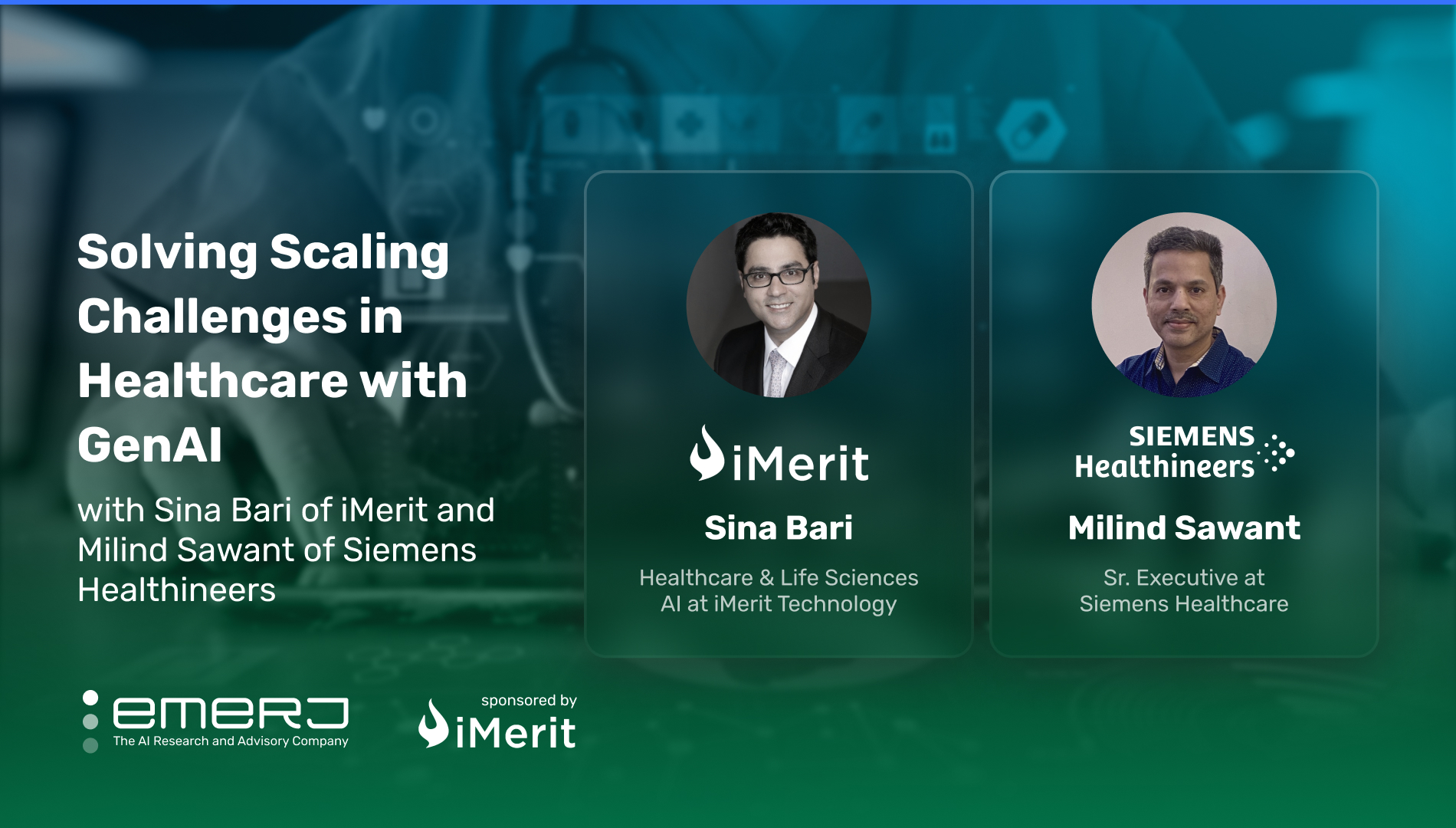
This interview analysis is sponsored by iMerit Technology was written, edited, and published in alignment with our Emerj sponsored content guidelines. Learn more about our thought leadership and content creation services on our Emerj Media Services page.
The healthcare industry faces unique scaling challenges and the integration of Generative AI (GenAI) offers a promising solution. GenAI is a transformative tool that leverages large language models (LLMs) and deep learning algorithms to address various complex healthcare problems — these range from optimizing patient care to streamlining administrative processes. By harnessing GenAI’s capabilities, healthcare providers can improve diagnostic accuracy, streamline record-keeping and enhance patient engagement.
Emerj CEO and Head of Research Daniel Faggella recently spoke with Milind Sawant, Founder & Lead for the AI/ML & DFSS Center of Excellence at Siemens Healthineers, and iMerit Technology AVP Sina Bari to discuss how to save costs while scaling the transformative power of generative AI in healthcare.
The following analysis examines three critical insights from their conversation:
- Streamlining healthcare policy navigation: Leveraging LLMs to quickly and accurately answer specific questions on healthcare insurance policies.
- Interfacing with internal proprietary data: Leveraging the language processing abilities of LLMs to create an intermediary interface for accessing a broad range of internal proprietary data.
- A two-point strategy to address challenges in cost-effective scaling: Optimizing international expertise by collaborating with domain experts worldwide and optimizing expert time, using automation for cost-effectiveness.
Guest: Milind Sawant, Founder & Lead, AI/ML & DFSS Center of Excellence, Siemens Healthineers
Expertise: DFSS and AI/ML integration with medical systems
Brief Recognition: Milind is a Senior R&D Executive with over two decades of experience at Siemens Healthcare who leads multifaceted teams and budgets. His expertise lies in engineering and AI/ML integration for global platform products, fostering innovation and cost savings. He founded two Centers of Excellence, uniting 450 employees to tackle complex problems. Milind excels in team leadership, DFSS and Agile methodologies while demonstrating strong communication skills.
Guest: Sina Bari, AVP, iMerit Technology
Expertise: AI, Machine Learning, Data Operations, Healthcare Information Technology
Brief Recognition: Sina is a Stanford-trained reconstructive surgeon with an accomplished history in the medical device and information technology spaces. His passions lie at the intersection of business and product development, healthcare and AI. As AVP of Healthcare & Life Sciences AI, he manages the strategy and growth of iMerit’s medical division from ideation to its current market leadership position.
Streamlining Healthcare Policy Navigation
Milind begins by addressing the potential and excitement surrounding LLMs. He acknowledges that these models have significant potential but are accompanied by a great deal of hype and high expectations. Providing a practical example of generative AI, he discusses a common scenario in organizations where employees must navigate healthcare-related HR policies. Typically, this involves sifting through numerous documents or contacting HR partners. LLMs can simplify this process by enabling employees to ask specific questions about their healthcare coverage, such as whether scuba diving is covered and receive quick, accurate responses.
Milind highlights an organizational challenge related to AI – a need for a comprehensive understanding of what AI can and cannot do across the enterprise. He shares an example from the pharmaceutical industry, where the pressure from top management to use generative AI may not align with the actual needs of the project, showcasing a gap in understanding between decision-makers and the technology.
“The problem I’m seeing is, because there is a lack of understanding, people think that generally, AI is a solution to all problems of mankind. One of my friends is in the pharmaceutical industry. He had a customer saying, ‘Can we user please use generative AI because my boss is asking me to use it in our workflow?’ The use cases that he was describing did not need generative AI; they could just use a traditional machine learning algorithm. But because they are under pressure from top management, who may or may not understand the power, strengths and weaknesses of this technology. They are forcing generative AI because they want to tell their top boss they’re using generative AI. Now you’re struggling, ‘How do we find a use case?'”
-Milind Sawant, Founder & Lead, AI/ML & DFSS Center of Excellence, Siemens Healthineers
Sina concurs with Milind regarding the rapid growth and subsequent cooling off of the hype surrounding generative AI. He then delves into a specific application in the radiology space, where large language models (LLMs) could be leveraged to translate model results into reports and highlight the importance of reinforcement learning in shaping these reports. He highlights the limitation of traditional computer vision models that rely solely on radiologic data, emphasizing the need for a broader, multimodal approach that considers clinical context, lab values and clinical history.
Furthermore, Sina underscores the need for flexibility and modularity in AI solutions, given the rapidly evolving nature of the field. He advises against static solutions that could quickly become outdated in this fast-paced landscape. Sina’s insights here emphasize a problem-driven approach to technology adoption, particularly regarding large language models in healthcare. He suggests mapping AI development by focusing the solution on a well-defined problem and applying technology as a solution.
Interfacing with Internal Proprietary Data
Milind and Sina agree on the cost and data security challenges of training large language models. Building upon the same, Milind adds the issue of proprietary data, where companies are hesitant to use their data for fear that it may be used to train the LLM and potentially be accessible to competitors.
Milind then introduces a “prompt engineering” strategy to address these concerns. Instead of directly training the LLM using the company’s sensitive data, he suggests creating an intermediary interface. This interface would allow for uploading HR documents, such as PDFs and Excel files, which are then processed through a cognitive search system.
Such a system can then extract relevant information from the documents and HR policies. The LLM is utilized primarily for its language processing abilities, such as understanding questions, searching through records and generating grammatically correct responses.
To this, Sina raises the issue of whether LLMs should be hosted on-premises or in single-tenancy environments. He underscores the significance of integrating a patient’s complete health data to achieve precision health.
Sina delves into the complexity of patient data, noting that it is about more than just ID identification, yet there are significant challenges to anonymizing comprehensive health histories fully. He explains that patient health issues are intrinsically tied to their longitudinal history, making it essential to maintain stringent data security measures.
“When it comes to the entire patient’s health data, it’s not simply a matter of identification. There is no way to truly anonymize, if I tell you someone’s whole life story in terms of their health history, that’s going to be a very unique and potentially identifiable piece of information, even if I take your name and medical record number out. But that is what gets us towards precision health because every health problem happens within the context of a longitudinal patient history. So to work with all of that data requires that we maintain the sort of the most stringent data security policies.”
– Sina Bari, AVP, iMerit Technology
Gearing the conversation towards the landscape of regulatory challenges associated with AI and machine learning, Milind addresses the fact that regulatory bodies like the FDA have been evolving their guidance to accommodate the rapid advancement of technology.
He notes that these regulations aim to ensure safety, especially in the healthcare industry, where lives are at stake. Milind emphasizes that as a manufacturer of medical products distributed worldwide, they must comply with various regional regulations and standards, not limited to the FDA.
He further highlights that FDA guidance documents have evolved over the years, focusing on understanding how software and machine learning models will be upgraded and the potential risks these changes may pose. The overarching goal is to ensure no harm to patients or users due to technology. However, Milind notes that – while making significant strides – regulatory bodies still have a long way to go before they are caught up to the rapidly evolving technology landscape.
Sina discusses their experience supporting products undergoing FDA regulatory approval using pathways like the 510(k). He notes that while the FDA has focused on metrics like test data representation, they still need to address training data specifics. He anticipates that the FDA may eventually require a certain level of expertise and digital signature in training data.
He touches on the importance of creating a “gold set” through consensus and arbitration for validating AI models. Sina explains that the definition of good performance can vary depending on the context and expertise levels. He acknowledges the leadership of companies like Siemens in developing a framework for testing AI applications in healthcare and how it extends to other domains as they mature.
A Two-Point Strategy to Address Challenges in Cost-Effective Scaling
Milind highlights the necessity of a multimodal approach when diagnosing patients, drawing an analogy to how physicians consider multiple parameters and data points to arrive at a diagnosis. He stresses the importance of incorporating this multifaceted perspective into the same AI tools healthcare leaders are developing to facilitate the diagnostic process. He emphasizes that a single parameter or data source cannot adequately address the complexity of diagnosing patients.
Milind then transitions to discussing the challenges inherent in clinical decision support systems. He addresses several critical aspects, including bias mitigation and ensuring that patient data is used exclusively and with explicit patient consent.
He also touches on the potential involvement of insurance companies, particularly in the context of potential lawsuits or legal matters related to patient data. It adds a layer of complexity to the process, as the priorities and interests of these various stakeholders may not always align perfectly.
Milind delves into the issue of patient privacy and the fear that their data might be misused or inadvertently leaked. He acknowledges the genuine desire of patients to help others with similar health conditions despite their concerns about data privacy. Patients prefer that their names and specific health information remain confidential on public platforms like social media. Still, they are often willing to share anonymized data to advance medical knowledge and treatment options.
Echoing Milind’s concerns about data silos and privacy, Sina offers a two-point strategy to address the challenges, particularly in cost-effective scaling for expertise. The techniques he mentions are:
- Leveraging international expertise: He suggests utilizing domain experts in different regions like Latin America, India and Africa to pool knowledge from diverse sources and enhance collective expertise. He highlights the importance of efficiency in communication tools for collaboration among these global experts. Despite disparities in technology availability in various communities, he suggests that physicians often share standard textbooks and knowledge sources. Leveraging this common foundation can bridge technological gaps.
- Efficient use of resources and automation: He draws an analogy to the principle in surgery, where efficiency is achieved by performing fast tasks quickly and slow tasks slowly. In the data structuring context, this means focusing expert resources judiciously based on task complexity and using automation where feasible.