning and AI in a number of areas. Rastogi explains some of these areas below:
Correcting Addresses
Addresses in India are not well structured and often users enter incorrect addresses (e.g. wrong pin code or city name) or addresses with missing information (e.g. missing street name). Wrong addresses cause packages to miss delivery dates and lead to failed deliveries. The company has been using machine learning techniques to detect junk addresses, compute address quality scores, correct city-pin code mismatches, and provide suggestions to users to correct wrong addresses.
Catalog Quality
Product catalog defects such as missing attributes like brand, color or poor-quality images/titles can adversely impact customer experience. The company is using AI and machine learning to extract missing attribute information like brand or color from product titles and images.
While we didn’t have the time to confirm specifics on the phone, we can presume that natural language processing is used to assess the written information about a product (in order to fill in information gaps, such as missing brand name). By analyzing thousands of other product listings, and connections between catalog items, a system could hypothetically be trained to fill in some missing information on other products. We can also presume that machine vision is used to add additional information (such as missing color or size of a product listed online).
Product Size Recommendations
In categories such as shoes and other apparel, different brands often have different size conventions. For example, a catalog size 6 may correspond to a physical size of 15 cm for Reebok while for Nike a catalog size 6 may correspond to a physical size of 16 cm. To quote Rastogi:
“We are using machine learning to recommend the product size that would best fit a customer when the customer visits a product page. Our algorithms leverage past customer purchase and returns data (e.g. product size was too large/small) to infer the best size for the customer.”
Amazon India obviously also employs product recommendations in general – just the same way Amazon in the US does. At 22:30 into the video below, Rastogi explains some of the processes and challenges behind Amazon’s approach to product recommendations during a speaking event hosted by Kalaari Capital:
Deals for Events
Machine learning is used to identify relevant products for specific events such as Diwali, Christmas, etc. These are typically products that are in high demand during the event or get high volumes of search queries and review mentions during the event period. Machine Learning algorithms also predict the deals and discounts to offer on the products to achieve a certain sales forecast that helps in better planning.
By training a machine learning system on past holiday purchase data and current purchase activity, a system may be able to calibrate demand more accurately in order to sell products at the right prices to either (a) move certain items at high volume, or (b) maximize profit margins by matching the highest margin products to users during the holiday season.
Product Search
In order to show the most relevant products in search results, the queries are classified into a product category and then show products from that category in search results. “So for instance, the query ‘red Apple iPhone’ belongs to the smartphones category and so we should show smartphones in search results but the query ‘red Apples’ belongs to the grocery category and so we should show grocery items in search results,” says Rastogi.
Myntra (owned by Flipkart)
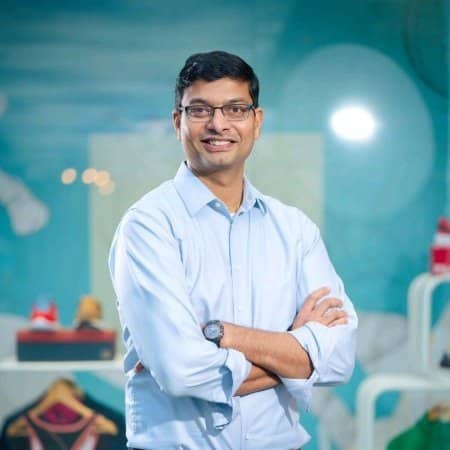
Fashion e-tailer Myntra’s AI initiatives are centred around three key pillars – product, experience, and logistics, says Ambarish Kenghe, Chief Product Officer, Myntra. Each of these areas are explained in detail below:
Product
Myntra is focussing on building intelligent fast fashion through its AI platform known as Rapid. “Fast fashion” is defined as: A contemporary term used by fashion retailers to express that designs move from catwalk quickly to capture current fashion trends (source).
This can dramatically reduce the time taken to create a fashion product to few weeks from the typically long 9-14 months lifecycle. “Based on the available sales data, we figure out what attributes are selling. Then, based on that, our designers start producing the fashion items,” Kenghe told Emerj. “The results have surprised us…we have cracked the code of figuring out what fashion is,” he says.
“You can call it machine augmented design,” says Kenghe. Myntra is using a new technique called Generative Adversarial Networks (GANs) for design which creates products that are similar but not the same. “It’s working very well and this is going to scale in a big way…it’s like the self-driving cars of fashion,” Kenghe claims.
Myntra seems to have also gone a step further in automatically designing its fashion products. This February, they launched T-shirts fully machine generated designs.
Myntra is also using Rapid to intelligently select what to sell on the platform. “You can’t sell everything. We pride ourselves in selling unique fashion.”
Experience
Myntra is using machine learning to improve the payment acceptance rates of online transactions – an issue which is particularly prevalent in India where failure rates are high. According to a study by the Institute for Business in the Global Context (IBGC), online payments transactions typically fail in India due to two distinct set of reasons.
- The first is user abandonment; this could be due to anything from a patchy internet connection, a clunky interface, to just loss of interest.
- The second is that banks, which provide acquiring services in any payment transactions, tend to have poor IT systems.
The study cites as statement from Jitendra Gupta, CEO and co-Founder of Citrus: “bank systems in India can be down for anywhere up to 4-5 hours at a time, which makes the experience extremely frustrating for customers.”
Using machine learning, the system figures out the best payment gateway the payment needs to be routed through. This is done by detecting and analysing thousands of success and failure patterns and then sending it through the most optimized route.
Myntra also enhances the user experience by giving the right recommendations based on what a customer has seen or bought in the past. It uses “collaborative filtering” (a concept we explain in depth in our recommendation engine article), which recommends products to one person based on what another person has just bought and also helps match which fashion goes well with what.
Logistics
As customers often complain about late refunds, Myntra created ‘Sabre’, an AI-based returns system that enables faster refunds for customers who demonstrated good buying-return behaviour. Myntra wants to make its returns policy more efficient as it believes that returns is an integral part of the fashion industry – which relies on sizes, fits, and tastes that make returns more common than other sectors (differentiated goods like apparel tend to have higher return rates than undifferentiated goods).
By analysing a customer’s past returns patterns, Myntra claims that ‘Sabre’ is able to detect which customers are genuinely returning the shipments and which ones are attempting fraudulent activities.
It should be noted that companies at the scale of Myntra (i.e. large online retailers) are in the best position to detect fraud, because they have the most instances of both legitimate and fraudulent activity on record, allowing them to train machine learning systems to predict fraud based on more robust contextual and historical data.
Myntra is also aiming to reduce its rate of return to origin (RTO). A higher RTO translates to higher losses as many cash on delivery (COD) orders are not delivered for various reasons such as customers not being present or not having cash at that point in time. Some customers are even said to offer unbelievable or absurd reasons for placing their returns. “We are now starting to predict if something is going to result in an RTO. If yes, we call that particular customer… AI helps here as calling everyone is not worth our time and effort…Today, we have the least RTO in the industry as far as I know,” says Kenghe.
This kind of customer service “intervention” approach is something that Emerj has previously covered in an interview with AI researcher Dr. Mazin Gilbert AT&T. The company is also concerned with intervening to prevent refunds or customer churn. We suspect this may become common along many industries in the years ahead – particularly in B2C sectors with massive volume.