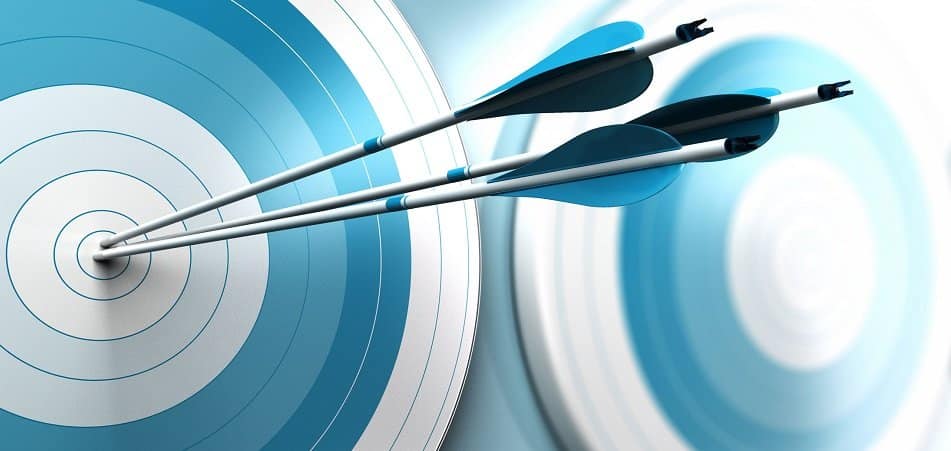
This article was written and contributed by Tom Gilbert, PhD Student in Machine Ethics and Epistemology, UC Berkeley, and was edited and published by the Emerj team.
Machine learning (ML) is now widely deployed to shape life outcomes in high-risk social settings such as finance and medicine. However, this automation of human-led tasks may generate opaque classifications or inefficient interventions.
Consider the case of predicting pneumonia risk. A group of researchers was tasked with assigning hospital patients with a pneumonia risk score, signifying their probability of death. An ML system unexpectedly revealed that asthmatics were less likely to die from pneumonia than non-asthmatics. This clearly did not match clinical expertise, but appeared to be a legitimate pattern in the data.
The paradox was explained after examining the context of use: asthmatics were more likely to seek medical attention for pneumonia symptoms than non-asthmatics and were more likely to be admitted to an intensive care unit.
Hence, the asthma variable implicitly encoded other clinical characteristics, such as time to care, implying a relationship between asthmatics and lower risk scores. Had this relationship fed back into the decision pipeline, it might have exposed patients to major healthcare risks and compromised the hospital’s credibility.
Through proactive attention to interactions between the environment and ML models, stakeholders could both protect their company’s values and help ensure organizational trust.
We propose the problem of measurement itself as a core domain for ML, interpreting interventions in terms of how well institutions can predict and reshape the context-specific social dynamics that motivate their own decision-making.
ML’s discoveries will clarify when assumptions about the value of any specific metric are in need of revision. As such a proactive example, consider a predictive analytics approach used to redesign the Medicare Shared Savings Program contract.
Comparing different hospital configurations by type of care and marginal spending, the model boosted Medicare savings up to 40% without sacrificing provider participation, improving financial outcomes while also aligning organizational incentives.
With such cases in mind, we propose a social measurement assurance program (sMAP) that deploys ML to investigate how institutional policies align with measured outcomes. sMAP rests on a representational mapping between observed human data, difficult-to-measure human qualities of causal significance, and ML-informed interventions.
When institutions conduct a mapping from observed data to decisions, there is an implicit mapping onto some construct space that defines the context for the decision.
For example, in college admissions, GPA scores hint at general intelligence, a difficult-to-define construct that the admissions officers are actually using as a basis for decisions. Assessing algorithms and using ML to solve real problems is about recognizing how these three distinct spaces of observation, constructs, and decisions shape each other.
An sMAP helps prevent the following confusions between distinct mappings:
(1) The methods of measurement are inconsistent or misaligned with a business’s causal models of the world. For example, hospice workers may use significant discretion when providing data to healthcare actuaries about specific terminal patients, making their testimonies unreliable or unrepresentative.
(2) Gathered measures fail to map onto the institution’s construct space. We have already seen this in the case of predicting pneumonia risk.
(3) The decision space does not account for changes made to the observation space. Medical triage may still de-prioritize asthmatics for specific types of urgent care even after actuaries learn to control for admission to intensive care units when measuring intakes.
(4) There is no feedback mechanism to constructs, such that they are not adjusted to better align with intervention aims. To continue the example in (1), hospice workers may rarely communicate patients’ preferences to actuaries (e.g. the construct of palliative care is ignored), making interventions grossly neglectful.
The image above demonstrates these steps.
While an sMAP could incorporate diverse tools and approaches, below we highlight how ML itself could be positioned as a tool to support it. By reframing the above examples in positive terms, we show how ML’s significance is less defined by the algorithms it employs than by the role it plays in making decisions:
- Measure Validity. Hospice workers agree to report the same kind of data to actuaries, making ML predictions able to precisely validate, verify, and correctly implement specific components of the measurement procedure.
- Measure to Construct Correspondence. As in the pneumonia risk case, even simple regression methods surface relations between measures and decisions that encourage critical reviews of how a given measure reflects the construct of time to care.
- Decision-to-Measurement Feedback Adjustment. Actuaries can compare a predictive ML model based on the old measurement of terminal cases and a new predictive model controlling for intensive care unit intakes. This would suggest the relative impact of decisions if the observation space (i.e. pneumonia patients) is updated to include intake triage.
- Construct Verification. Hospice workers are required to exhaustively report patients’ requests for support that actuaries would label as palliative care. If only specific types of patients request this, the construct may be either (a) not as important as the institution suspects, or (b) within the hospital’s economic purview for selected patients, thereby preserving efficient care as well as palliative care.
ML could both surface and contour covariates for decision-making. Not only are important measures revealed, unimportant constructs may be dismissed as context-inappropriate.
Depending on the method used, connections may also be drawn between measures, hinting at deeper relations between constructs and potentially altering existing legal or scientific understandings of relevant social variables.
Entirely new measures could be codified if ML helps identify covariates for which we have no prior construct-based intuition. ML thus constitutes a technical intervention in how policy interventions are made, shifting the grounds by which decisions are justified by altering the conditions under which they can be envisioned, enacted, and evaluated.
In these ways, use of ML could hint at where a given institution might dedicate future resources to improve understanding of the context of its own decisions.
Header image credit: Adobe Stock