AbbVie is a global biopharmaceutical leader with approximately 55,000 employees in over 70 countries. In 2024, the company invested over $10.8 billion in research and development, supporting active immunology, oncology, and neuroscience clinical programs.
To accelerate drug discovery, AbbVie is applying artificial intelligence (AI) to improve early-stage decision-making. The company aims to streamline target discovery and design more effective therapeutics using AI tools that enable faster insights from complex biomedical data.
The following sections explore how AbbVie is applying artificial intelligence across two core areas:
- Improving drug targeting through data integration: AbbVie applies machine learning to integrate multi-source data, enabling the identification of promising new drug targets to accelerate the drug discovery process.
- Optimizing drug design with generative AI (GenAI): AbbVie leverages GenAI and deep learning models to predict and optimize drug candidates, improving the efficiency of the drug design process.
Improving Drug Target Discovery Through Data Integration
Drug discovery is a complex and time-consuming process, on average taking 10 to 15 years, from initial research to market approval. As exemplified in a 2021 review article from Drug Discovery Today, a major bottleneck in the drug discovery process is target identification, which requires researchers to navigate massive, fragmented datasets across genomics, proteomics, and clinical domains. Without cohesive systems, critical insights are easily overlooked.
AbbVie developed the R&D Convergence Hub (ARCH), a machine learning-driven platform that streamlines early-stage drug discovery by applying AI to a centralized, multi-domain dataset to address these inefficiencies.
According to AbbVie use case documentation, ARCH:
- Centralizes data access: Connects over 200 internal and external structured and unstructured data sources, helping eliminate fragmented search efforts.
- Enables large-scale collaboration: It provides researchers with access to over 2 billion points of scientific knowledge across genomics, proteomics, and clinical research domains.
- Surfaces hidden relationships: Uses machine learning to help researchers identify relevant patterns and connections across datasets faster than traditional manual review.
By consolidating diverse datasets and applying AI models, ARCH empowers AbbVie researchers to accelerate early-stage drug discovery efforts and improve the efficiency of identifying novel drug targets.
Starting at roughly two minutes in the video below and running until the 3:21 mark, Howard Jacobs, VP of Quantitative Medicine and Genomics at Abbvie, explains the multiple ways Abbvie used machine learning to fine tune proteins for monoclonal antibodies:
According to AbbVie, AI consolidation of multi-domain data allows researchers to more quickly progress from hypothesis to target validation — freeing them to spend more time on experimental design and accelerating the early stages of drug discovery.
Although AbbVie does not publicly disclose specific metrics on time savings from its ARCH platform, available data suggests a measurable acceleration in R&D output since its introduction.
According to Evaluate Vantage, between 2016 and 2021, AbbVie secured five new drug approvals. By contrast, a 2023 AbbVie press release highlights 19 major product or indication approvals since 2021, with an additional 12 expected by 2025 — a shift that aligns with the platform’s growing role in early-stage discovery.
This trend echoes broader industry research. McKinsey estimates that AI can reduce drug development timelines by up to 500 days and lower R&D costs by approximately 25%.
Additionally, research from Harvard’s Therapeutics Data Commons supports the strategic integration of diverse biomedical datasets to improve target identification and streamline discovery—ARCH’s core function. While many variables influence drug approval volume, the ongoing expansion of ARCH and AbbVie’s recent pipeline momentum points to the platform’s strategic value in enhancing research workflows.
GenAI for Drug Design Optimization
Traditional drug design methods often rely on high-throughput screening (HTS) of existing chemical libraries — a time-consuming and costly process that can limit the exploration of novel and more effective molecular structures.
Researchers historically needed to manually sift through large chemical datasets, increasing the risk of overlooking promising compounds. Before the advent of HTS, screening approximately 3,000 compounds could take 1–2 years. Even with modern HTS, screening up to a million compounds might still require 1–3 months, depending on the complexity of assays and available automation infrastructure.
To address these limitations, AbbVie is leveraging GenAI to enhance molecular design’s efficiency and creativity.
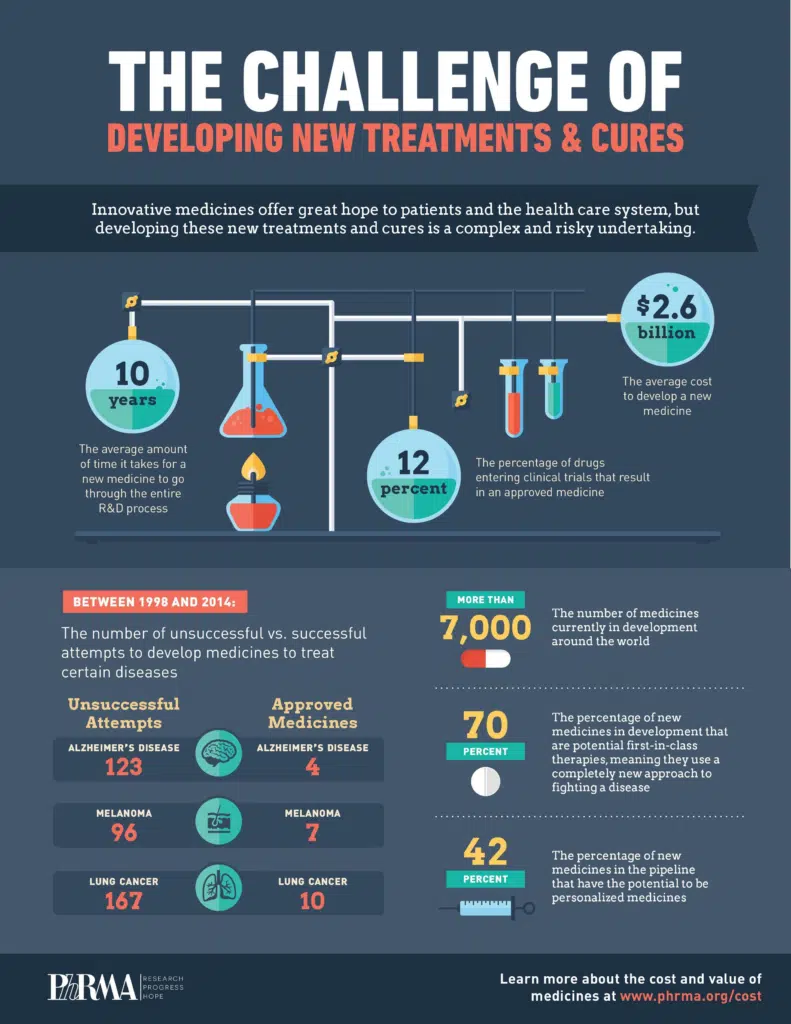
Infographic illustrating challenges in drug development. (Source: PhRMA)
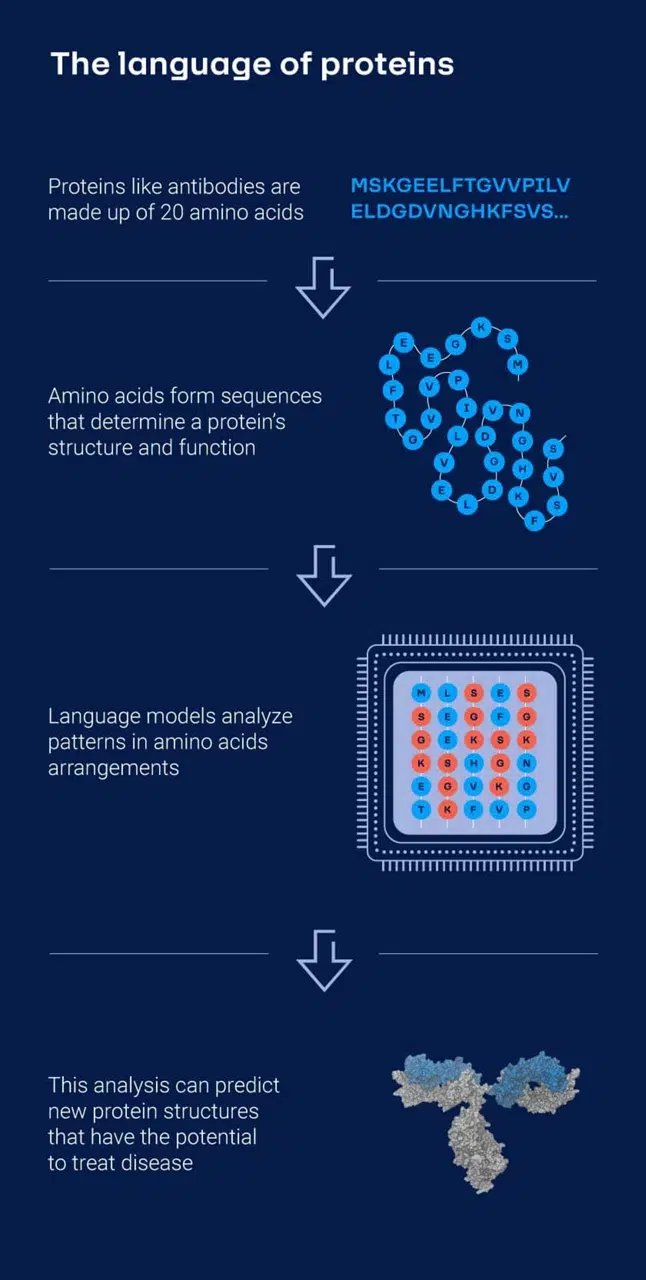
Image illustrating how GenAI processes protein language. (Source: AbbVie)
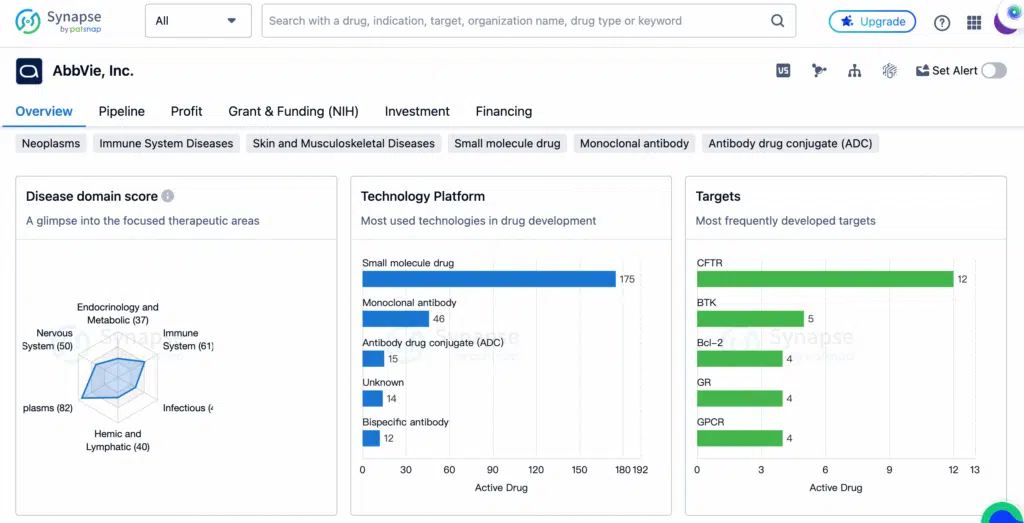
AbbVie’s current pipeline by target, disease area, and modality—key domains that inform the training of generative AI models for novel drug design. (Source: AbbVie)
AbbVie’s approach involves training generative models on extensive datasets of known chemical structures, properties, and biological interactions. These models learn fundamental principles of molecular behavior and can generate entirely new molecules tailored to desired traits, such as optimal binding affinities or favorable drug-like properties. Predictive algorithms further assess the viability of these AI-generated compounds before physical synthesis.
In the one-minute video below, Christopher Negron, Ph.D., Principal Research Scientist at AbbVie, shares how protein language models and AI can accelerate the discovery of new medicines:
In addition to small molecule design, AbbVie applies large language models (LLMs) to antibody engineering. These models are trained on amino acid sequences to learn the underlying protein structures.
Understanding these underlying protein structures enables researchers to generate new antibody sequences that exhibit desired traits, such as greater stability or improved binding to specific biological targets.
Key improvements enabled by GenAI at AbbVie include:
- Expanded molecular exploration: AI models design molecules beyond traditional chemical libraries, opening new therapeutic possibilities.
- Accelerated lead discovery: Predictive modeling significantly reduces the time required to identify and prioritize high-potential drug candidates.
- Prioritized candidate selection: Researchers receive ranked lists of novel molecules based on AI-predicted binding efficacy and drug-likeness, focusing their resources on the most promising candidates.
- Reduced manual workload: Automating early-stage design tasks allows AbbVie’s chemists to concentrate more on experimental validation and optimization.
Research from MIT’s Computer Science and Artificial Intelligence Laboratory (CSAIL) and Harvard’s Wyss Institute supports using AI to accelerate early drug discovery by improving compound screening and prioritization.
While AbbVie has not disclosed specific ROI from its GenAI initiatives, industry research from McKinsey estimates that AI-enabled drug discovery can reduce development costs by up to 30% and accelerate timelines by as much as 40%. These findings reinforce AbbVie’s investment in generative tools as a strategically grounded method to streamline innovation in drug development.
In line with this broader trend, AbbVie reports that its clinical trial site selection process is now 50% faster, thanks to predictive analytics and machine learning models that guide decision-making. This acceleration helps the company prioritize the most viable candidates and reduce delays in transitioning from molecule to trial.