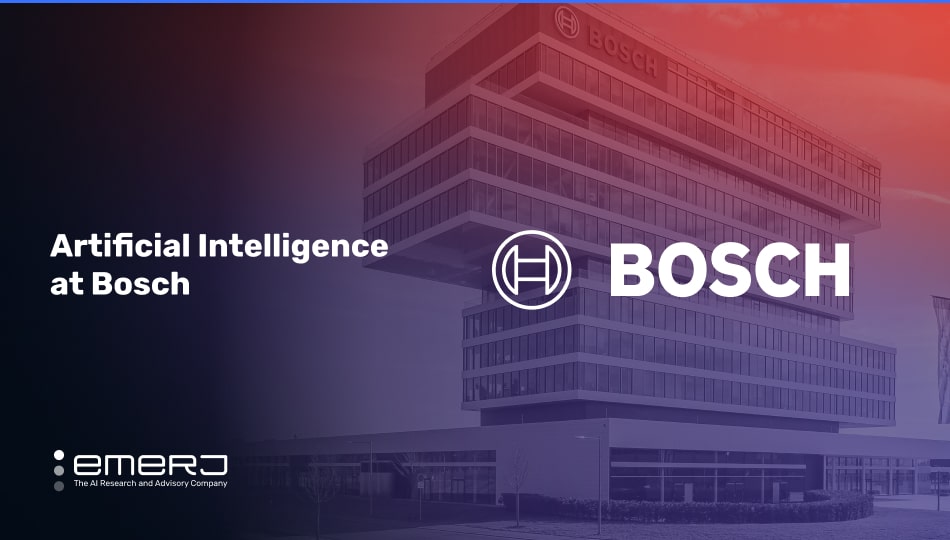
Bosch is a German multinational technology and engineering company headquartered in Gerlingen, Germany. Bosch manufactures products across various industries, including aerospace, automotive, energy and utilities, healthcare and others.
In 2022, the company reported a gross profit of $28 billion. Bosch employs approximately 421,000 people worldwide. Bosch seems very active in research and producing AI-enabled products and services, as evidenced by the more than 1,000 patents the company reports filing since 2018.
The company has its Bosch Center for Artificial Intelligence, designed to enable Bosch to develop and deploy cutting-edge AI technology and services across business sectors. The company researches and integrates deep learning, natural language processing, probabilistic modeling and reinforcement learning.
Bosch’s AI offerings are examined in this article using two timely, relevant use cases:
- Automated Visual Quality Inspection: Reduce inspection time and costs by detecting defects in equipment and increasing inspection accuracy with computer vision and machine learning algorithms.
- Real-time Recommendation Engine: Providing real-time recommendations through mobile apps with recommendation engines to increase customer spend and generate new revenue streams.
First, we explore how Bosch leverages computer vision, machine learning and deep learning to automate quality inspection.
Use Case #1: Automated Quality Inspection
The use of AI and machine learning in predictive maintenance, a close cousin of visual quality inspection, is growing rapidly, expanding into many different use cases.
In a recent podcast episode, Maya HTT Vice President of Industrial AI Remi Duquette discusses the expanding capabilities of computer vision in the context of thermal imaging and where these capabilities best serve the enterprise in reinforcing safety concerns and beyond:
“Thermal imaging cameras are certainly aware. They definitely augment human capabilities because we can’t see any infrared signal, and where those areas could become a safety security issue. But [overheating machinery] definitely is an example of where we would, in those cases, put thermal imaging cameras in locations where we know there’s potential for something to heat up when it’s not necessarily supposed to. And where those areas could become a safety or security issue — or even just change the productivity or efficiency of the line. If you’re going too fast, for instance, in the motor is heating up a bit too much, you may want to dial down a tiny bit to safeguard the longevity of your motors in some cases.”
– Maya HTT Vice President of Industrial AI Remi Duquette
The quality inspection process has progressed significantly in recent years, and strides in automation have played a large part. However, considerable challenges remain, such as lack of efficiency, high costs, limitations of human quality inspection (e.g., fatigue, errors, etc.) and regulatory mandates for the human labor force.
Bosch has developed a solution dubbed Visual Quality Inspection (VQI), an AI-based system that uses computer vision and deep learning algorithms to automate the quality inspection process.
The platform uses generative adversarial networks (GAN) and transfer learning to identify varying defects over time. Aquant CEO Shahar Chen tells Emerj about the enormous impact of generative AI on making predictions in applications with limited training data, such as visual quality inspection:
“You can’t really take data out of the equipment; everything needs to be done on the data that you already have. All the predictions need to be done based on the data that you already have. This is where generative AI creates a gigantic opportunity for that mark. This is where the possibilities are now open to do all different kinds of things, and build all different kinds of models based on the data that you already have.”
– Co-founder and CEO of Aquant Shahar Chen
The Bosch VQI workflow is as follows:
- Image acquisition (inputs): cameras and sensors capture images of the product to be inspected using cameras and sensors.
- Preprocessing: Image calibration and enhancement are employed to improve image quality.
- Feature extraction: features relevant to product quality, such as color, shape, size and texture, are extracted from the preprocessed images.
- Analysis: machine learning algorithms or rule-based systems analyze the extracted features to detect and classify anomalies or defects that may be present.
- Model training: Algorithms are trained using transfer learning, enabling faster and more straightforward adaptation of the VQI solution to different products, processes and industries.
VQI Workflow. (Source: Bosch)
A predictive model and feedback system provides the output data in real-time results, reports and recommendations to operators and managers. Data contained within these reports may include:
- The number and types of defects detected.
- Statistical information (acceptance rates, false positive/negative rates, defect intensity, average inspection time, etc.).
The VQI solution is designed to integrate into the manufacturer’s existing cameras, sensors, IT and operational technology (OT) systems (e.g., ERP, MES, PLC, SCADA). Bosch claims that VQI can be optimized and adapted to different industries, processes, or products using transfer learning and edge cloud computing.
There are potential challenges and limitations for manufacturers to consider, some of which Bosch mentions in its whitepaper:
- Data availability and quality: Bosch’s VQI systems require a large volume of high-quality training data. Collecting, labeling and annotating such a diverse dataset can be resource-intensive, particularly for rare or complex defects.
- Data security: Data privacy and security issues may arise when dealing with sensitive or proprietary data.
- Model scalability and adaptability: Retraining or fine-tuning the models to be scalable and adaptable to different products and processes is costly and time-consuming.
- Environmental and equipment challenges: Trained models must be resilient to environmental changes, such as lighting, noise, or camera angle variations.
In its whitepaper, Bosch cites the following results from a multistage manufacturing process for producing automotive parts:
- 99.8% inspection accuracy, compared to 95% for manual inspection.
- 75% reduction in inspection time, from 20 seconds to 5 seconds per part.
- 50% reduction in inspection costs, from .2 euros (22 cents) to .1 euros (11 cents) per part.
- Projected, unquantified savings from customer complaints, warranty claims, or recalls.
Use Case #2: Real-time Shopping Personalization
SPAR, a multinational supercenter chain founded in the Netherlands, operates in various countries across Europe, Asia, Africa and Oceania.
SPAR locations in India sought to implement a solution that enhances customer experience and engagement.
SPAR India decided to integrate a solution that could provide personalized offers, deals and promotions to its customers in real-time via a mobile app.
To accomplish this, SPAR teamed up with Bosch to develop a recommendation engine that leverages indoor positioning systems, machine learning and big data analytics to provide real-time, personalized recommendations to its customers.
The platform, codenamed IERO, was to be integrated into SPAR’s customer relationship management (CRM), point-of-sale (POS) and enterprise resource planning (ERP) systems and processes.
Bosch’s value proposition for IERO. (Source: Bosch)
According to the case study published by Bosch, the IERO workflow is as follows:
- Data collection and analysis: The platform collects various data, including consumer profiles, purchase histories and loyalty membership data, to understand the preferences and behavior of each customer.
- Recommendation generation: The platform generates personalized offers for each consumer using the input data collected in the first step. The platform uses big data analytics and machine learning algorithms to create recommendations.
- Recommendation delivery: The platform delivers the offers to SPAR consumers through the mobile app, either when they open or enter a SPAR store.
- Feedback and adjustment: The platform receives feedback from consumers via ratings and reviews and uses it to improve the accuracy and effectiveness of the recommendations.
Bosch reports that SPAR’s business has benefited in the following ways:
- Improved ROI via targeted marketing and advertising
- Increased customer engagement and retention
- Better positioning of products
- More thorough understanding of customer behavior via analytics
- Additional revenue streams via advertisement conversions
The Bosch use case does not share any quantitative results.